
Collective Brain Alignment During Story Reception: Shared Neural Responses in French, Chinese, and English Listeners of Le Petit Prince, 小王子, or The Little Prince
Copyright ⓒ 2024 by the Korean Society for Journalism and Communication Studies
Abstract
Across linguistic and cultural divides, humans engage with stories as a central form of communication. Previous studies reveal that when humans listen to the same story told in their native language, their brain activity becomes temporally aligned. This story-evoked alignment of inter-subjective brain processes holds great theoretical significance as it points to neural commonalities that underlie communication. Yet, does this collective alignment of brains transcend linguistic boundaries? Analyzing brain responses of French, English, and Chinese speakers exposed to their respective renditions of ‘Le Petit Prince,’ this study reveals alignment across the brain’s default mode network. This network, which is implicated in sense-making and social cognition, exhibits similar spatio-temporal responses regardless of language, suggesting a universal processing framework. Our findings highlight the covert connection stories forge between diverse brains. This underscores the fundamental neurocognitive architecture underlying story processing and the collective impact of stories on human cognition and communication.
Keywords:
communication neuroscience, collective alignment, mutual understanding, story, listeninglisteningThe same story can be told in different languages to audiences across the world. For instance, the famous story ‘Le Petit Prince’ by Antoine St. Exupery has been translated into 382 languages and sold over 140 million times. This suggests that the story’s popularity is neither dependent on the linguistic surface form of the original French story, nor the specifics of particular communities. Instead, the story’s ability to resonate with people across the world appears to be a result of its content. This paper explores how listeners’ brain activities align1 when they are exposed to the same story, offering a theoretical rationale for this phenomenon and its significance for communication.
The paper is organized as follows: First, we introduce research on how brain responses to stories emerge along a continuum from basic sensation to higher-level cognition. Next, we present how inter-subject correlation analysis of neural data can reveal how listeners’ brains become temporally aligned during story reception, and how the extended neurocognitive network model provides a theoretical framework for understanding these effects. Then, we introduce the specific study in which 110 listeners (27 French, 33 Chinese, and 50 English) were exposed to the ‘Le Petit Prince’ story told in their respective native language: French (‘Le Petit Prince’), Chinese (‘小王子’), or English (‘The Little Prince’). After presenting the results, we discuss the implications of this story-evoked brain alignment for communication theory.
How Stories Prompt Brain Activity Along the Sensation-to-Cognition Continuum
When individuals listen to a narrated story, this sets forth a complex, but orderly cascade of internal neurocognitive responses that can be measured via neuroimaging (Margulies et al., 2016; Mesulam, 1998). Initially, the sounds entering the ear are transformed into neural impulses, which then travel along the auditory nerve into the brain. The early auditory cortex analyzes basic sensory2 and perceptual aspects of this incoming stimulus, such as its frequency content (Saenz & Langers, 2014). At a subsequent stage of information processing, neurocognitive processes related to word-level analysis (e.g., parsing, lexical retrieval, etc.) are engaged, which in turn provides the basis for yet more abstract analyses of words in the context of other words (e.g., sentence-level and syntactic neurolinguistic processes). The neural processes underlying these computations are increasingly understood (Caucheteux et al., 2021; Toneva et al., 2020; Wehbe et al., 2014), and their functional neuroanatomy has been mapped out in hundreds of auditory neuroscience and neurolinguistic studies (de Heer et al., 2017; Huth et al., 2016; Specht, 2014). Last, the breakthrough success of large language models has further emboldened these notions of parallel and distributed neural networks as a biological and computational mechanism of language3 (Goldstein et al., 2022; Hale et al., 2021).
However, it is obvious that beyond sensoryperceptual and linguistic processes, stories evoke a host of additional processes, such as those related to social cognition and story understanding (Grall et al., 2021; Green et al., 2003). As neuroimaging advanced over the past two decades, more and more studies also have begun to address the brain basis of these processes, which are key for their impact and success (Ferstl et al., 2008; Mar, 2011; Willems et al., 2020). Although this literature is too voluminous to review extensively here, we know that processes related to story cognition recruit post-perceptual and extralinguistic brain systems, such as the so-called salience, the executive control, and particularly the default mode networks (Ferstl et al., 2008; Mar, 2011; Menon, 2015; Schmälzle & Grall, 2020; Schmälzle et al., 2013; Yeshurun et al., 2021). Thus, to understand how the brain responds to stories beyond auditory and linguistic mechanisms alone, additional regions across the whole brain need examination. In particular need of examination is the default-mode network (DMN), a system that integrates incoming sensory-perceptual information (e.g., word order or spacing, vocal tone, etc.) with the broader context and situational model (e.g., keeping track of characters and events over the entire story) (Grall et al., 2021; Hasson et al., 2015; Lerner et al., 2011; Mesulam, 1998; Yeshurun et al., 2021). Given the DMN’s role in integrating this information, the DMN has often been described as having sense-making functions.
In conclusion, this continuum from sensation to cognition offers a guiding theoretical framework for understanding how the brain reacts to narrated stories: As people process a story, such as ‘Le Petit Prince,’ auditory regions should be sensitive to over-time variations of sound-level characteristics, perisylvian regions should be modulated by variations in word- and sentence-level linguistic properties, and brain systems beyond audition and language processing should respond to features that go beyond the information extracted during previous analyses, i.e., making sense of the influx of information by integrating it into a meaningful internal model of the unfolding story (Kintsch, 1998; Lerner et al., 2011; Yeshurun et al., 2021).
Why Listening to the Same Story Induces Temporally Aligned Brain Responses Across Audience Members
The previous section provides a general model for how a story is analyzed in the brain of one single individual along a continuum that ranges from sensation (hearing sounds) to perception (analyzing language) to cognition (understanding the story and following it over time). In this section, we provide a rationale for why listening to the same story should evoke similar brain activity along this continuum across the brains of audience members.
Considering the reception of a story from a communication perspective, it is apparent that a story provides the same content to all recipients (i.e., one-to-many type of communication). For example, the ‘Le Petit Prince’ story that will be the focus of this paper comprises a temporal sequence of words that is identical for all readers or listeners. Thus, if this identical temporal word sequence (i.e., the same story) is received and processed by different individuals’ brains, we would expect this to set forth similar neurocognitive processes at different levels of the sensation-to-cognition continuum. To the extent that people’s brains are similar in terms of structure and functions, which we know is the case, we can expect that the same story (or word sequence) would also induce similar processes in auditory brain regions, language-related brain regions, and regions involved in narrative processing (Grady et al., 2022; Grall et al., 2021; Nguyen et al., 2019). This reasoning of brain’s functioning similarly has been termed extended neurocognitive network model (Schmälzle, 2022) because the logic expands Mesulam’s classical sensation-to-cognition framework to encompass situations where multiple brains are exposed to the same stimulus, or nearly identical stimuli, such as a story, a movie, or other types of mass communication. A more elaborate discussion of the theoretical framework and its connection to communication science is provided in communication science (Schmälzle, 2022; Schmälzle & Grall, 2020a) and neuroscience articles (Hasson et al., 2012; Zadbood et al., 2017).
Measuring Story-Induced Temporal Alignment of Brain Activity Via the Inter-Subject Correlation Approach and Previous Findings
The inter-subject correlation (ISC) approach provides a principled framework to examine this story-induced temporal alignment of brain activity (Hasson et al., 2012; Nummenmaa et al., 2018; Schmälzle & Grall, 2020a). At its heart, this approach involves cross-correlating brain activity time series from recipients who were exposed to the same stimulus. This approach works on a region-by-region basis, or correlating brain responses in corresponding brain regions, like auditory cortex activity in listener 1 to the auditory cortex activity in listener 2, frontal cortex activity in listener 1 to frontal cortex activity in listener 2, and so forth. The resulting ‘inter-subject correlation’ maps reveal the degree to which the brain responses evoked by a common stimulus, such as a story, resemble each other across members of an audience over time and where in the brain this inter-subjective alignment occurs.
Previous work using the ISC framework for audience response measurement has yielded several key insights (Hasson et al., 2010; Lerner et al., 2011). First, we know that shared responses in the auditory cortex are relatively obligatory and primarily driven by the sensory properties of individual sounds. For instance, when people are presented with the same auditory stimulus, their auditory cortices show very similar responses, but when the sound is altered for one individual, that particular individual’s auditory cortex activity will decouple from the rest.
Second, as we proceed further along the sensation-to-cognition continuum, the degree of shared brain responses becomes less a function of the specific input stimulus, and more a function of the match between the stimulus input and existing knowledge structures or regional processing sensitivities. To illustrate, if people are presented with a story in a language of which they are in command4, then their brain activity in languagerelated regions will correlate relatively strongly across brains (Honey et al., 2012). However, if a person is not in command of the language, then this person’s brain activity will be less similar to that of others, and she/he will not be able to understand the story.
Third, it has also been shown that shared responses in post-perceptual brain regions are sensitive to manipulations of higher-level content or audience properties (Chen et al., 2017; Schmälzle et al., 2013). For instance, if people are given different prior information and then exposed to the same story, people who received the same prior information exhibit more similar brain responses (Yeshurun et al., 2017).
To summarize the logic so far, we assert that the story-evoked brain responses along the sensationto- cognition continuum constitute the nuts and bolts of story reception processes, and computing ISC analysis allows us to reveal how these brain systems become engaged and temporally aligned while audience members are processing the same story (Schmälzle & Grall, 2020a). Starting with sensory processes in the auditory cortex, it is clear that listeners’ auditory cortices respond with similar, i.e., time-aligned responses, as they respond to the incoming acoustic stimulus. However, as the sensory input is transformed into cognition, a purely stimulus-driven explanation of the resulting time-aligned brain responses falls short of explaining why ISC emerges in areas beyond the auditory cortex. In the following section, we will present the rationale for why we expect that even different versions (translations) of the same story should induce temporally aligned responses across listeners’ brains.
The Case for Aligned Responses to Different Story Versions in Sense-Making Regions
Previous work examining temporally coupled brain responses to stories and related narrative stimuli suggests that similar responses in postperceptual brain regions, such as the precuneus or prefrontal cortex, are associated with more complex cognitive processes (e.g., short-term memory, interpretive framework, sense-making). For example, Tikka et al. (2018) investigated which brain regions responded to narrative content regardless of the media form presenting the narrative content. Specifically, participants were presented either with a short movie or the script of the movie. The results revealed that responses in higher-level brain regions were similar despite the different physical input. Similarly, Nguyen et al. (2019) presented either a visual animation or narrated version of a Heider-Simmer-type social short story (Heider & Simmel, 1944). They again found similar responses independent of the presentation modality, and additionally similar brain activity between participants during story reception predicted similar interpretations.
In a similar vein, we may assume that recipients across the different language communities might still show similar responses in regions involved in sense-making and story cognition – regardless of whether a story, such as the famous ‘Le Petit Prince’, is presented as the French original, the Chinese version ‘小王子’, or the English ‘The Little Prince’ rendition. Although this reasoning is plausible and consistent with the latest research on brain responses to stories, only a few neuroimaging studies have examined these questions. Perhaps the strongest supporting evidence for cross-language similarities comes from a study by Honey et al. (2012), who played the same story to Russian and English listeners. However, this story was relatively short, the sample size was low, and the story was manipulated to be maximally comparable across languages. As such, there is a need for replications with larger and more diverse samples, and with different narratives (Turner et al., 2019). Therefore, the current study addresses the question of whether, where, and how temporally aligned audience brain responses arise in the brains of listeners exposed to translations of the same story (see Figure 1).
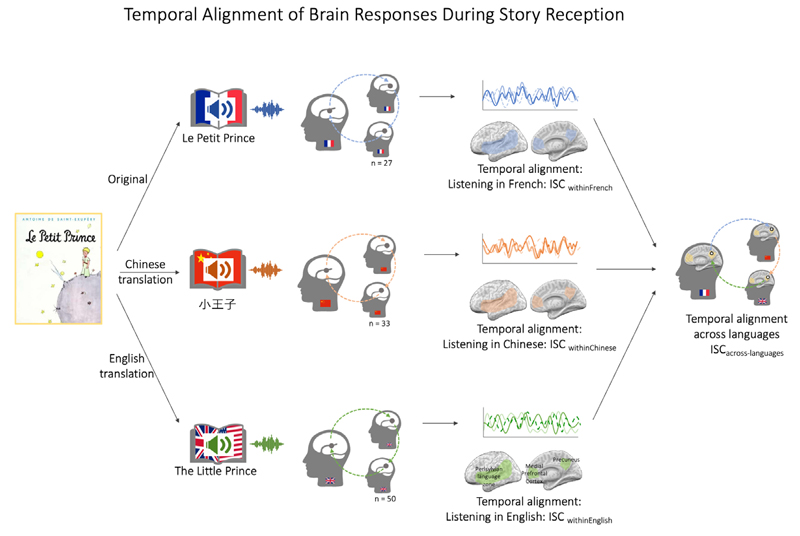
Conceptual overview of the study design and main predictionsNote. We expect that processing the same sounds, hearing and understanding the speech, and following the story events should evoke similar, temporally aligned brain responses along the sensation-to-cognition continuum, as indexed by robust inter-subject correlations (ISC) within each language group (H1a). Moreover, this effect should be similarly expressed across the three groups (H1b). To the extent that higher-level, post-perceptual brain regions are not responding to the immediate properties of the incoming stimulus but to more abstracted information, we can further expect that these regions should exhibit similar responses even across different-language versions of the same story (H2).
The Present Study and Hypotheses
This study examines how the brains of French, Chinese, and English listeners respond during the reception of the ‘Le Petit Prince’ story told in their respective languages. Based on the model discussed above, we were interested in examining temporally aligned brain responses to the same story within and across language communities.
First, we can expect that (H1a) listeners will exhibit strongly aligned responses to the specific story they are exposed to. Specifically, as French listeners listen to the French story, their brains should exhibit very similar neural processes (indexed by robust ISC) in brain regions involved in audition (because they receive the same auditory input and thus should respond with similar over-time responses to the timevarying input). The same applies to each of the other audiences composed of Chinese or English listeners, respectively. Moreover, there should be strong ISC in language-related regions (e.g., inferior frontal gyrus, medial temporal gyrus), again among (or within) members of each language community (because the story comes in a language that the recipients possess the necessary knowledge to decode/understand). Finally, there should be robust, albeit less strong ISC in regions beyond language-sensitive cortex. These include the medial frontal cortex, the anterior cingulate, the precuneus, and other parts of the salience, executive, or default mode system. In sum, we expect a pattern of ISC that follows the general sensation-to-cognition continuum – and this pattern should be evident for each of the groups (i.e., French listeners exposed to the story in the ‘Le Petit Prince’ version, Chinese Listeners exposed to ‘小王子’, and English listeners exposed to ‘The Little Prince Story’). A corollary of the expected distribution of ISC results across the brain is that we expect that the pattern of these shared responses5 will be similar across the three sub-audiences of French, Chinese, and English listeners (H1b). Put differently, we predict that the regions exhibiting high, medium, and low ISC should be the same across the independent ISC analyses for each audience. Therefore, H1b predicts a strong, significant correlation of the ISC pattern across the audience groups of French, Chinese, and English listeners.
In addition to predicting shared responses within each language group, we ask whether the brain response to the story will exhibit similarities across groups of people who process the same story told in a different language. Based on the above discussion, this should be the case – and particularly for regions located post-perception along the sensation-to-cognition continuum (H2: ISC across languages). Specifically, if brain responses to stories occur along the sensation-to-cognition continuum and shared neural responses (indexed by ISC) are contingent on whether the incoming stimulus content meets ‘matching’ knowledge structures6 that are shared between listeners’ brains, then we can expect ISC especially in regions that are located at postperceptual levels of the sensation-to-cognition continuum. Therefore, regions involved in integrating and making sense of higher-level story information should show commonalities in their neural responses, and these should occur among listeners of a given language version of the story and also across people exposed to the same story in different languages.
Overall, the main rationale of this study is that there should be a global, common, and temporally aligned neural response to the same story. In other words, regardless of the specific language in which a story is delivered to the brains of listeners, higher-level regions of their brains should exhibit similar processes because they do not simply respond to the auditory input (which indeed differs across languages), but receive a pre-transformed version of the story, one that is somewhat abstracted from the concrete acoustic input into a neural pattern or signature that we expect to be similar across listeners – regardless of the specific language they can speak and thus listened to. In particular, higher-level brain regions like the precuneus, which are not responding to the physical input’s immediate sensoryphysical properties, but rather to an internally transformed version of the input (as sense is made and meaning has been extracted from the sounds), should exhibit some common responses over time – regardless of the input language. Conversely, finding such across-language brain activity correlations despite the different auditory input would provide strong evidence that intersubjectively correlated brain responses are sensitive to higher-level story processes, thereby underscoring their relevance as a theoretical tool to study reception processes.
METHOD
This study uses data from the ‘Le Petit Prince’ data collection, a large, international, and multimodal dataset originally collected to examine questions in computational neurolinguistics (Li et al., 2021). Data are publicly available as ds003643 on the OpenNeuro platform. In the following, we provide an overview of the dataset, followed by a description of our analyses.
Sample. The original sample includes data from 116 participants (mean age = 21.5, sd = 3.2; 60 female, 30 French, 35 Chinese, and 51 English speakers), which were collected at the Inria Research Institute in Paris (French), Jiangsu Normal (Chinese), and Cornell University (English, i.e. Americans speaking English). To be included in the study, participants had to be native speakers of each language (French, Chinese, and English), pass standard MRI research requirements, and all participants provided informed consent to the IRB-approved study. Further details on the sample and inclusion criteria can be found in the data paper (Li et al., 2021). Data from individual participants were excluded if they were either marked as problematic (e.g. incomplete, scanner failure) in the OpenNeuro repository or if their functional data were shorter than that of the majority of other same-language participants. This led to a final sample of 27 French, 33 Chinese, and 50 English listeners, respectively.
Story Stimulus and Procedure. Al l participants listened to the same story, the famous book ‘Le Petit Prince’ by Antoine St. Exupery. Critically, however, the same story was read in French, Chinese, or English, respectively. The entire scanning session lasted about 1.5 hours and contained 9 subsections lasting ca. 10 minutes each, during which a part of the story was read. Each subsection covered the same part of the story across different versions, thus leading to slightly different lengths across the corresponding segments (e.g., the first segment lasted for 10 min 18 sec in French, 9 min 26 sec in Chinese, and 9 min 24 seconds in English). Each section was followed by comprehension/attention check questions.
Data Acquisition and fMRI Preprocessing. All data were acquired with 3T MRI scanners, including an anatomical T1 scan (MP-RAGE) and multi-echo planar functional imaging with a TR of 2.0 seconds. Preprocessing was carried out using AFNI, including skull-stripping, nonlinear anatomical normalization to the MNI coordinate system, realignment, slice-time correction, coregistration, and normalization of the functional data. Further details can be found in the datadescriptor paper (Li et al., 2021).
Data Analyses. Data Extraction. All analyses were conducted using NiLearn and BrainIAK packages (Abraham et al., 2014; Kumar et al., 2020) and in-house code. The analysis pipeline is documented in the form of reproducible Jupyternotebooks at https://github.com/ nomcomm/ littleprince_multilingual. In brief, functional data were downloaded, high-pass filtered at 0.01 Hz, detrended, and standardized. Next, averaged regional time series were extracted from individual brain regions spanning the entire brain in order to be able to peek into sensory, perceptual, and cognitive brain systems. Specifically, we extracted functional time series using the 268 node cortical parcellation provided by Shen et al. (2013), which has been used for analyzing fMRI data during movie-watching and narrative listening. We further added 25 anatomically defined subcortical regions (Edlow et al., 2012; Pauli et al., 2018). Thus, the basic data structure comprised fMRI time series data extracted from 293 regions, from 27, 50, and 33 French-, English-, or Chinesespeaking participants, and from 9 story segments (with ca. 300 fMRI volumes each).
Data Resampling Procedure. Our primary analysis necessitated a standardization of fMRI data duration across languages. This requirement arose from the inherent variability in the length of story subsections across different languages. For instance, while section #8 showed good consistency in duration across French, Chinese, and English versions (293, 294, and 295 TRs, respectively), other sections exhibited an average disparity of 13 TRs (please refer to Supplementary Materials for a detailed breakdown). This temporal inconsistency posed a challenge for temporal inter-subject correlation techniques, which demand uniform time series lengths to facilitate cross-brain fMRI data comparisons. While there are several ways to address this type of temporal mismatch, we implemented a resampling strategy (see Supplementary Materials for a discussion). In this strategy, each language group's data was adjusted to align with the mean duration across all three groups. As an example, for the aforementioned eighth section, all data were standardized to 294 samples. We conducted additional control analyses as detailed in the Supplementary Materials to ensure that our approach was robust, accurate, and avoided overfitting that other approaches are prone to. Furthermore, we extended this resampling procedure to the extracted acoustic RMSE feature. We then replicated our original analyses using this resampled acoustic data. This step was crucial to confirm that the correlation between acoustic features and neural activity remained intact post-resampling. Our results corroborated this preservation of correlation. We also explored other options to align the data based on semantics or utilize alternative procedures such as intersubject pattern correlations (See Supplementary Materials). However, we found the current approach (though not without caveats and limitations that will be discussed below) to be the most straightforward and transparent.
Inter-Subject Correlation (ISC) Analysis: Temporally Aligned Brain Responses Among Listeners. Shared neural responses within and across languages were assessed via inter-subject correlation methods, which yield the main results for this study (H1 and H2). In brief, the ISCanalysis we use identifies temporally correlated responses across the brains of audience members who are exposed to the same message (Hasson et al., 2004; Nastase et al., 2019; Schmälzle & Grall, 2020a). ISC analyses were performed following previously reported procedures based on the implementation of ISC functions in the brainIAK and nltools package (Chang, 2018; Kumar et al., 2020), and documented code is available at https://github.com/nomcomm/littleprince_ multilingual, along with a brief overview of provided in the Supplementary Materials.
ISC within languages: First, separate ISC analyses were carried out for data from each language subgroup (ISCFrench listeners, ISCChinese listeners, and ISCEnglish listeners). Specifically, regional data were z-scored, the first and last 10 volumes were removed to avoid ISC-contamination by transients, and ISC results were tested against phase shifted pseudo-ISC based on 500 permutations. As for the RMSE analysis, we again confirmed that the resampling did not yield different results (see Supplementary Materials).
ISC across languages: Second, after computing ISC analyses within each language group, we proceeded to test for common brain responses across languages. To this end, we combined data from all 110 participants, ran ISC-analyses between all possible pairs of listeners, then collapsed pairwise ISC results based on whether participants were listening to the same stimulus (i.e. French-French, Chinese-Chinese, and English-English pairings, called ISCwithin-language) or whether participants were listening to the same story conveyed in different languages (i.e. between English-French, French-Chinese, or Chinese-English listeners, called ISCacross-language; see Figure 1). Of note, this analysis is only feasible with temporally resampled data and thus all results are based on data that were brought to such a common timeline.
Inter-Subject Functional Connectivity (ISFC) Analysis: Exploring Networked Brain Dynamics. In addition to the main ISC analysis, which reveals how different brains respond similarly to the same messages in corresponding brain regions (e.g. similarity among French listeners, among Chinese listeners, or among English listeners, or even across the listeners exposed to differentlanguage versions of the same audiobook), other kinds of neuroimaging analyses offer insights into brain dynamics, like how different regions operate similarly or differently. Broadly speaking, this is known as connectivity analysis, and conventional connectivity analysis compare brain activity time series from different regions of the same brain. However, an inter-subject extension of connectivity analysis, called intersubject functional connectivity (Simony et al., 2016) extends the ISC approach, thus examining e.g. how auditory cortex in one listener responds not only to auditory cortex in another listener (i.e. ISC of auditory cortex), but also how the auditory cortex in one listener relates to e.g. frontal cortex activity in all other listeners. To gain insight into these higher-level relationships of brain regions during story processing, we also carried out an ISFC analysis. However, due to the complexity of these results, we present them largely in the supplementary materials as well as the online reproducibility package.
Statistical Significance Assessment. To assess the statistical significance of the ISC results, we performed a phase-shift ISC procedure with 1000 randomizations (Lerner et al., 2011; Nastase et al., 2019); after obtaining a p-value for the observed ISC compared to the null distribution, we further performed a multiple comparison correction using the false discovery rate procedure (q = 0.05; Benjamini & Yekutieli, 2001). This correction for multiple comparisons was applied for all ISC analyses we computed, i.e. the within- ISC analyses for each language (English, French, and Chinese), the individual story subsections, as well as for the within- and across-ISC analyses. In the accompanying reproducibility package and the Supplementary Materials and Methods, we provide further details and examine the robustness of the results with different procedures to ensure statistical significance of ISC results.
RESULTS
Correlations between Acoustic Features and Brain Activity. Before presenting the results from inter-subject correlation analyses, which compare the temporal alignment of brain activity between listeners (i.e., a listener-to-listener or brain-tobrain correlation), we first demonstrate that the stories indeed evoked expected brain activity in auditory brain regions. To this end, we perform a different kind of analysis (not the brain-to-brain ISC-type analysis), specifically one that links a quantified parameter of the auditory input (the sound envelope) to the brain activity this evokes (see Schmälzle et al., 2022). Specifically, it is wellknown that fMRI activity in the auditory cortex tracks with the time-varying acoustic properties of the incoming speech signal.
To confirm that this is the case in the French, Chinese, and English datasets, RMSE scores were extracted from the raw audio recording, down sampled to match the resolution of the fMRI signal, shifted by two TRs to account for delays in the hemodynamic response function, and correlated against the fMRI time courses from each brain region (Honey et al., 2012; Schmälzle et al., 2015). As shown in Figure 2, the RMSE sound energy of each story correlates significantly with the fMRI activity in the bilateral superior temporal cortex, matching the location of the auditory cortex. Attesting to the effect’s robustness, we can see converging results for all three language groups and each of the nine story chapters. In the supplementary materials and the online reproducibility package, we provide further details.
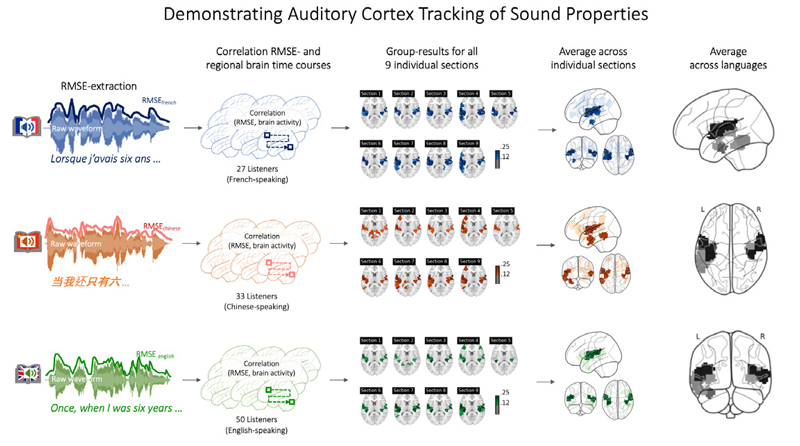
Confirming that brain activity tracks with the acoustic feature (RMSE) for all three story versions and for each of the nine chaptersNote. To demonstrate the integrity of the dataset and our processing pipeline, we extracted the root-mean-squared-energy (RMSE) of the soundtrack from each version of the audiobook (i.e. French, Chinese, and English). This RMSE-time course was then correlated against the regional brain activity from French, Chinese, and English listeners. As each audiobook was split into nine sections, this procedure was carried out for each section to demonstrate consistency. The results confirm that brain activity in auditory regions tracks robustly with the RMSE feature, and that this effect was consistent across runs and across listener groups. See Supplementary Materials for further details. Note that these results represent the correlation between the RMSE-feature and the brain activity. Going forward, result images will display inter-subject correlations (i.e. brain regions colored in will mean that there is a temporally correlated response across participants).
Alignment of Brain Responses Within Each Language Group (H1)
Having demonstrated that the audiobook evoked the expected brain activity, we next moved on to the first main analysis: To address H1, we computed ISC analyses within each group of French, Chinese, and English listeners, respectively. Consistent with previous studies, the results confirmed that many regions exhibited similar neural time courses across listeners. As shown in Figure 3, listening to the story in each language prompted strongly correlated neural responses in early auditory areas (e.g., Heschl’s gyrus, primary auditory cortex), linguistic (e.g., perisylvian language regions), and extralinguistic regions (e.g., medial and lateral frontal cortex, anterior and posterior cingulate cortex, precuneus) – and this was the case regardless of whether the story was presented in French, Chinese, or English. Moreover, the spatial distribution and strength of these shared responses confirmed predicted patterns, i.e., strong ISC in auditory/early language regions (MTG, STG) and weaker, but still significant ISC in higher-level regions.
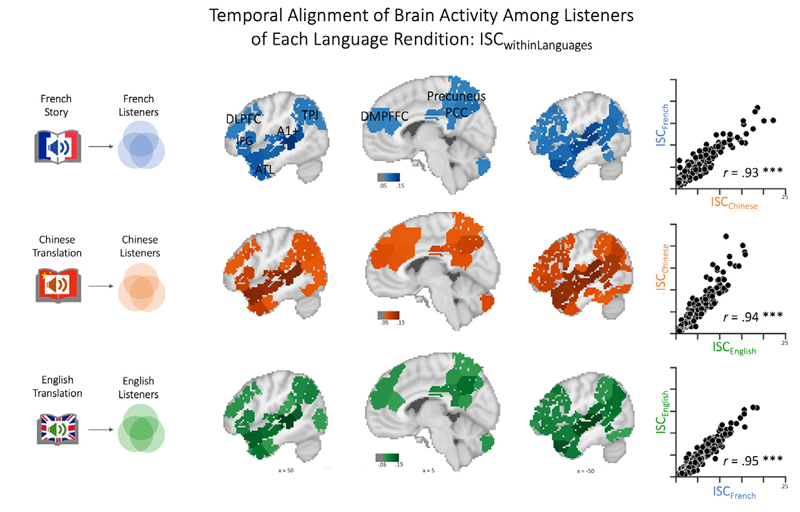
Collective alignment of brain activity among members of each language group (Within-Language-ISC)Note. Correlating brain activity time courses among all participants exposed to the same audiobook (i.e. within each language community) reveals significantly aligned processing in widespread brain regions, including early auditory regions (primary auditory cortex, BA41/52), linguistic areas (including STG, angular gyrus, and IFG), and extralinguistic regions (including precuneus, medial and dorsolateral cortex, and prefrontal cortex). Results are significant and corrected for multiple comparisons using the phase-randomization and FDR procedures (see Supplement for additional statistics). The scatterplots to the right demonstrate that the pattern of inter-brain alignment is highly similar (p < .001) across the three independent analysis streams, demonstrating that the same regions are either strongly, moderately, or weakly aligned. Note that the sagittal brain sections represent the average across pairwise ISC across all nine sections, which have also been additionally thresholded at r = .05 for better illustration. See text for further details and Supplementary Results for the ISC maps corresponding to each of the nine subsections. (DLPFC - Dorsolateral Prefrontal Cortex, DMPFC - Dorsomedial Prefrontal Cortex, IFG - Inferior Frontal Gyrus, ATL - Anterior Temporal Lobe, A1+ - Extended Early Auditory Cortex, PCC - Posterior Cingulate Cortex).
Figure 3 also shows that the spatial distribution of ISC results for the three different language groups was highly similar. This was statistically confirmed by highly significant correlations of the ISC result patterns across the 293 brain regions: Specifically, the ISC results for French and Chinese listeners were correlated at r = 0.93, French and English listeners’ ISC results correlated r = 0.95, and r = 0.94 for Chinese- English results (all p’s < .001). These results support H1b.
Furthermore, these effects were not just present in the overall results, but also within each of the nine subsections – thus demonstrating very high consistency (See Supplementary Results). Moreover, in addition to computing these ISC analyses on the original subsections, we also ran the same set of analyses for the resampled data (i.e. the data that had been stretched or compressed to make them equally long across language groups). As expected, we find that the pattern of ISC results matches the one reported for the original data (see Supplementary Results).
Exposing Aligned Neural Responses to the Same Story Told in Different Languages (H2)
Next, we tested whether the same story, even when told in different languages, would evoke similar responses across listeners, especially in higher-level, post-perceptual brain regions such as the precuneus (H2). To this end, we first began exploring the data from section 4, as this was the version of the book that naturally had almost the same length. As shown in Figure 4, examining data from the precuneus via split-half and groupaveraging, we find evidence that a shared signal emerges even across the brains of listeners exposed to different-language versions of the same story.
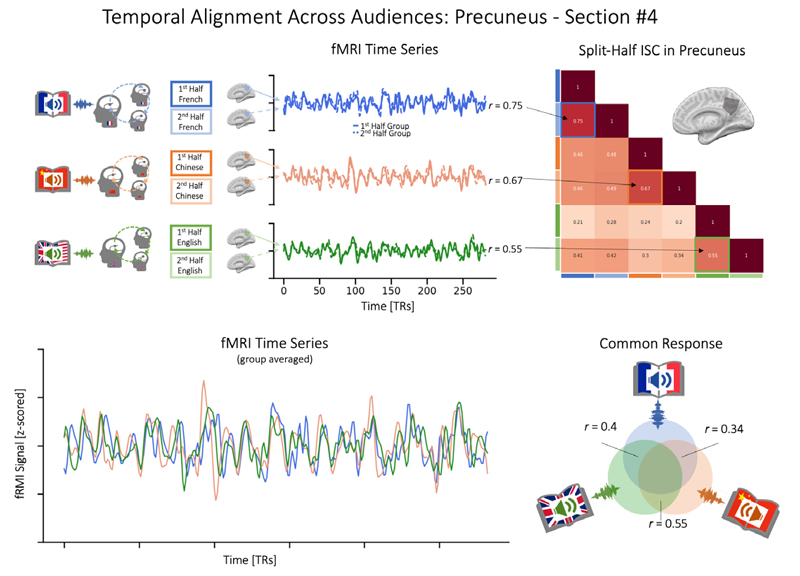
Aligned Neural Processes Across Language GroupsNote. Top panels: Each group’s fMRI data were averaged across half of the group (for visualization purposes only), and correlations were assessed within and across groups. Bottom Panels: A shared response across groups is visible once data are averaged across all participants who were exposed to each version of the book. Due to different group sizes, the signal-to-noise ratio differs, and data were z-scored individually and for visualization on a common timeline. Note that the data for section #4 of the audiobook required almost no resampling, as the different language versions were already of the same length.
The results from this analysis suggest a common signal, but they use averaging to boost the signalto- noise ratio and thus optimize the conditions for detecting (and visualizing) it. Thus, to test our hypothesis also for all sections, all regions of the brain, and at the level of single listeners, we performed a pairwise ISC analysis across the combined dataset consisting of brain responses from 110 listeners (27 French, 33 Chinese, and 50 English). Specifically, we collapse the within- and across-language ISC from the ISCresult matrices (see Supplementary Methods for details)7. Moreover, to gauge the degree to which random time series could yield spurious positive across-language ISC results, we performed the phase-shift ISC analysis with 500 permutations to generate a null distribution (see Methods section).
The results, shown in Figure 5, reveal acrosslanguage ISC, thereby supporting H2. Specifically, we find that as French, Chinese, and English listeners listen to the French, Chinese, or English version of the same story, their brain activity in the precuneus exhibits similar responses over time. Of note, the across-language ISC in the precuneus is clearly weaker than the within-language ISC, but it is still present and can be made visible via group averaging. As can be seen from Figure 5, significant across-language ISC is also present in other regions, such as the anterior and middle temporal lobes, the dorsomedial prefrontal cortex, and the temporo-parietal junction. Many of these regions are part of the default mode network, which is involved in higher-level processes, and thus, these results are also consistent with H2.
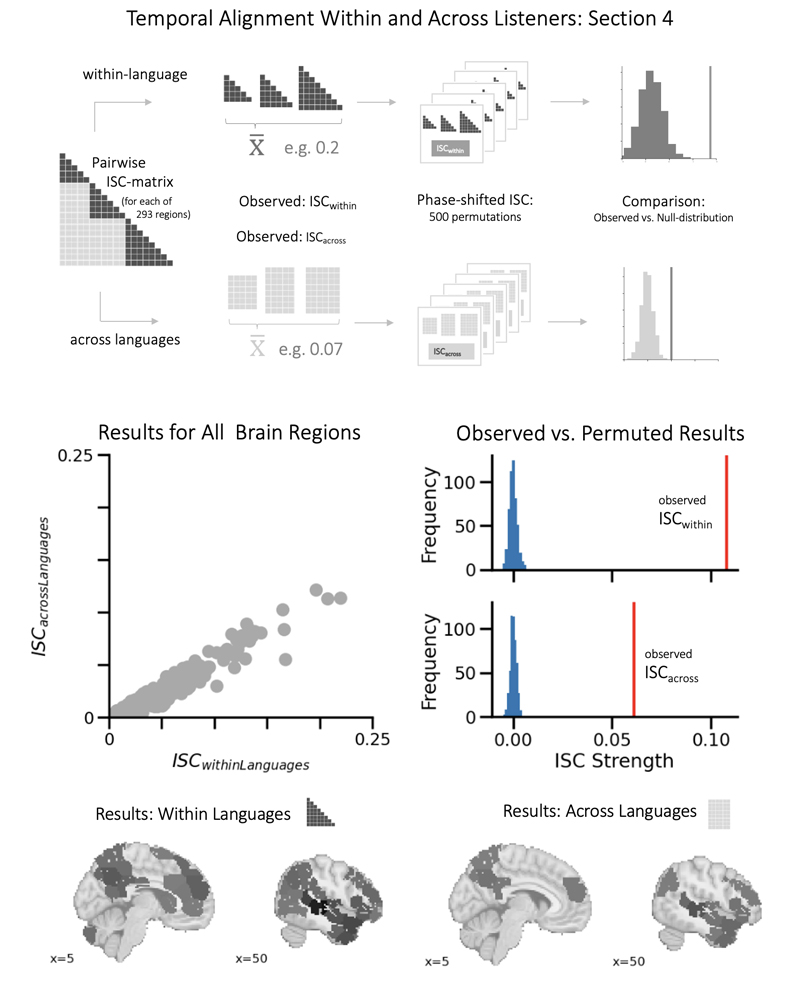
Aligned Processes Across Language GroupsNote. Top panel: Illustration of the analysis stream that splits up pairwise ISC data into within-language and acrosslanguage comparisons and compared with a surrogate distribution. Middle left panel: Scatterplot comparing the strength of ISC for the within and across-language comparison. As can be seen, the within-language comparison is stronger (all values below the diagonal), but the across-language results are also positive for some regions. Middle right panel: Results for one selected region, the precuneus. Bottom left panel: ISC within languages plotted on brains (compare Figure 3). Bottom right panel: ISC across languages (Results are statistically significant and additionally thresholded at r = 0.04 to show only stronger ISC).
However, the across-language ISC for auditory regions (see Figure 5) was unexpected and thus was followed up on in a series of control analyses (see Supplementary Materials and Results). Also, we note that there was also some evidence of differential coupling between sub-audiences and variability over the course of the story. Specifically, not all nine sections exhibited across-language ISC, and we conducted follow-up analyses to examine this issue in greater detail. It appears that those sections in which the French, Chinese, and English versions had a comparable length showed higher ISC, which raises some questions about the resampling and alignment for the across-language analysis in those runs that differed in length (see Figure 6 & Supplementary).
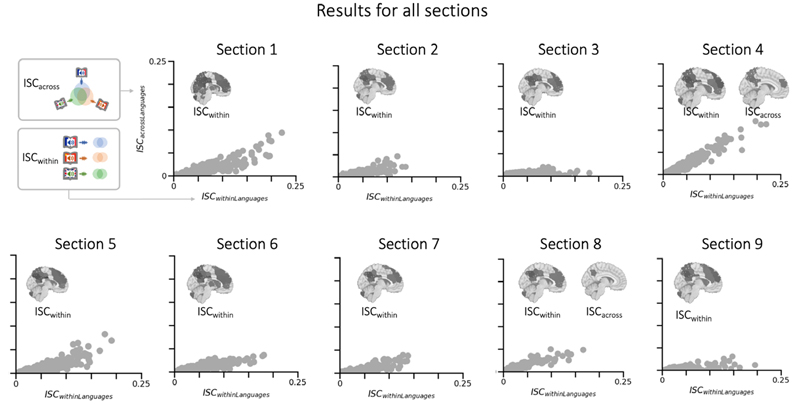
Aligned Processes Within Across Language Groups for All Nine SectionsNote. The scatterplots show the ISC for all 293 studied brain regions, with the x-axis representing the within-language ISC and the y-axis the across-language ISC. As can be seen, the within-language ISC is far stronger, but there is also evidence that for some regions, across-language ISC emerges in the same regions. The inserted brain-plots show significant within-language ISC (tested via a 500-fold phase-scrambling distribution) and across-language brain plots are shown for the sections where they survived statistical corrections.
DISCUSSION
This study reveals how the same story aligns the brain activity of listeners. This inter-subjective alignment of neural processes is seen when French listeners listen to the French version of Le Petit Prince, when Chinese listeners listen to the Chinese version, and also when English listeners listen to the English version. Moreover, regions of the brain that are involved in sense-making and related integrative processes exhibit aligned responses even across the brains of listeners who process different versions of the same story. Below, we first review and interpret these findings and then discuss implications for neuroscientifically grounded explanations of story reception and communication more broadly.
Story-Induced Neural Alignment Within and Across Language-Specific Audiences
The strong temporal alignment between the brains of listeners who were exposed to a given story told in their respective language (i.e. ISCEnglish-English, ISCFrench-French, and ISCChinese-Chinese, or simply ISCwithin-language) supports H1. The strength and regional distribution of within-language ISC also followed the expected pattern (H1a), being strongest in auditory and lower-level language regions, but still significant in areas of the default mode, saliency and executive networks. Moreover, the similarity of these regional ISC patterns confirms H1b.
The most interesting result of this study relates to the across-language ISC, which supports H2. This ISCacross-language indicates temporal similarities of brain responses among participants who listened to different-language versions of the same story. These results are compatible with the idea that the precuneus, as well as default-mode regions more broadly, are involved in integrating higher-level information over extended time scales and supramodal, and extralinguistic information (Chang et al., 2022).
These results also provide a conceptual replication and extension of earlier results by Honey and colleagues (Honey et al., 2012). Specifically, these researchers presented direct translations of the same story to English and Russian listeners. The current study further expands the range of languages studied (adding French and Chinese, a language from a very different language family), and it also extends the findings to a different story and a larger sample.
Theoretical Implications for Communication Neuroscience
These findings hold broader significance as they contribute to a neurally plausible understanding of communication as discussed in the extended neurocognitive network model (Schmälzle et al., 2022), or by Gasiorek and Aune (2019) with regard to aligned conceptual systems. Although definitions of communication vary, a unifying element is the notion that ideas are conveyed and lead to shared information, which is also reflected in the Latin origin of the word ‘communis’ (meaning ‘shared’; Gleick, 2011; Hasson et al., 2012; Littlejohn & Foss, 2009; Schmälzle & Grall, 2020a). For example, the story medium enables the preservation and transmission of the author’s original thoughts to a large audience that is distant in space and time. From the perspective of the input (story content) and the output (media effects, narrative impact), we have ample evidence that such communication is possible. However, a significant explanatory gap remains regarding the underlying neural mechanisms by which this is achieved. Although more and more communication scholars are embracing a communication neuroscience approach (Coronel et al., 2021; Floyd & Weber, 2020; Hopp et al., 2023; Huskey et al., 2020; Kang et al., 2018; Turner et al., 2019), it is fair to say that most of the field’s theories still rely on hypothetical constructs that lack a neurocognitive and thus mechanistic explanation of how communication can occur.
The picture that emerges from this study is thus one in which the temporally aligned brain responses (i.e., ISC) indicate that the story has been successfully transmitted into the listeners’ brains (Zadbood et al., 2017). More specifically, this transmission involves many substages along the sensation-to-cognition continuum – from the successful transmission of acoustic information to the ear, the perceptual analysis of the sound (Wilson et al., 2008), various linguistic mechanisms related to word and sentence decoding (Brennan et al., 2012), and ultimately the comprehension of complex ideas (e.g., ‘stimulating meaning in the mind’; Beatty et al., 2009; or ‘understanding’; Gasiorek & Aune, 2019; Nguyen et al., 2019; Schmälzle et al., 2015). Although we admit that there are still many questions about how people comprehend, cognitively interpret, and are emotional affected by this particular story about the fictional little prince character (e.g., Thye et al., 2023), the current findings support the following: Coordinated and similar brain activity across different individuals offers neural evidence that communication, or at least elemental forms of it, has taken place. Noninvasive neuroimaging allows us to monitor brain responses during ongoing story reception across multiple regions (e.g., early auditory areas, language-processing regions, extralinguistic areas) without interruption. By utilizing the ISC approach to uncover how different brains align in response to shared content, we gain a powerful method for studying how messages are received on a collective level.
For example, if the auditory cortex of 99 listeners responds very similarly to the same spoken story, but another individual shows uncorrelated responses, then this suggests that this person did not hear the sounds and is functionally deaf. The same reasoning can be applied beyond auditory neurocognition to higher-level knowledge representations, such as shared linguistic knowledge. For instance, if a French listener heard the ‘Le Petit Prince’ story and a person from the United States heard the same story (i.e., the French version), then high ISC in language-related regions would emerge if the US listener had knowledge of the French language, but no ISC should emerge if the information transmission were blocked due to a lack of French knowledge. In fact, this is precisely the study design of Honey et al. (2012), which we were not able to test here because the French, Chinese, and English stories were only played to listeners of the same language. These thought experiments are intriguing, but their outcomes are predictable from the current understanding of brain systems for sound and language processing.
However, moving beyond acoustic or languagespecific knowledge structures, the presence of a cross-language ISC suggests that the shared story content induces convergent brain responses across participants and beyond language barriers. This points to regularities of information processing principles across brains. What are these shared processes about? Mounting evidence suggests that terms like ‘sense-making’, which have long been part of the discipline’s repertoire (Littlejohn & Foss, 2009), may provide adequate descriptions (Yeshurun et al., 2021), albeit it is difficult to put a single verbal label on the information transformations that regions like the precuneus are executing. However, research suggests that these processes operate over multiand supramodal inputs (Fuster, 2003; Mesulam, 1998), as well as over longer time scales (Hasson et al., 2015; Lerner et al., 2011); theoretical, modeling, and experimental work suggests a similar picture (Chang et al., 2022; Hasson et al., 2015; Kumar et al., 2023).
From a neurocognitive perspective, it is also interesting to point out the compatibility between the current results and work on artificial neural networks, such as the paralleldistributed processing (PDP) theory that formed the foundation for the current AI revolution (Mitchell, 2019; Wolfram, 2023). In particular, work flowing from a PDP perspective has long suggested that words and language serve as ‘pointers to knowledge’ (Hinton, 1984; Hinton & Shallice, 1991; McClelland & Rogers, 2003). This is also compatible with the use of word embeddings in natural language processing (Rong, 2014), which are in turn related to psychological approaches to measure meaning (Osgood et al., 1957). Moreover, recent work in multilingual machine translation suggests ideas like ‘hidden state vectors’, which represent meaning in forms that are not bound to a specific language but could be more universally shared. Although speculative, it seems quite plausible that the shared brain patterns across the brains of listeners from different continents are related to such a concept (Chen et al., 2017), and this would also make much sense from an evolutionary perspective (Hasson et al., 2020).
Current work in neurolinguistics, which uses advanced artificial language models (e.g., GPT) is obviously also relevant here. Compared to the current work, which is centrally about the notion of temporal alignment between the brains of different listeners, such work tends to take a different approach though: Specifically, using content-annotations provided by the language model (i.e., feature vectors), it is possible to see if those annotation predict brain activity data, much like we did with the RMSE feature, but now for more abstracted representations from the LLM. This approach can provide deeper insights into the likely representations and transformations that are carried out within specific regions. (Brennan, 2022; Hale et al., 2021; Thye et al., 2023). In sum, we view the inter-subject correlation approach taken here and the approach of languagemodel- based annotations of the stimulus as complementary. The latter has the benefit of providing more insight into content properties although it still grapples with quantifying more abstract pragmatic and semantic variables that likely matter for social cognition and story comprehension (but see Thye et al., 2023). Given the breathtaking advances in artificial language models, however, we expect rapid progress in this direction.
Limitations and Suggestions for Future Research
Although the ‘Le Petit Prince’ story is a rich and extremely popular story, it is only one single story. In addition to the need for a broader variety of story stimuli across contents and genres, one could also hope for a broader variety of languages. The current approach to scan brain activity to the same story told in three different languages is already an advancement over mono- and bilingual studies. However, given the fact that the LPP story has been translated into 382 languages, we currently have only about 1% of ‘coverage.’
One methodological issue that seems worth pointing out specifically is differences in length. For instance, the English story was almost always slightly shorter than the Chinese and French versions. This is relevant insofar as the ISC analysis requires that data be aligned to a common timeline. We overcame this problem by resampling all datasets to a common length. Conceptually, this amounts to stretching or compressing the data, which is supported by prior work but still comes with some caveats (Lerner et al., 2014). This approach is straightforward, but it assumes that the linear time transformation is appropriate. However, given what we know about language, this assumption may be problematic. Based on our own translation and data checks, we confirmed that the basic progression of the story’s event descriptions is coarsely aligned across languages (e.g., each of the nine segments started and ended with the same sentence, suggesting that although the resampling may not be accurate at the millisecond level, it is at least coarsely aligned with a +/- 5 second window, which is close to the resolution of fMRI and the even slower behavior of, e.g., the precuneus (Lerner et al., 2014; Stephens et al., 2013). Still, it will be interesting to improve on the ways in which the story text is time-aligned across languages and incorporate such refined alignment in the analysis of neuroimaging data. Although this is somewhat outside of the scope of communication and falls more under the purview of neurolinguistics, it would help resolve the neurocognitive computations beyond the fairly coarse-grained differentiation in sensory, perceptual-linguistic, and higher-level/extra-linguistic response systems.
Finally, it will be crucial to experimentally unpack the functional significance of the crosslanguage similarities observed in this study. Although this analysis offers intriguing insights, it remains secondary, and the data available for interpretation was limited to comprehension scores, which likely suffered from a ceiling effect. To fully understand the implications of these findings, future research must delve deeper into the relationships between brain responses and interpretations across different cultures (e.g. Momenian et al., 2024). This could involve directly comparing how individuals from diverse cultural backgrounds interpret the same stimuli or story. Such comparisons could reveal not only the shared neural processes that facilitate common interpretations but also the cultural nuances that lead to divergent understandings. For instance, exploring the specific aspects of a narrative or message that different cultures tend to agree or disagree upon could shed light on the cognitive mechanisms that underlie these cross-cultural similarities and differences. Are there particular themes, emotional responses, or moral conclusions that resonate universally, or do cultural frameworks significantly shape how stories are perceived and internalized? To answer these questions, experimental designs could include stimuli that are culturally neutral, alongside those that are culturally loaded, to observe how brain responses vary in each context (e.g. Hopp et al., 2023; Schmälzle et al., 2024).
Summary and Conclusion
In summary, the same story elicited temporally aligned neural processes across listeners. When hearing the story in their native language, listeners’ brains demonstrated the anticipated spatiotemporal alignment. Remarkably, we also observed this alignment across the brains of listeners processing different-language versions of the same story. These findings directly address a core question in communication: the neural mechanisms that enable stories to be transmitted and understood across different brains, regardless of language.
Disclosure Statement
No potential conflict of interest was reported by the author.
References
-
Abraham, A., Pedregosa, F., Eickenberg, M., Gervais, P., Mueller, A., Kossaifi, J., Gramfort, A., Thirion, B., & Varoquaux, G. (2014). Machine learning for neuroimaging with scikit-learn. Frontiers in Neuroinformatics, 8, 14.
[https://doi.org/10.3389/fninf.2014.00014]
- Beatty, M. J., McCroskey, J. C., & Floyd, K. (2009). Biological dimensions of communication: Perspectives, methods, and research. Hampton Press.
-
Benjamini, Y., & Yekutieli, D. (2001). The control of the false discovery rate in multiple testing under dependency. Annals of Statistics, 29(4), 1165–1188.
[https://doi.org/10.1214/aos/1013699998]
- Brennan, J. R. (2022). Language and the brain: a slim guide to neurolinguistics. Oxford University Press.
-
Brennan, J., Nir, Y., Hasson, U., Malach, R., Heeger, D. J., & Pylkkänen, L. (2012). Syntactic structure building in the anterior temporal lobe during natural story listening. Brain and Language, 120(2), 163–173.
[https://doi.org/10.1016/j.bandl.2010.04.002]
- Caucheteux, C., Gramfort, A., & King, J.-R. (2021). Disentangling syntax and semantics in the brain with deep networks. In M. Meila & T. Zhang (Eds.), Proceedings of the 38th International Conference on Machine Learning (Vol. 139, pp. 1336–1348). PMLR.
-
Chang, C. H. C., Nastase, S. A., & Hasson, U. (2022). Information flow across the cortical timescale hierarchy during narrative construction. Proceedings of the National Academy of Sciences of the United States of America, 119(51), e2209307119.
[https://doi.org/10.1073/pnas.2209307119]
-
Chang, L., Jolly, E., Cheong, J. H., Burnashev, A., & Chen, A. (2018). cosanlab/nltools: 0.3.11 (0.3.11) [Software]. Zenodo.
[https://doi.org/10.5281/zenodo.2229813]
-
Chen, J., Leong, Y. C., Honey, C. J., Yong, C. H., Norman, K. A., & Hasson, U. (2017). Shared memories reveal shared structure in neural activity across individuals. Nature Neuroscience, 20(1), 115–125.
[https://doi.org/10.1038/nn.4450]
-
Coronel, J. C., O’Donnell, M. B., Pandey, P., Delli Carpini, M. X., & Falk, E. B. (2021). Political humor, sharing, and remembering: Insights from neuroimaging. Journal of Communication, 71(1), 129–161.
[https://doi.org/10.1093/joc/jqaa041]
-
de Heer, W. A., Huth, A. G., Griffiths, T. L., Gallant, J. L., & Theunissen, F. E. (2017). The hierarchical cortical organization of human speech processing. Journal of Neuroscience, 37(27), 6539–6557.
[https://doi.org/10.1523/JNEUROSCI.3267-16.2017]
-
Edlow, B. L., Takahashi, E., Wu, O., Benner, T., Dai, G., Bu, L., Grant, P. E., Greer, D. M., Greenberg, S. M., Kinney, H. C., & Folkerth, R. D. (2012). Neuroanatomic connectivity of the human ascending arousal system critical to consciousness and its disorders. Journal of Neuropathology and Experimental Neurology, 71(6), 531–546.
[https://doi.org/10.1097/nen.0b013e3182588293]
-
Ferstl, E. C., Neumann, J., Bogler, C., & von Cramon, D. Y. (2008). The language network: A meta-analysis of neuroimaging studies on comprehension. Human Brain Mapping, 29(5), 581–593.
[https://doi.org/10.1002/hbm.20422]
- Floyd, K., & Weber, R. (2020). The handbook of communication science and biology. Routledge.
- Fuster, J. M. (2003). Cortex and mind: Unifying cognition. Oxford University Press.
-
Fuster, J. M., & Bressler, S. L. (2012). Cognit activation: A mechanism enabling temporal integration in working memory. Trends in Cognitive Sciences, 16(4), 207–218.
[https://doi.org/10.1016/j.tics.2012.03.005]
- Gasiorek, K., & Aune, G. (2019). Toward an integrative model of communication as creating understanding [Paper presentation]. International Communication Association Annual Conference, Washington, D.C., USA.
- Gleick, J. (2011). The information: A history, a theory, a flood. Knopf Publishing Group.
-
Goldstein, A., Zada, Z., Buchnik, E., Schain, M., Price, A., Aubrey, B., Nastase, S. A., Feder, A., Emanuel, D., Cohen, A., Jansen, A., Gazula, H., Choe, G., Rao, A., Kim, C., Casto, C., Fanda, L., Doyle, W., Friedman, D., ... Hasson, U. (2022). Shared computational principles for language processing in humans and deep language models. Nature Neuroscience, 25(3), 369–380.
[https://doi.org/10.1038/s41593-022-01026-4]
-
Grady, S. M., Schmälzle, R., & Baldwin, J. (2022). Examining the relationship between story structure and audience response. Projections, 16(3), 1–28.
[https://doi.org/10.3167/proj.2022.160301]
-
Grall, C., Tamborini, R., Weber, R., & Schmälzle, R. (2021). Stories collectively engage listeners’ brains: Enhanced intersubject correlations during reception of personal narratives. Journal of Communication, 71(2), 332–355.
[https://doi.org/10.1093/joc/jqab004]
- Green, M. C., Strange, J. J., & Brock, T. C. (2003). Narrative impact: Social and cognitive foundations. Taylor & Francis.
-
Hale, J., Campanelli, L., Li, J., Bhattasali, S., Pallier, C., & Brennan, J. (2021). Neurocomputational models of language processing. Annual Review of Linguistics, 8, 427–446.
[https://doi.org/10.1146/lingbuzz/006147]
-
Hasson, U., Chen, J., & Honey, C. J. (2015). Hierarchical process memory: Memory as an integral component of information processing. Trends in Cognitive Sciences, 19(6), 304–313.
[https://doi.org/10.1016/j.tics.2015.04.006]
-
Hasson, U., Ghazanfar, A. A., Galantucci, B., Garrod, S., & Keysers, C. (2012). Brain-to-brain coupling: A mechanism for creating and sharing a social world. Trends in Cognitive Sciences, 16(2), 114–121.
[https://doi.org/10.1016/j.tics.2011.12.007]
-
Hasson, U., Malach, R., & Heeger, D. J. (2010). Reliability of cortical activity during natural stimulation. Trends in Cognitive Sciences, 14(1), 40–48.
[https://doi.org/10.1016/j.tics.2009.10.011]
-
Hasson, U., Nastase, S. A., & Goldstein, A. (2020). Direct fit to nature: An evolutionary perspective on biological and artificial neural networks. Neuron, 105(3), 416–434.
[https://doi.org/10.1016/j.neuron.2019.12.002]
-
Hasson, U., Nir, Y., Levy, I., Fuhrmann, G., & Malach, R . (2004). Intersubject synchronization of cortical activity during natural vision. Science, 303(5664), 1634–1640.
[https://doi.org/10.1126/science.1089506]
-
Heider, F., & Simmel, M. (1944). An experimental study of apparent behavior. The American Journal of Psychology, 57(2), 243–259.
[https://doi.org/10.2307/1416950]
- Hinton, G. E. (1984). Distributed representations. https://kilthub.cmu.edu/articles/Distributed_representations/6604925/files/12095348.pdf
-
Hinton, G. E., & Shallice, T. (1991). Lesioning an attractor network: Investigations of acquired dyslexia. Psychological Review, 98(1), 74–95.
[https://doi.org/10.1037/0033-295X.98.1.74]
-
Honey, C. J., Thompson, C. R., Lerner, Y., & Hasson, U. (2012). Not lost in translation: Neural responses shared across languages. Journal of Neuroscience, 32(44), 15277–15283.
[https://doi.org/10.1523/JNEUROSCI.1800-12.2012]
- Hopp, F. R., Amir, O., Fisher, J. T., Grafton, S., Sinnott-Armstrong, W., & Weber, R. (2023). Moral foundations elicit shared and dissociable cortical activation modulated by political ideology. Nature Human Behaviour, 7(12), 2182–2198.
-
Huskey, R., Bue, A. C., Eden, A., Grall, C., Meshi, D., Prena, K., Schmälzle, R., Scholz, C., Turner, B. O., & Wilcox, S. (2020). Marr’s tri-level framework integrates biological explanation across communication subfields. Journal of Communication, 70(3), 356–378.
[https://doi.org/10.1093/joc/jqaa007]
-
Huth, A. G., de Heer, W. A., Griffiths, T. L., Theunissen, F. E., & Gallant, J. L. (2016). Natural speech reveals the semantic maps that tile human cerebral cortex. Nature, 532(7600), 453–458.
[https://doi.org/10.1038/nature17637]
-
Kang, Y., Cooper, N., Pandey, P., Scholz, C., O’Donnell, M. B., Lieberman, M. D., Taylor, S. E., Strecher, V. J., Dal Cin, S., Konrath, S., Polk, T. A., Resnicow, K., An, L., & Falk, E. B. (2018). Effects of self-transcendence on neural responses to persuasive messages and health behavior change. Proceedings of the National Academy of Sciences of the United States of America, 115(40), 9974–9979.
[https://doi.org/10.1073/pnas.1805573115]
- Kintsch, W. (1998). Comprehension: A paradigm for cognition. Cambridge University Press.
-
Kumar, M., Ellis, C. T., Lu, Q., Zhang, H., Capotă, M., Willke, T. L., Ramadge, P. J., Turk-Browne, N. B., & Norman, K. A. (2020). BrainIAK tutorials: User-friendly learning materials for advanced fMRI analysis. PLoS Computational Biology, 16(1), e1007549.
[https://doi.org/10.1371/journal.pcbi.1007549]
-
Kumar, M., Goldstein, A., Michelmann, S., Zacks, J. M., Hasson, U., & Norman, K. A. (2023). Bayesian surprise predicts human event segmentation in story listening. Cognitive Science, 47(10), e13343.
[https://doi.org/10.1111/cogs.13343]
-
Lerner, Y., Honey, C. J., Katkov, M., & Hasson, U. (2014). Temporal scaling of neural responses to compressed and dilated natural speech. Journal of Neurophysiology, 111(12), 2433–2444.
[https://doi.org/10.1152/jn.00497.2013]
-
Lerner, Y., Honey, C. J., Silbert, L. J., & Hasson, U. (2011). Topographic mapping of a hierarchy of temporal receptive windows using a narrated story. Journal of Neuroscience, 31(8), 2906–2915.
[https://doi.org/10.1523/JNEUROSCI.3684-10.2011]
-
Li, J., Bhattasali, S., Zhang, S., Franzluebbers, B., Luh, W.-M., Nathan Spreng, R., Brennan, J. R., Yang, Y., Pallier, C., & Hale, J. (2021). Le Petit Prince: A multilingual fMRI corpus using ecological stimuli. BioRxiv.
[https://doi.org/10.1101/2021.10.02.462875]
- Littlejohn, S. W., & Foss, K . A. (2009). Encyclopedia of communication theory. SAGE.
-
Mar, R. A. (2011). The neural bases of social cognition and story comprehension. Annual Review of Psychology, 62, 103–134.
[https://doi.org/10.1146/annurev-psych-120709-145406]
-
Margulies, D. S., Ghosh, S. S., Goulas, A., Falkiewicz, M., Huntenburg, J. M., Langs, G., Bezgin, G., Eickhoff, S. B., Castellanos, F. X., Petrides, M., Jefferies, E., & Smallwood, J. (2016). Situating the default-mode network along a principal gradient of macroscale cortical organization. Proceedings of the National Academy of Sciences, 113(44), 12574–12579.
[https://doi.org/10.1073/pnas.1608282113]
- McClelland, J. L., & Rogers, T. T. (2003). The parallel distributed processing approach to semantic cognition. Nature Reviews. Neuroscience, 4(4), 310–322.
- Menon, V. (2015). Salience network. In A. W. Toga (Ed.), Brain mapping: An encyclopedic reference (Vol. 2, pp. 597–611). Academic Press/Elsevier.
-
Mesulam, M. M. (1998). From sensation to cognition. Brain, 121(6), 1013–1052.
[https://doi.org/10.1093/brain/121.6.1013]
- Mitchell, M. (2019). Artificial intelligence: A guide for thinking humans. Penguin UK.
-
Momenian, M., Ma, Z., Wu, S., Wang, C., Brennan, J., Hale, J., Meyer, L., & Li, J. (2024). Le Petit Prince Hong Kong (LPPHK): Naturalistic fMRI and EEG data from older Cantonese speakers. Scientific Data, 11(1), 992.
[https://doi.org/10.1038/s41597-024-03745-8]
-
Nastase, S. A., Gazzola, V., Hasson, U., & Keysers, C. (2019). Measuring shared responses across subjects using intersubject correlation. Social Cognitive and Affective Neuroscience, 14(6), 667–685.
[https://doi.org/10.1093/scan/nsz037]
-
Nguyen, M., Vanderwal, T., & Hasson, U. (2019). Shared understanding of narratives is correlated with shared neural responses. NeuroImage, 184, 161–170.
[https://doi.org/10.1016/j.neuroimage.2018.09.010]
-
Nummenmaa, L., Lahnakoski, J. M., & Glerean, E. (2018). Sharing the social world via intersubject neural synchronisation. Current Opinion in Psychology, 24, 7–14.
[https://doi.org/10.1016/j.copsyc.2018.02.021]
- Osgood, C. E., Suci, G. J., & Tannenbaum, P. H. (1957). The measurement of meaning. University of Illinois Press.
-
Pauli, W. M., Nili, A. N., & Tyszka, J. M. (2018). A high-resolution probabilistic in vivo atlas of human subcortical brain nuclei. Scientific Data, 5, 180063.
[https://doi.org/10.1038/sdata.2018.63]
-
Rong, X. (2014). word2vec parameter learning explained. arXiv.
[https://doi.org/10.48550/arXiv.1411.2738]
- Saenz, M., & Langers, D. R . M. (2014). Tonotopic mapping of human auditory cortex. Hearing Research, 307, 42–52.
-
Schmälzle, R. (2022). Theory and method for studying how media messages prompt shared brain responses along the sensation-to-cognition continuum. Communication Theory, 32(4), 450–460.
[https://doi.org/10.1093/ct/qtac009]
-
Schmälzle, R ., & Grall, C. (2020). The coupled brains of captivated audiences: An investigation of the collective brain dynamics of an audience watching a suspenseful film. Journal of Media Psychology, 32(4), 187–199.
[https://doi.org/10.1027/1864-1105/a000271]
-
Schmälzle, R., Häcker, F. E. K., Honey, C. J., & Hasson, U. (2015). Engaged listeners: shared neural processing of powerful political speeches. Social Cognitive and Affective Neurosciences, 10(8), 1137–1143.
[https://doi.org/10.1093/scan/nsu168]
-
Schmälzle, R., Häcker, F., Renner, B., Honey, C. J., & Schupp, H. T. (2013). Neural correlates of risk perception during real-life risk communication. Journal of Neuroscience, 33(25), 10340–10347.
[https://doi.org/10.1523/JNEUROSCI.5323-12.2013]
-
Schmälzle, R., Lim, S., Wu, J., Bezbaruah, S., & Hussain, S. A. (2024). Converging crowds and tied twins: Audience brain responses to the same movie are consistent across continents and enhanced among twins. Journal of Media Psychology.
[https://doi.org/10.1027/1864-1105/a000422]
-
Schmälzle, R., Wilcox, S., & Jahn, N. T. (2022). Identifying moments of peak audience engagement from brain responses during story listening. Communication Monographs, 89(4), 515–538.
[https://doi.org/10.1080/03637751.2022.2032229]
-
Shen, X., Tokoglu, F., Papademetris, X., & Constable, R. T. (2013). Groupwise whole-brain parcellation from resting-state fMRI data for network node identification. NeuroImage, 82, 403–415.
[https://doi.org/10.1016/j.neuroimage.2013.05.081]
- Simony, E., Honey, C. J., Chen, J., Lositsky, O., Yeshurun, Y., Wiesel, A., & Hasson, U. (2016). Dynamic reconfiguration of the default mode network during narrative comprehension. Nature Communications, 7(1), 12141.
- Specht, K. (2014). Neuronal basis of speech comprehension. Hearing Research, 307, 121–135.
-
Stephens, G. J., Honey, C. J., & Hasson, U. (2013). A place for time: The spatiotemporal structure of neural dynamics during natural audition. Journal of Neurophysiology, 110(9), 2019–2026.
[https://doi.org/10.1152/jn.00268.2013]
-
Thye, M., Hoffman, P., & Mirman, D. (2023). The words that little by little revealed everything: Neural response to lexical-semantic content during narrative comprehension. NeuroImage, 276, 120204.
[https://doi.org/10.1016/j.neuroimage.2023.120204]
-
Tikka, P., Kauttonen, J., & Hlushchuk, Y. (2018). Narrative comprehension beyond language: Common brain networks activated by a movie and its script. PloS One, 13(7), e0200134.
[https://doi.org/10.1371/journal.pone.0200134]
-
Toneva, M., Mitchell, T. M., & Wehbe, L. (2020). Combining computational controls with natural text reveals new aspects of meaning composition. BioRxiv.
[https://doi.org/10.1101/2020.09.28.316935]
-
Turner, B. O., Huskey, R., & Weber, R. (2019). Charting a future for fMRI in communication science. Communication Methods and Measures, 13(1), 1–18.
[https://doi.org/10.1080/19312458.2018.1520823]
-
Wehbe, L., Murphy, B., Talukdar, P., Fyshe, A., Ramdas, A., & Mitchell, T. (2014). Simultaneously uncovering the patterns of brain regions involved in different story reading subprocesses. PLoS ONE, 9(11), e112575.
[https://doi.org/10.1371/journal.pone.0112575]
-
Willems, R. M., Nastase, S. A., & Milivojevic, B. (2020). Narratives for neuroscience. Trends in Neurosciences, 43(5), 271–273.
[https://doi.org/10.1016/j.tins.2020.03.003]
- Wilson, S. M., Molnar-Szakacs, I., & Iacoboni, M. (2008). Beyond superior temporal cortex: Intersubject correlations in narrative speech comprehension. Cerebral Cortex, 18(1), 230–242.
- Wolfram, S. (2023). What is ChatGPT doing... and why does it work? Stephen Wolfram.
-
Yeshurun, Y., Nguyen, M., & Hasson, U. (2021). The default mode network: Where the idiosyncratic self meets the shared social world. Nature Reviews Neuroscience, 22(3), 181–192.
[https://doi.org/10.1038/s41583-020-00420-w]
-
Yeshurun, Y., Swanson, S., Simony, E., Chen, J., Lazaridi, C., Honey, C. J., & Hasson, U. (2017). Same story, different story: the neural representation of interpretive frameworks. Psychological science, 28(3), 307–319.
[https://doi.org/10.1177/0956797616682029]
-
Zadbood, A., Chen, J., Leong, Y. C., Norman, K. A., & Hasson, U. (2017). How we transmit memories to other brains: Constructing shared neural representations via communication. Cerebral Cortex, 27(10), 4988–5000.
[https://doi.org/10.1093/cercor/bhx202]
Appendix
Appendix
Supplementary Materials
Inter-subject correlation analysis (ISC) is a method used in neuroscience and psychology to examine how similar brain activity is across different individuals while they engage in the same task or experience, here a common story. The basic idea behind ISC is to measure the correlation or similarity of temporal brain activity time series (or patterns) between different participants (see Figure S1). ISC computations were carried out following previously published procedures and general guidelines (Nastase et al., 2019), and we document analysis methods in the form of Jupyter notebooks. To address the main withinlanguage ISC, we computed ISC analysis between all listeners of a given story version (e.g., all 33 French listeners exposed to the first section of the book, the second section – or the concatenated sections), correlating the region-wise time courses (from each of 293 brain regions) between all listeners across the full duration of the story (or story subsection). This yields an ISC matrix for every brain region. Statistical significance was assessed using the phaseshift_isc procedure (1000 iterations). The resulting statistical values were corrected using the FDR procedure (q = 0.05) and used to threshold the ISC result maps for display. Details and code are available at https://github. com/nomcomm/littleprince_multilingual.
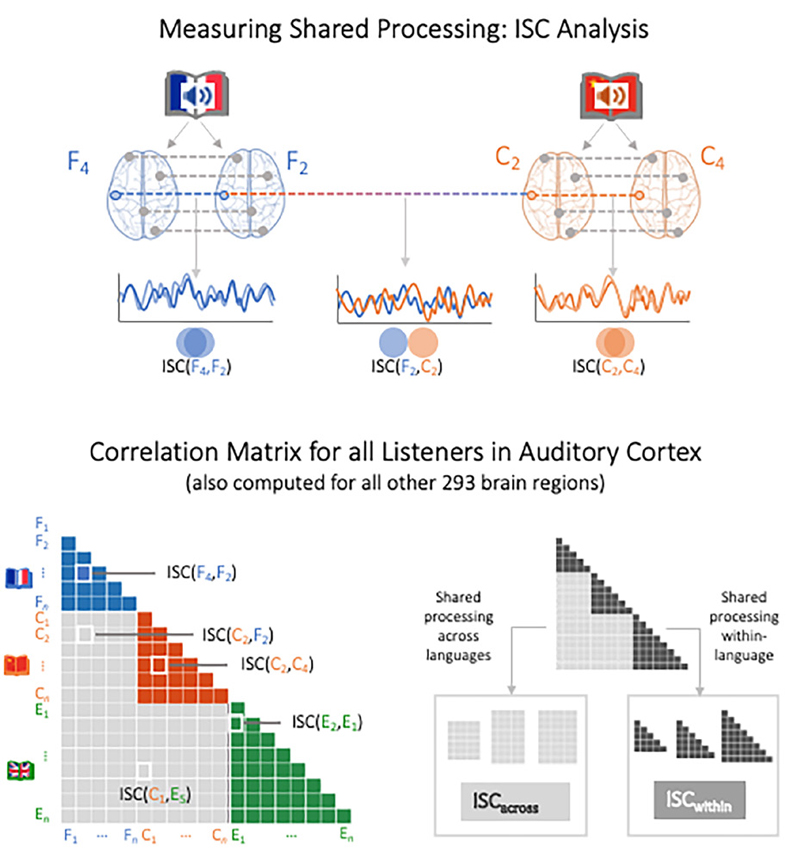
Theoretical rationale for data analysisNote. Top panel: Logic of ISC analysis: Correlating brain activity between corresponding regions from listeners’ brains to reveal shared processing. Middle panel: Computing all pairwise correlations across all pairs of listeners from French, Chinese, and English groups allows examining the ISC in response to the same physical stimulus (i.e. audiobook in French, Chinese, and English), called ISCwithin, and to the same story told in different languages, called ISCacross. Bottom panel: For the auditory cortex, we expect that the type of sound input (i.e. whether recipients process the same or different language versions) will evoke similar auditory responses among those recipients who listen to the same language version of the audiobook. For higher-order regions, such as the precuneus, similar responses should be less dependent on concrete stimulus properties (e.g. sound characteristics), but on abstract similarities. Since the content conveyed by the story is practically identical across different language versions, we can expect that brain activity time courses in these regions are similar across listeners even though they might be listening to different versions of the same audiobook.
The Le Petit Prince story, with its French, Chinese, and English versions, was split into 9 sections. Each section lasted about 10 minutes, which is also a duration that helps participants lie still in the scanner for this time and relax a bit in between the sections. Although the individual French, Chinese, and English sections were roughly the same duration (see Figure S2), they differed slightly in length. Two runs (4 and 8) are naturally almost identical in length, whereas others varied more. However, a prerequisite of the standard ISC analysis is that the data have the same length. Hence, we resampled the fMRI data to a common length for each section, and in doing so also corrected for slight differences in acquisition times across sites.
To implement this resampling, we used temporal resampling as implemented in the SciPy signal analysis module (Gommers et al., 2022; see the online repository for corresponding notebooks: https://github.com/nomcomm/ littleprince_multilingual/). The resulting run lengths were 291, 315, 338, 308, 283, 371, 340, 293, and 368 TRs (TR was 2000 msec across all three sites), respectively.
We note that although this resampling procedure is straightforward and possible to implement, it comes with some caveats as it dictates a common length on the three different language versions. Thus, while this approach helps to formally fulfill the criteria for ISC analysis (enabling equal-length time series to be correlated across language versions) and preserves the underlying semantic progression of the story, the evolution of neurocognitive reception responses may be somewhat blurred by this approach. To address this concern and explore alternative approaches, we carried out extensive additional analyses. Specifically, we explored data alignment on a by-sentence basis, using modern transformer-based translation modules and BERT vectors. However, we found that these approaches, though elegant in theory, raised challenges in practice. Specifically, since sentences do not align with the TR (sampling rate of fMRI), it would require resampling the TRs, often partially (to match sentence boundaries), and then compute weighted averages (leading to a sort of interpolation or resampling of the fMRI data to align with the story content). This proved problematic in practice, and we ran into problems like divergent numbers of sentences across languages, which ultimately did not solve the alignment problem but only shifted it to another level.
Alternatively, we also explored using patternbased analyses, which have shown some promise in similar contexts (e.g., Chen et al., 2017). However, conducting these pattern analyses with the regional parcellation (293 brain regions) is too coarse-grained, and previous patternbased analyses were often carried out using e.g., searchlight approaches or voxel-pattern analyses using data from the single areas. We attempted to implement this approach using patterns from the precuneus, but found no pattern alignment, which could be due to prior preprocessing procedures or cross-brain anatomical differences.
Thus, while these analyses yielded no superior approach, we opted to keep with the straightforward linear resampling (expansion/ compression) approach. However, to demonstrate that this approach, which may inevitably cause some blurring of temporal patterns, does not interfere with the basic logic of the analysis, we also applied the resampling to the auditory waveforms used to demonstrate auditory speech tracking. This demonstrated that the resampling preserved the basic auditory tracking results (see below). 3. Neutral message
Results reported in the main text demonstrate that the fMRI signal in the auditory cortex robustly tracks with the RMSE auditory feature, i.e., the signal in the auditory cortex goes up and down depending on the energy of the auditory input, confirming that the sound arrived in this auditorysensory brain regions (Honey et al., 2012). As discussed above, the resampling procedures applied to the fMRI data lead to an expansion or compression of the brain activity time courses (Lerner et al., 2014). Hence, it is important to demonstrate that this auditory tracking can still be detected even after resampling. To this end, we carried out the same procedures described to demonstrate that this effect is still present after resampling. As shown in Figure S3, this was the case. Thus, although the resampling procedure inevitably expands or compresses the data, the auditory tracking of the sound envelope still tracks with the measured fMRI response.
The within-language ISC results reported in the main text were based on computing a separate ISC analysis for every section. Moreover, we used the popular phase-shifting method to compute statistical thresholds and averaged the final results across all nine sections for presentation (Nastase et al., 2019) . This approach succinctly summarizes complex data, but some readers may want to examine the results at a more fine-grained level. In Figure S4, ISC-result maps are thus shown for every individual section of the audiobook as heard by the French, Chinese, or English listeners, respectively. As can be seen, significant ISC, indicating temporally aligned brain responses, was present in every individual section and for every language. Moreover, the cortical distribution of ISC was highly similar across different story sections and even across the languages in every subsection.
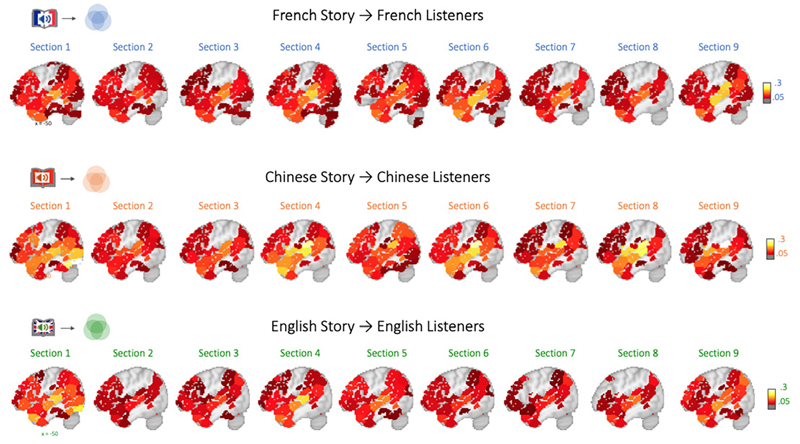
Within-language ISC analysis at finer scale: Temporally aligned processing within each language community across all nine sections
In addition to examining the results at the level of individual sections, we also examined the results using different statistical methods and using resampled vs. non-resampled data. First, a bootstrap-based approach has been suggested for ISC statistics (Nastase et al., 2019) . Thus, we also conducted the same analysis using this approach, finding practically identical results (see Figure S5, left panel).
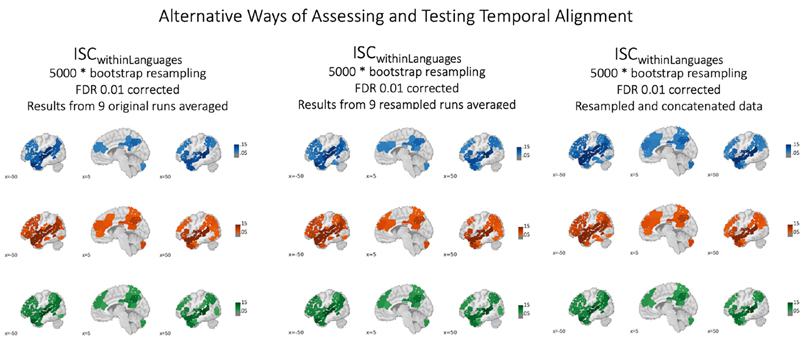
Within-Language ISC Control AnalysesNote. Within-language ISC results are the same with or without resampling and also hold when data are concatenated into one continuous time series.
Furthermore, although the resampling is less of a concern for the within-language data as one can assume that this expands or compresses all data in a similar way, we still wanted to demonstrate that results stay the same when computed with original or resampled data, respectively (Lerner et al., 2014). As shown in the middle panel in Figure S5, this was also the case.
Lastly, while the data had been collected in nine separate runs, and thus computing ISC over each run and averaging the result is an obvious strategy, an alternative strategy is to concatenate the data into one single continuous time series and compute ISC over the entire, concatenated data. In theory, the results should be similar as long as the strength of ISC coupling does not vary too much between sections, but it is still important to examine this empirically. Empirically, we find that ISC is robust and follows the same pattern as for the previous methodological variants (see Figure S5, right panel). Taken together, results from these additional analyses fully support the main analyses from the main text.
Control Analysis Description: Because we were worried that part of this effect could be driven by preexisting similarities in the auditory input, we performed further control analyses. Specifically, we jointly examined the auditory signals themselves (i.e., the cross-correlations between RMSE time courses from the three languages), the coupling between the RMSE feature and brain activity from each language group (e.g., RMSE-French to fMRI from French, Chinese, and English listeners, etc.), and the ISC (i.e., fMRI signal correlations between French, Chinese, and English listeners). This control analysis confirmed that the RMSE signal was always most highly correlated against the fMRI time courses from the same-language listeners (green boxes). By contrast, RMSE-time courses from one language (e.g., French RMSE) correlated only weakly with the auditory cortex fMRI signal from the two other language groups (e.g., Chinese and English listeners - blue boxes). After all - the French RMSE never entered the brains of Chinese or English listeners - since those samples were acquired on different continents. However, a noteworthy finding of these analyses was that there are correlations between the French, Chinese, and English listeners’ auditory cortex fMRI time courses. Moreover, this correlation between auditory fMRI activity from French, Chinese, and English listeners appears even higher than what one would expect based on the correlations of either the French, Chinese, or English RMSE features or from the coupling between each group’s RMSE-signal and their brain activity. Although we are cautious to interpret these data cautiously (because correlations are not transitive), this could be taken as evidence for top-down attentional effects, i.e., that higher-level factors prompt more similar activity in auditory regions beyond what is explained solely by bottom-up processing.
The auditory cortex provides an interesting test case to examine whether across-language ISC is different, as one may expect given the different sound input. Figure S6 shows the results of these analyses for an early auditory cortex region (shown here again for section #4, which was selected for illustrative purposes). As can be seen, there is robust evidence for ISC within each language group, as one could expect based on the analyses above testing H1. Moreover, the block-like structure of the ISC matrices in Figure S6 again indicates that the across-language ISC is much lower than the within-language ISC. Interestingly, however, we also find that the signals from the auditory region showed some correlation across languages (e.g., r = 0.12 between French and Chinese split halves). Although these correlations are lower than those we find for the precuneus, they are surprising because these regions were supposed to process very different auditory inputs. To zoom in on this region, we reran the RMSE-correlation analyses (see Methods section), but this time also across datasets that were not paired in the original study (i.e., French listeners’ auditory cortices were never exposed to the audio-signal from the Chinese- or Englishlanguage audiobook). The results of this analysis stream (details in Supplementary Figure S5) confirm that each language-specific RMSE signal always correlated most strongly with the fMRI signal from the corresponding language’s listeners. Moreover, results suggest that the correlation between auditory brain signals from different languages is not simply explained by residual correlations between the auditory input stimulus (e.g., one could have expected that a story told in French, Chinese, or English could contain pauses at similar time points, thereby triggering some ISC in auditory regions due to low-level stimulus similarities). Thus, a potential explanation for this result pattern could be a top-down attention effect.
In the realm of neuroscience studies examining how our brains process information, particularly during naturalistic tasks like listening to audiobooks, several methods exist. Inter- Subject Correlation (ISC) analysis examines how similar brain responses are across different people when exposed to the same stimulus. For example, when people listen to the same story, ISC analysis identifies how similar the brain activity patterns are in a specific brain region across these individuals. ISC is thus particularly useful for identifying which parts of the brain are consistently engaged in processing the stimulus in a similar way across people. Therefore, ISC provides insights into the shared neural processes that occur during common experiences, making the method theoretically relevant for communication and audience research. This is why the main analysis in this manuscript relies on the ISC approach.
Inter-Subject Functional Connectivity (ISFC) analysis, on the other hand, was conceived as an extension of ISC analysis, connecting the ‘acrossbrain’ ISC approach to the burgeoning area within neuroscience that focuses on intra-brain dynamics (a.k.a. functional connectivity Friston, 2011; Simony et al., 2016). Instead of just looking at how similar the brain activity is in a specific region across different people, ISFC examines how the brain activity time series in a given region from one person relates to the brain activity in many other brain regions of other recipients. Essentially, ISFC looks at how the relationships between different brain regions, and it has the advantage that while intra-brain functional connectivity measures are often contaminated by naturalistic stimulus dynamics and instead investigated in the resting state, ISFC analysis might, at least in part, be able to disentangle stimulus-driven brain dynamics (e.g., due to the story sound’s acoustic properties) from internally driven brain modulations (e.g., due to resting-state fluctuations).
The main analyses reported in the paper focus on ISC methodology because it is most apt to demonstrate cross-audience alignment, whereas this aspect is not as relevant for ISFC. However, because the brain undeniably operates on a networked basis, and ISFC is the most suitable method to study brain network dynamics during natural stimulation, we complemented the ISC analysis with a supplementary ISFC analysis (and, to compare ISFC results, we also carried out a conventional function connectivity analysis).
To this end, we computed an ISFC analysis for each language group. Specifically, we used the ISFC implementation in the nltools package (Chang et al., 2022). The nltools IFSC implementation uses the leave-one-out approach to compute, for each subject, the cross-correlation between each brain region and the average of the rest of the subjects’ data. Thus, for each language group, we first loaded all listeners’ data (e.g., 33 matrices of n_regions (293) by n_TR (varies across runs/sections, ca. 300) and combined and reordered these appropriately for the ISFC function. We then submitted the resulting matrix/ list to ISFC analysis as well as conventional intra-brain functional connectivity analysis (i.e., correlating each regional brain activity vector from a given person against all other regions from this subject). We averaged the results and plotted them as ISFC or connectivity matrices, respectively. Importantly, this approach was repeated for each language group.
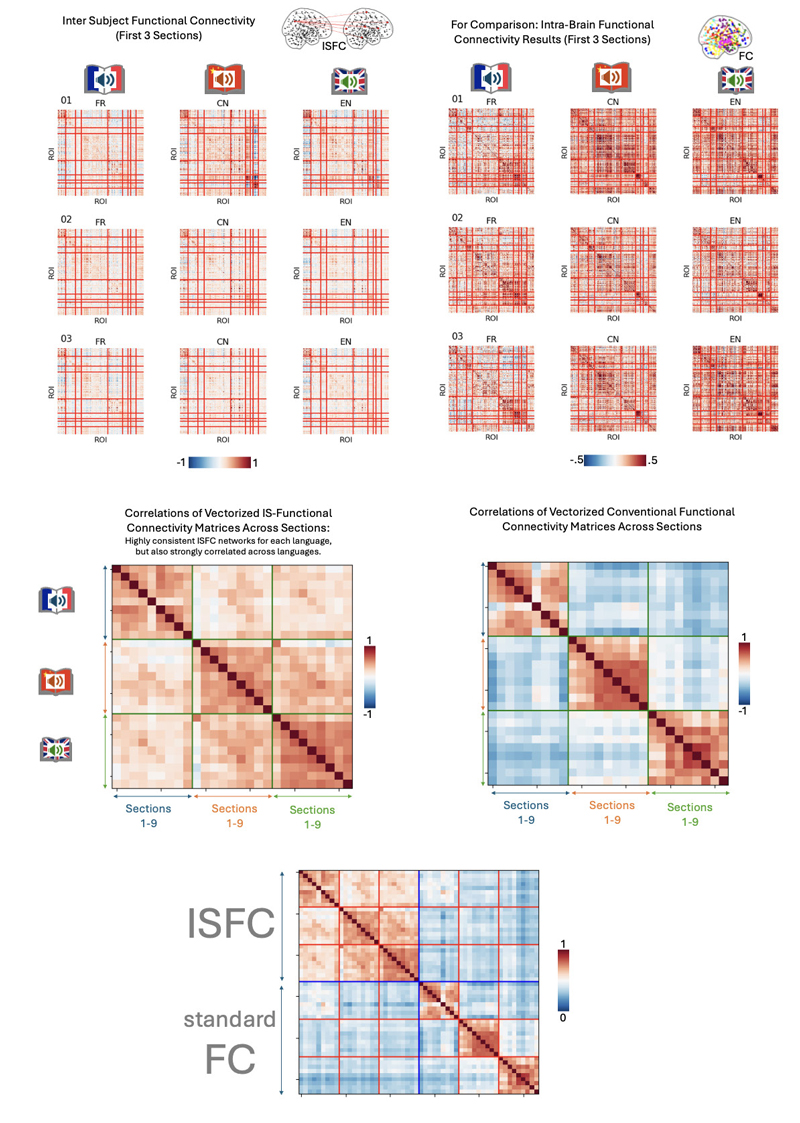
Theoretical rationale for data analysisNote. Top left: ISFC results for first three sections. Red lines indicate distinct network as defined by the Shen et al. (2013) labels for canonical brain networks. Shown are results for the first three subsections of the audiobook, and the remaining results images are provided in the online repository: https://github.com/nomcomm/littleprince_multilingual. Top right. Corresponding results using conventional intra-brain connectivity analyses (i.e. time-series correlations), which reflect the influence of both intrinsic as well as extrinsic brain dynamics. Middle left. The ISFC matrices from all sections as well as the different language versions are vectorized and compared. As can be seen, this reveals common connectivity structures across the different sections (internal consistency), but also to some degree across languages (albeit lower than within each language). Middle right: Same analysis for conventional intra-brain connectivity does not reveal this effect because intra-brain connectivity results are less apt to isolate the extrinsic (i.e. story-induced). Bottom panel: Combining the results from the ISFC and FC analyses shows that ISFC analyses reveal some cross-brain convergence, not seen for the FC results.
SUPPLEMENTARY REFERENCES


