
The Not-So-Obvious Harm of Cyberhate: Source Magnification of Hate Tweets, Unhealthy Food Choice, and the Moderating Role of Group Identification
Copyright ⓒ 2021 by the Korean Society for Journalism and Communication Studies
Abstract
Guided by research demonstrating the impact of social identity threat on physical health and the source magnification framework, we investigated how hate tweets from multiple sources, relative to hate tweets from a single source, influence target group members’ health-related behavior—specifically, food choice. We conducted an online experiment with a sample of Asian Twitter users in the United States. Participants were randomly assigned to view an identical set of anti-Asian hate tweets either from a single individual or from multiple individuals. Subsequently, in an ostensibly unrelated task masked as a marketing survey, participants customized a pizza by selecting toppings from a list presenting an equal number of healthy and unhealthy toppings. We found that participants exposed to multiple-source hate tweets selected a greater number of unhealthy toppings than those exposed to single-source hate tweets. We also found that group identification was a significant moderator, with those lower in group identification more likely to choose unhealthy toppings when exposed to multiple-source hate tweets. Our findings suggest that exposure to multiple-source hate messages, relative to single-source hate messages, may exert a detrimental influence on target group members’ health to a greater extent, while highlighting a possible buffering role of group identification.
Keywords:
cyberhate, hate tweet, multiple source effect, food choice, group identificationWhile relatively less visible in face-to-face interaction contexts, explicit hate pervades online environments (Douglas et al., 2005; Tsesis, 2001). The Internet has long served as a visible and influential platform for mobilizing and propagating hate (Douglas et al., 2005; Leets, 2001), and, more recently, cyberhate messages are frequently and widely disseminated through popular social platforms. In particular, Twitter—a social media platform for microblogging and online social networking (Vergeer & Hermans, 2013)—has received attention for creation and dissemination of hate messages (Bartlett et al., 2014; Chaudhry, 2015). Twitter users often tap into the Twitter platform as a catalyst for social good, such as mobilization of collective action (Louis et al., 2020) and exchange of social support to enhance well-being (Kim & Eltantawy, 2016). However, the relative brevity of Twitter-based communication has been noted as a possible driver of uncivil behaviors including hate speech (Jaidka et al., 2019).
One of the key technological affordances of social media is that multiple sources and their messages can be presented in an aggregated form, enabling many-to-many communication (Anstead & O’Loughlin, 2011; Marwick & boyd, 2011) and making social platforms “an ideal instrument for the wide spreading of hate speech by extremists and hate mongers” (Iglezakis, 2017, p. 368). Inspired by the multiple source effect framework (Harkins & Petty, 1981, 1987) and the concept of source magnification (Harkins & Petty, 1981; Moore et al., 1994; Moore & Reardon, 1987), we investigated whether hate tweets from multiple sources, relative to those from a single source, inflict greater harm on target group members. Specifically, guided by research on the influence of social identity threat and discrimination experience on health-impairing behaviors (e.g., Pascoe & Richman, 2011), we investigated the effect of multiple-source hate tweets on unhealthy food choice. In so doing, we also examined the possible moderating role of group identification.
Social Identity Threat and Unhealthy Food Choice
Social identity threat, which refers to situations in which people feel concerned about being stigmatized, devalued, or marginalized because of their group memberships (Adams et al., 2006; Inzlicht & Kang, 2010), is an acute social stressor that can exert a detrimental influence not only on target group members’ psychological well-being but also on their physical well-being (Pascoe & Richman, 2009). Research has shown that perceived group-based discrimination can increase target group members’ engagement in health-impairing behaviors such as smoking (Landrine & Klonoff, 1996), problem drinking (Kwate et al., 2010), and substance use (Gibbons et al., 2010; Kam et al., 2014) by reducing mental energy and resources for self-control (Pascoe & Richman, 2009).
Another behavioral outcome that merits attention is food-related decisions, specifically, unhealthy food choice. The relationship between social identity threat and unhealthy food choice, which may not be intuitively obvious, can be explained by how the experience of social identity threat affects self-control processes. Specifically, when experiencing social identity threat, people’s attempt to cope with the threat can deplete self-regulatory resources, thereby interfering with psychological processes related to self-control (Major et al., 2014). As people engage in healthy eating when they can “overcome temptations to eat high-calorie, high-fat food, both of which require self-regulatory resources to be successful” (Inzlicht & Kang, 2010, p. 472), those who experience social identity threat may find it difficult to exercise self-control when they are presented with high-calorie, high-fat food items.
Several studies have yielded empirical support for the relationship. One study, for example, demonstrated that Black American students, after recalling their own experiences of discrimination, were more likely to choose unhealthy food options (e.g., fried chicken sandwich) than healthy food options (e.g., grilled chicken sandwich) in a food choice task (Pascoe & Richman, 2011, Study 1). Also, female students who received negative feedback from a reviewer ostensibly exhibiting sexist attitudes, compared to those who received negative feedback from a seemingly fair reviewer, were more likely to make less healthy food-related decisions (e.g., choosing a candy bar over an “all-natural” granola bar) when asked to select a treat (Pascoe & Richman, 2011, Study 2).
In a similar vein, Inzlicht and Kang (2010) investigated the effect of social identity threat associated with negative gender-related stereotype on eating behavior. In their study, female participants were instructed to take a math test labeled as a test of math intelligence, which was intended to heighten the salience of a negative stereotype associated with their female identity (i.e., “females are not good at math”). One group was given an opportunity to reappraise and cope with the threat; the other group was not given an opportunity to cope with the threat through reappraisal. After taking the math test, participants were offered high-calorie, high-fat food (i.e., ice cream) in a task disguised as food tasting task. The results showed that the females who were challenged with social identity threat but were not armed with a coping strategy, compared with those who were challenged but were armed with a coping strategy, consumed a greater amount of ice cream after the math test, and this pattern was more pronounced among those with higher levels of gender stigma consciousness (Inzlicht & Kang, 2010).
Taken together, the extant research suggests that threat to one’s social identity significantly increases the likelihood for target group members to engage in unhealthy eating. Particularly noteworthy of this phenomenon is that the effects of social identity threat on tasks that require self-regulatory resources often “occur in the absence of self-reported threat or anxiety, suggesting that they are outside of conscious awareness” (Major et al., 2014, p. 75). Racist speech, which refers to utterances that derogate and stigmatize certain groups of people based on their racial/ethnic backgrounds (Sedler, 1991), “remains one of the most pervasive channels through which discriminatory attitudes are imparted” (Delgado, 1982, p. 135); therefore, exposure to such messages can trigger social identity threat to members of the targeted group (Leets & Giles, 1999). Guided by these ideas, we investigated the effect of social identity threat on unhealthy food choice in a context that has yet to be studied: possible magnification of the harmful effect of cyberhate in a many-to-many communication context involving social platforms such as Twitter.
Application of the Source Magnification Framework to Hate Tweets
Twitter has attracted attention as a social media environment that instigates expressions of negative sentiment against various social groups (Bartlett et al., 2014; Chaudhry, 2015; Fox et al., 2015; Lee, 2021). Twitter is particularly important to note in terms of its capacity to disseminate messages from multiple sources that share a particular idea or theme in an aggregated form through the hashtag feature (Fox et al., 2015). Given this technological context, a question worth addressing is whether and how the involvement of multiple sources (as opposed to a single source) in dissemination of hate messages could magnify their harmful effects on target individuals. Initial empirical support for this idea has been provided by Lee-Won et al. (2020), who built their argument upon the multiple source effect and source magnification literature (Harkins & Petty, 1981, 1987; Moore et al., 1994; Moore & Reardon, 1987).
Originally developed as a model of persuasion, the multiple source effect framework has adopted an information processing approach to explain attitude change. Specifically, the multiple source effect framework postulates that persuasion depends not only on the characteristics of messages but also on the number of sources that deliver the messages, as involvement of multiple sources (as opposed to a single source) in message delivery increases message recipients’ diligence in cognitive processing of the messages. Consequently, messages from multiple sources, compared to identical messages from a single source, induce greater message elaboration and can “lead to either enhanced or reduced persuasion, depending on the quality and cogency of the arguments” (Harkins & Petty, 1981, p. 411). For example, participants exposed to three high-quality arguments delivered by three different sources found the messages more persuasive than those exposed to the same arguments delivered by a single source; by contrast, participants exposed to three low-quality arguments delivered by three different sources were less persuaded than those exposed to the same arguments delivered by a single source. These results provided empirical support for the occurrence of source magnification.
The multiple source effect research focused on information processing in persuasion. Extending the concept of source magnification to hate messages, Lee-Won et al. (2020) investigated whether a similar form of information processing could occur when target group members are exposed to hate messages from multiple sources. Noting the important role played by source independence in the persuasive advantage of multiple-source messages (Harkins & Petty, 1987; Moore et al., 1994), Lee-Won and her colleagues postulated that multiple independent sources could magnify the effects of hate messages on target individuals “because such messages are (1) less likely to be disregarded and (2) more likely to be processed with renewed attention” compared to messages from a single source (Lee-Won et al., 2020, p. 607). Their online experiment supported the prediction, demonstrating that multi-source hate tweets against Blacks aggregated through a hashtag, relative to single-source hate tweets of identical content, led Black participants to report greater emotional distress and, in turn, greater attribution to prejudice. Building upon the work by Lee-Won et al. (2020), we predict that hate tweets coming from multiple independent sources that are aggregated via hashtagging may be more difficult to dismiss as an isolated or random incident than hate tweets from a single source, as each message coming from a distinct source may be processed with renewed attention and increased thinking. Such multiple-source hate tweets may also signal that the hate directed against one’s ingroup is collective and pervasive (Lee-Won et al., 2020).
The multiple-source effect of hate messages on health-related behavior such as unhealthy eating has yet to be demonstrated. However, if multiple-source hate tweets magnify target group members’ perception of threat to their group identity, it is likely that coping with social identity threat from multiple-source hate tweets may consume even greater self-regulatory resources, relative to coping with the threat from single-source hate tweets. Considering this possibility, we predict that multiple-source hate messages would lead to greater likelihood of unhealthy food choice among target group individuals. Along this line of reasoning, we hypothesized:
- H1: Target group members exposed to multiple-source hate messages will more likely choose unhealthy food items than will those exposed to single-source hate messages.
The Role of Group Identification
Individuals vary in the degree to which they identify themselves with a group. This individual difference in group identification—the extent to which a social group or category membership is included into one’s self-concept and fosters sense of belonging (Hansen & Sassenberg, 2006; Tynes et al., 2008)—is a key determinant of how people react to threats to their social identity (McCoy & Major, 2003). Members strongly identified with a marginalized or stigmatized group are less likely to distance themselves from the group and more likely to be concerned with the status of the group when compared with weak identifiers (Ellemers et al., 2002). At the same time, strong group identification can serve as a source of psychological resources for group members to cope with challenging situations (Branscombe et al., 1999). Consequently, group identification can play a moderating role in how social identity threat affects target individuals’ wellness (Pascoe & Richman, 2009).
On the one hand, group identification has been found to play an exacerbating role in the harmful effect of social identity threat on target group members, largely because those who are strongly identified with their ingroup, compared to weak identifiers, are more likely to be attentive to the cues signaling the threat associated with their membership (Sellers & Shelton, 2003). For example, upon receiving a negative evaluation ostensibly due to sexist biases, female college students low in gender identification reported less negative emotion when compared to their counterparts high in gender identification (McCoy & Major, 2003). Similar patterns were found for health-related consequences. For example, Asian Americans who were strongly identified with their ethnic group suffered the impact of frequent discrimination experience on depressive symptoms to a greater extent when compared with weak identifiers (Lee, 2005). Also, identification with one’s ethnic group was found to aggravate the negative influence of acculturative stress on psychological well-being among Mexican immigrants (Kim et al., 2014). Based on these empirical findings, we hypothesized:
- H2a: The effect of multiple-source (vs. single-source) hate tweets on unhealthy food choice will be greater for those with higher group identification than for those with lower group identification.
On the other hand, strong identification with one’s group has been found to buffer the stress from threat experiences and reduce their deleterious impact on mental and physical health. In the context of media exposure, when exposed to rap music videos portraying thin ideal images, African American females with strong ethnic identity were less likely to exhibit bulimia action tendencies while those with weak ethnic identity were more vulnerable to the harmful influence of thin-ideal rap music videos (Zhang et al., 2009). Also, a study conducted with Latino Americans revealed that ethnic identification was a positive predictor of both mental and physical health (Ai et al., 2014), suggesting that group identification may protect the group members against stressors associated with identity-related threat. Research conducted with college women also showed that a strong sense of positive, action-oriented feminist identity attenuated the impact of sexist events on disordered eating (Sabik & Tylka, 2006). Also noteworthy is that group identification not only showed a significant and positive association with personal control but also protected individuals against a loss of personal control (Greenaway et al., 2015). Given these findings, group identification may reduce the potentially detrimental effect of exposure to multiple-source hate tweets on unhealthy food choice. Therefore, we proposed the following competing hypothesis:
- H2b: The effect of multiple-source (vs. single-source) hate tweets on unhealthy food choice will be greater for those with lower group identification than for those with higher group identification.
METHOD
We conducted an online experiment to test the proposed hypotheses. Prior to data collection, approval was obtained from the institutional review board (IRB) of the first authors’ institution. We focused on Asians living in the United States as the target racial group in this experiment, considering that Asians are often targeted by racist jokes and negative stereotyping on Twitter (Nakagawa, 2013).
Participants and Design
A total of 143 Asian Twitter users living in the United States (67 females, 46.9%) were recruited through the online panel service managed by Qualtrics. Participants’ age ranged from 18 to 47 (M = 30.84, SD = 7.08). The experiment was based on two between-participants conditions: single-source hate tweets vs. multiple-source hate tweets.
Materials
We conducted a pretest of anti-Asian tweets with 56 college students attending a large Midwestern university as judges. The judges rated a total of 31 anti-Asian tweets, collected from keyword searches, on a 7-point scale (1 = not at all hateful to 7 = very hateful). Eight tweets that ranked highest were selected (e.g., “You are Asian, so I hate you”; “The Asian restaurant on my block is officially closed. Haven’t seen this many Asians clear out since Hiroshima”; “I just got hushed at the library by an Asian. I’m going to tell her go back to her own country if she wants to study!”). The mean hatefulness ratings of the selected tweets ranged from 6.43 (SD = 0.93) to 6.73 (SD = 0.95).
The multiple-source tweets condition presented the anti-Asian tweets as a hashtag feed containing eight messages with the hashtag #IHateAsians written by eight different Twitter users. The single-source tweets condition presented the same set of anti-Asian tweets shown in the multiple-source condition on a single Twitter user’s timeline (see Appendix).
As with the research conducted on the source magnification effects of anti-Black hate tweets (Lee-Won et al., 2020), we followed the stimuli creation approach used in the original multiple source effect framework designed to compare messages from a single source and those from multiple sources while controlling for possible effects of the individual source cues (Harkins & Petty, 1987; Lee & Nass, 2004; Moore et al., 1994). Specifically, we used all of the eight sources presented in the multiple-source conditions as the Twitter profile holder in the single-source condition stimuli, and one of these eight sources was randomly presented to participants within the single-source condition. All of the eight usernames used as the sources of the anti-Asian tweets were European American names used for both males and females (e.g., Glenn, Jordan, Sam). Also, we sought to minimize unwanted influence of the hashtag feature per se; therefore, the same hashtag (#IHateAsians), which was essential to creating the multiple-source condition, accompanied all of the tweets in the single-source condition as well.
Procedure
In order for us to properly assess the effect of hate tweets on food choice while minimizing demand characteristics or social desirability bias, it was important to disguise the true intent of the experiment. Therefore, the cover story stated that participants would be asked to partake in a series of unrelated short studies investigating people’s social media use and lifestyle. After providing online consent, participants were randomly assigned to either the single-source (n = 72) or the multiple-source (n = 71) condition. In both conditions, participants were first presented with general information about Twitter and then were presented with the hate tweets. The next task was presented as a marketing survey, which asked participants to customize a pizza by choosing toppings they wanted to have if they were to order a pizza from a local pizza restaurant right at the moment. Following this task, participants responded to a questionnaire containing measurement and manipulation check items. Upon completion, participants were fully debriefed on the deception method used in the experiment (i.e., information on the true intent of the study withheld from the participants). Then, participants were given instructions about how to contact the research team in case they wanted to withdraw their data after learning about the incomplete disclosure and deception involved in the study. None of the participants contacted the research team to request withdrawal of their data.
Measures
Overview. Unhealthy food choice was operationalized as participants’ selection of food items that are high in saturated fat and calories. We employed a context of food consumption that is pretty common to most people in the United States and is also relatively less sensitive to dietary restrictions and food preferences: pizza customization (Godek, 2002; Nagpal et al., 2015). A total of 14 items generally offered as toppings in U.S. pizza restaurants were selected. Half of them were relatively unhealthy (i.e., high in calories and fat): bacon, beef, Canadian bacon, cheese, ham, pepperoni, and sausage. The rest were items relatively low in saturated fat and calories: basil, black olive, green pepper, mushroom, onion, pineapple, and tomato.
Pretest of the Perceived Healthiness of the Food Items. We recruited 82 individuals on Amazon.com’s MTurk as judges for pretesting the items used in the food choice task. On a 6-point scale, each of the 14 food items were rated in terms of perceived healthiness (1 = unhealthy to 6 = healthy). Among the seven “healthy” topping items, tomato received the highest rating, M = 5.65 (SD = 0.50), and black olive received the lowest rating, M = 4.79 (SD = 0.92); all of the seven unhealthy topping items received generally low healthiness ratings, with bacon rated lowest, M = 2.07 (SD = 0.99) and beef rated highest, M = 3.56 (SD = 1.05). We set the mean value of black olive (4.79), which had the lowest healthiness rating among the seven healthy toppings, as a predetermined benchmark score. Against this mean value, we conducted one-sample t-tests to assess whether the seven “unhealthy” topping items were perceived as significantly more unhealthy. The results confirmed that all of the seven “unhealthy” toppings were rated significantly lower than black olive on healthiness, with t values ranging from -24.82 (bacon) to -10.54 (beef) and all ps < .001.
Instructions and Scoring. Participants were instructed to select seven toppings out of the 14 items, and the number of high-fat, high-calorie items included in their selection represented unhealthy food choice, with the scores ranging from 0 to 7.
The Multigroup Ethnic Identity Measure (MEIM), first developed by (Phinney, 1992), has served as an important measure of ethnic identity/identification for use with people from diverse racial/ethnic groups, including Asians/Asian Americans (Lee & Yoo, 2004; Yoo & Lee, 2005). Participants rated their agreement to the 12 items from the Revised Multigroup Ethnic Identity Measure (MEIM) scale. The scale items included “I have a strong sense of belonging to my own ethnic group,” “I have a clear sense of my ethnic background and what it means for me,” “I am happy that I am a member of the group I belong to,” “I have a lot of pride in my ethnic group,” and “I feel a strong attachment towards my own ethnic group” (Roberts et al., 1999). The items were rated on a 7-point scale (1 = strongly disagree to 7 = strongly agree) and were averaged (α = .91).
To minimize the influence of possible confounding variables, we controlled for the following four variables: vegetarianism, personal health consciousness, discrimination experience, and amount of Twitter use.
Vegetarianism. Given that most of the unhealthy toppings were meat-based and participants who consciously avoid meat-eating might not have selected those items, we assessed vegetarianism (i.e., the practice of abstaining from meat consumption) by asking participants whether they were vegetarian (1) or not (0).
Personal Health Consciousness. People who are high in personal health consciousness tend to reflect upon their physical health and fitness (Snell Jr. et al., 1991) and may be inclined to avoid eating unhealthy food items. Considering this, we controlled for personal health consciousness, which was assessed with three items (e.g., “I am generally attentive to how I feel about my physical health”) adapted from Kaskutas and Greenfield (1997); the items were rated on a 7-point scale (1 = strongly disagree to 7 = strongly agree; α = .88) and were averaged.
Past Experience of Discrimination. We controlled for self-reported past discrimination experience considering that such experience can influence both perception of and response to identity-threatening incidents (Richman et al., 2007). Following Pascoe and Richman (2011), we assessed past discrimination experience with the 10-item version of the Everyday Discrimination Scale (e.g., “You are treated with less courtesy than others,” “People ignore you or act as if you are not there”); the items were rated on a 6-point scale (1 = never to 6 = almost every day; α = .96) and were averaged.
Amount of Daily Twitter Use. As prior experience with a social platform (Twitter) could influence how one perceives and responds to hate messages distributed through the same platform, we controlled for daily Twitter usage (in minutes) by asking how much time participants spent on Twitter on an average day; the distribution was highly skewed (M = 81.33, SD = 138.84), so the variable was log-transformed.
RESULTS
Manipulation Check
At the end of the experiment, we checked whether participants correctly identified the source type of the tweets (single vs. multiple) by condition. A chi-square test confirmed that there was no significant difference in the correct vs. incorrect response frequency by condition, χ2(1, N = 143) = 2.62, p = .105. Those who provided an incorrect response (n = 7 for the multiple-source condition and n = 14 for the single-source condition) were excluded. As a result, a total of 122 valid cases (n = 64 for the multiple-source condition and n = 58 for the single-source condition) were included in subsequent analyses.1 Table 1 provides the zero-order correlation matrix of the measured variables, along with their means and standard deviations.
Hypothesis Testing
H1 was tested with analysis of covariance (ANCOVA) controlling for the four covariates and our proposed moderator, group identification (Table 2). The ANCOVA revealed a significant effect of source type, F(1, 115) = 4.60, p = .034, ηp2 = .04. Specifically, participants in the multiple-source condition chose more unhealthy toppings (Madj = 3.69, SE = 0.19) than those in the single-source condition (Madj = 3.10, SE = 0.20). Thus, H1 was supported.
The competing hypotheses on the role of group identification (H2a and H2b) were tested with ordinary least squares (OLS) regression based on Model 1 of the PROCESS macro for SPSS (Hayes, 2021) with the four covariates included (Table 3). The analysis yielded a significant interaction effect of source type and group identification, b = -0.54, SE = 0.27, t = -1.99, p = .048.

Ordinary Least Squares (OLS) Regression Examining the Moderating Role of Group Identification in the Effect of Hate Tweet Source Type on Number of Unhealthy Toppings
As Figure 1 shows, the exposure to the multiple-source hate tweets was more likely to increase participants’ choice of unhealthy toppings particularly among those relatively low in group identification. As group identification was a continuous moderator, we followed the recommendation of Hayes (2018) and used the Johnson-Neyman technique in further probing the interaction. Specifically, the Johnson-Neyman technique allowed us to determine the complete range of group identification values in which the multiple-source hate tweets significantly affected unhealthy topping choice. The region of significance confirmed that the effect of multiple-source hate tweets on the number of unhealthy toppings was significant when the group identification values were equal to or lower than 4.86 (Figure 2). Thus, the data supported H2a.2
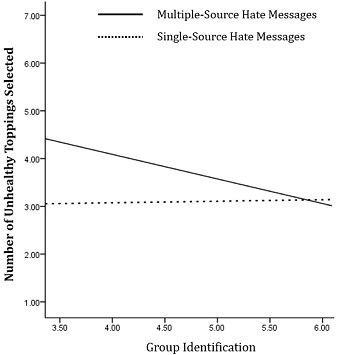
The Moderating Role of Group Identification in the Effect of Hate Tweet Source Type on Unhealthy Topping Choice
DISCUSSION
Theoretical and Practical Implications
The present study demonstrated that the multiple source effect, which has mostly been studied in the context of persuasion, may apply to hate messages disseminated through social media platforms such as Twitter. Our findings showed that anti-Asian hate tweets from multiple sources, relative to anti-Asian tweets of identical content from a single source, led participants to choose a greater number of relatively unhealthy (i.e., high in saturated fat and calories) toppings in a pizza customization task, which was disguised as a marketing survey question.
Building on the research on the detrimental effects of hate tweets on target group members (Lee-Won et al., 2017; Lee-Won et al., 2020) and on the impact of social identity threat on target group members’ engagement in health-impairing behavior (Gibbons et al., 2010; Kam et al., 2014; Kwate et al., 2010; Pascoe & Richman, 2011), our findings indicate that exposure to hate tweets from multiple sources may increase the likelihood for target group members to choose unhealthy food items. This confirms that cyberhate manifested through the many-to-many mode of message distribution via social media platforms such as Twitter (Anstead & O’Loughlin, 2011) may potentially magnify the psychological harm to target group members.
One unique contribution of our work is that we have extended the findings of Lee-Won et al. (2020) on source magnification of cyberhate. Whereas Lee-Won et al. (2020) focused on the possible interpersonal and relational harm of multiple-source hate tweets as manifested in attribution to prejudice, the present findings illustrate that source-magnified cyberhate can exert a harmful impact on target group members’ physical health by depleting the mental energy and resources for self-control.
Our work also contributes to the literature on the role of group identification in responses to social identity threat. Past research has yielded conflicting findings as to whether group identification ameliorates (Ai et al., 2014; Outten & Schmitt, 2015) or exacerbates (Lee, 2005; Noh et al., 1999) the deleterious impact of social identity threat on those who experience the threat. In our study, group identification was found to significantly reduce the multiple source effect of hate tweets on Asian targets’ unhealthy food choice, indicating that strong identifiers may be less vulnerable to source magnification of hate messages compared to weak identifiers. Extending past research elucidating the role of group identification as a source of resilience (Chan & Mak, 2021), our finding suggests that a sense of connection to one’s racial/ethnic group might have protected target group members (Pascoe & Richman, 2009) from the impact of derogative messages magnified by multiple sources. Specifically, given that the multiple source effect of hate tweets on unhealthy food choice was likely driven by a state of self-control depletion heightened by multiple sources, our findings confirm that group identification can shield individuals against a potential loss of self-control (Greenaway et al., 2015), even in identity-threatening contexts involving hate speech.
This study also has noteworthy practical implications. Unlike hate messages generated by online hate groups mobilized through the Internet (Leets, 2001), multiple-source hate tweets presented in an aggregated form might be perceived as causing little harm to target group members because of their seemingly casual and dispersed nature. Our findings, however, call attention to the harm of source-magnified hate tweets that can broadly impact target group members’ health, which resonate with recent findings on the pernicious effects of online aggressions—such as cyberbullying—on victims’ well-being (Peleg-Oren et al., 2012; Selkie et al., 2015). On a more positive note, the evidence for the protective role of group identification points to how members of the socially and culturally marginalized groups could potentially guard themselves against the harmful effect of multiple-source hate messages through forming and maintaining stronger social, cultural, and emotional ties with their group (Pascoe & Richman, 2009). This understanding may inform interventions designed for target group members of cyberhate. One’s group identification can be fostered through solidarity with others sharing the group membership (Gaertner et al., 1993; Yim & Jeong, 2020). Offering online communities for Asians/Asian Americans on social platforms to celebrate their racial/ethnic identity and to promote group solidarity may help Asian minorities cope with detrimental impact of hate messages disseminated via social platforms, particularly in the form of many-to-many communication.
Limitations and Future Directions
The present research is limited in several aspects. First, the current findings provided initial support for source magnification of hate messages and their effect on unhealthy food choice, and a possible mechanism underlying the effect is depletion of self-regulatory resources. However, the design of our study fell short of empirically demonstrating specific mechanisms, which may be considered a major limitation. It has been noted that behavioral responses to social exclusion or social identity threat involving depletion of self-regulatory resources—such as unhealthy food choice—may occur in the absence of, or independently of, self-reported negative affect (Baumeister et al., 1998; Pascoe & Richman, 2011) or perceived threat to one’s social identity (Major et al., 2014). In order to elucidate specific causal pathways, future research should consider employing non-self-report measures to properly capture underlying mechanisms gauging the use of self-regulatory resources. A possible step in this direction is to use physiological measures such as skin conductance level and heart rate to detect emotional distress (Iffland et al., 2014) or perceived social identity threat (Murphy et al., 2007).
Second, the target sample of this study was limited to Asians living in the United States; therefore, we are unable to determine whether the findings reported here would generalize to other social-cultural groups that are frequently targeted by cyberhate. Given this, it will be important to replicate the current findings with members of other social-cultural groups. Relatedly, taking a comparative approach across different social-cultural groups and understanding the patterns of similarities and differences in their responses to multiple-source hate messages will be fruitful.
Another limitation concerns our measurement of unhealthy food choice, which was based on a pizza customization task. We used this measure considering that the task would be relatively less sensitive to possible dietary restrictions and food preferences participants might have. Also, this approach allowed us to measure food choice that could closely align with eating behavior even in a non-laboratory setting. However, it should be noted that the task may not be optimal for replication of the present study in cultures where pizza consumption and customization may not be as common as in the United States.
In addition, this study primarily focused on the short-term effect of multiple-source hate tweets on unhealthy food choice. More work is needed to provide insight into possible long-term effects of multiple-source hate messages disseminated through social platforms on health-impairing behaviors.
Finally, although the present findings suggest that group identification—specifically, racial/ethnic identification—may buffer target group members against social identity threat associated with hate messages, the mixed findings of past research point to the complexities of the role played by group identification (Pascoe & Richman, 2009). Future research should continue to examine the role of group identification in target group members’ response to collective cyberhate manifested in social media environments, beyond the context examined in the present study.
CONCLUSION
Demonstrating the significant effect of multiple-source hate tweets on target group members’ unhealthy food choice, the present research provides initial evidence for the potential negative impact of many-to-many hate speech disseminated through Twitter on target group members’ health. Continued research efforts should be devoted to understanding the impact of source-magnified hate messages generated and disseminated in the many-to-many mode on popular social media platforms.
Notes
References
-
Adams, G., Garcia, D. M., Purdie-Vaughns, V., & Steele, C. M. (2006). The detrimental effects of a suggestion of sexism in an instruction situation. Journal of Experimental Social Psychology, 42(5), 602–615.
[https://doi.org/10.1016/j.jesp.2005.10.004]
-
Ai, A. L., Aisenberg, E., Weiss, S. I., & Salazar, D. (2014). Racial/ethnic identity and subjective physical and mental health of Latino Americans: An asset within? American Journal of Community Psychology, 53(1-2), 173–184.
[https://doi.org/10.1007/s10464-014-9635-5]
-
Anstead, N., & O’Loughlin, B. (2011). The emerging viewertariat and BBC Question Time: Television debate and real-time commenting online. The International Journal of Press/Politics, 16(4), 440–462.
[https://doi.org/10.1177/1940161211415519]
- Bartlett, J., Reffin, J., Rumball, N., & Williamson, S. (2014). Anti-social media. Demos. http://www.demos.co.uk/files/DEMOS_Anti-social_Media.pdf?1391774638
-
Baumeister, R. F., Bratslavsky, E., Muraven, M., & Tice, D. M. (1998). Ego depletion: Is the active self a limited resource? Journal of Personality and Social Psychology, 74(5), 1252–1265.
[https://doi.org/10.1037/0022-3514.74.5.1252]
-
Branscombe, N. R., Schmitt, M. T., & Harvey, R. D. (1999). Perceiving pervasive discrimination among African Americans: Implications for group identification and well-being. Journal of Personality and Social Psychology, 77(1), 135–149.
[https://doi.org/10.1037/0022-3514.77.1.135]
-
Chan, R. C. H., & Mak, W. W. S. (2021). Protective and compensatory effects of group identification on the mental health of people living with HIV. Archives of Sexual Behavior, 50(4), 1677–1687.
[https://doi.org/10.1007/s10508-020-01823-7]
-
Chaudhry, I. (2015). #Hashtagging hate: Using Twitter to track racism online. First Monday, 20(2).
[https://doi.org/10.5210/fm.v20i2.5450]
- Delgado, R. (1982). Words that wound: A tort action for racial insults, epithets, and name-calling. Harvard Civil Rights-Civil Liberties Law Review, 17(1), 133–181.
-
Douglas, K. M., Mcgarty, C., Bliuc, A.-M., & Lala, G. (2005). Understanding cyberhate: Social competition and social creativity in online white supremacist groups. Social Science Computer Review, 23(1), 68–76.
[https://doi.org/10.1177/0894439304271538]
-
Ellemers, N., Spears, R., & Doosje, B. (2002). Self and social identity. Annual Review of Psychology, 53, 161–186.
[https://doi.org/10.1146/annurev.psych.53.100901.135228]
-
Fox, J., Cruz, C., & Lee, J. Y. (2015). Perpetuating online sex i sm off line: Anonymity, interactivity, and the effects of sexist hashtags on social media. Computers in Human Behavior, 52, 436–442.
[https://doi.org/10.1016/j.chb.2015.06.024]
-
Gaertner, S. L., Dovidio, J. F., Anastasio, P. A., Bachman, B. A., & Rust, M. C. (1993). The common ingroup identity model: Recategorization and the reduction of intergroup bias. European Review of Social Psychology, 4(1), 1–26.
[https://doi.org/10.1080/14792779343000004]
-
Gibbons, F. X., Etcheverry, P. E., Stock, M. L., Gerrard, M., Weng, C.-Y., Kiviniemi, M., & O'Hara, R. E. (2010). Exploring the link between racial discrimination and substance use: What mediates? What buffers? Journal of Personality and Social Psychology, 99(5), 785–801.
[https://doi.org/10.1037/a0019880]
- Godek, J. (2002). Special session summary personalization and customization: Implications for consumer decision making and behavior. In S. M. Broniarczyk & K. Nakamoto (Eds.), Advances in Consumer Research (Vol. 29, pp. 155–157). Association for Consumer Research.
-
Greenaway, K. H., Haslam, S. A., Cruwys, T., Branscombe, N. R., Ysseldyk, R., & Heldreth, C. (2015). From “we” to “me”: Group identification enhances perceived personal control with consequences for health and well-being. Journal of Personality and Social Psychology, 109(1), 53–74.
[https://doi.org/10.1037/pspi0000019]
-
Hansen, N., & Sassenberg, K. (2006). Does social identification harm or serve as a buffer? The impact of social identification on anger after experiencing social discrimination. Personality and Social Psychology Bulletin, 32(7), 983–996.
[https://doi.org/10.1177/0146167206287639]
-
Harkins, S. G., & Petty, R. E. (1981). Effects of source magnification of cognitive effort on attitudes: An information-processing view. Journal of Personality and Social Psychology, 40(3), 401–413.
[https://doi.org/10.1037/0022-3514.40.3.401]
-
Harkins, S. G., & Petty, R. E. (1987). Information utility and the multiple source effect. Journal of Personality and Social Psychology, 52(2), 260–268.
[https://doi.org/10.1037/0022-3514.52.2.260]
- Hayes, A. F. (2018). Introduction to mediation, moderation, and conditional process analysis: A regression-based approach (2nd ed.). The Guilford Press.
- Hayes, A. F. (2021). PROCESS for SPSS and SAS (Version 3.5.3). http://processmacro.org/download.html
-
Iffland, B., Sansen, L. M., Catani, C., & Neuner, F. (2014). Rapid heartbeat, but dry palms: Reactions of heart rate and skin conductance levels to social rejection. Frontiers in Psychology, 5, 956.
[https://doi.org/10.3389/fpsyg.2014.00956]
-
Iglezakis, I. (2017). The legal regulation of hate speech on the Internet. In T.-E. Synodinou, P. Jougleux, C. Markou, & T. Prastitou (Eds.), EU Internet Law: Regulation and Enforcement (pp. 367–383). Springer International Publishing.
[https://doi.org/10.1007/978-3-319-64955-9_15]
-
Inzlicht, M., & Kang, S. K. (2010). Stereotype threat spillover: How coping with threats to social identity affects aggression, eating, decision making, and attention. Journal of Personality and Social Psychology, 99(3), 467–481.
[https://doi.org/10.1037/a0018951]
-
Jaidka, K., Zhou, A., & Lelkes, Y. (2019). Brevity is the soul of Twitter: The constraint affordance and political discussion. Journal of Communication, 69(4), 345–372.
[https://doi.org/10.1093/joc/jqz023]
-
Kam, J. A., Castro, F. G., & Wang, N. (2014). Parent–child communication's attenuating effects on Mexican early adolescents' perceived discrimination, depressive symptoms, and substance use. Human Communication Research, 41(2), 204–225.
[https://doi.org/10.1111/hcre.12043]
-
Kaskutas, L. A., & Greenfield, T. K. (1997). The role of health consciousness in predicting attention to health warning messages. American Journal of Health Promotion, 11(3), 186–193.
[https://doi.org/10.4278/0890-1171-11.3.186]
-
Kim, E., Hogge, I., & Salvisberg, C. (2014). Effects of self-esteem and ethnic identity: Acculturative stress and psychological well-being among Mexican immigrants. Hispanic Journal of Behavioral Sciences, 36(2), 144–163.
[https://doi.org/10.1177/0739986314527733]
-
Kim, S. C., & Eltantawy, N. (2016). Sharing #Depression in Twitter? A content analysis of tweets and user interactions in South Korea and the U.S. Asian Communication Research, 13(1), 33–57.
[https://doi.org/10.20879/acr.2016.13.1.33]
-
Kwate, N. O. A., Meyer, I. H., Eniola, F., & Dennis, N. (2010). Individual and group racism and problem drinking among African American women. Journal of Black Psychology, 36(4), 446–457.
[https://doi.org/10.1177/0095798409355795]
-
Landrine, H., & Klonoff, E. A. (1996). The schedule of racist events: A measure of racial discrimination and a study of its negative physical and mental health consequences. Journal of Black Psychology, 22(2), 144–168.
[https://doi.org/10.1177/00957984960222002]
-
Lee-Won, R. J., Lee, J. Y., Song, H., & Borghetti, L. (2017). “To the bottle I go... to drain my strain”: Effects of microblogged racist messages on target group members’ intention to drink alcohol. Communication Research, 44(3), 388–415.
[https://doi.org/10.1177/0093650215607595]
-
Lee-Won, R. J., White, T. N., Song, H., Lee, J. Y., & Smith, M. R. (2020). Source magnification of cyberhate: Affective and cognitive effects of multiple-source hate messages on target group members. Media Psychology, 23(5), 603–624.
[https://doi.org/10.1080/15213269.2019.1612760]
-
Lee, J. (2021). The effects of racial hate tweets on perceived political polarization and the roles of negative emotions and individuation. Asian Communication Research, 18(2), 51–68.
[https://doi.org/10.20879/acr.2021.18.2.51]
-
Lee, K. M., & Nass, C. (2004). The multiple source effect and synthesized speech: Doubly-disembodied language as a conceptual framework. Human Communication Research, 30(2), 182–207.
[https://doi.org/10.1111/j.1468-2958.2004.tb00730.x]
-
Lee, R. M. (2005). Resilience against discrimination: Ethnic identity and other-group orientation as protective factors for Korean Americans. Journal of Counseling Psychology, 52(1), 36–44.
[https://doi.org/10.1037/0022-0167.52.1.36]
-
Lee, R. M., & Yoo, H. C. (2004). Structure and measurement of ethnic identity for Asian American college students. Journal of Counseling Psychology, 51(2), 263–269.
[https://doi.org/10.1037/0022-0167.51.2.263]
-
Leets, L. (2001). Responses to Internet hate sites: Is speech too free in cyberspace? Communication Law and Policy, 6(2), 287–317.
[https://doi.org/10.1207/S15326926CLP0602_2]
-
Leets, L., & Giles, H. (1999). Harmful speech in intergroup encounters: An organizational framework for communication research. In M. E. Roloff (Eds.), Communication Yearbook (Vol. 22, pp. 91–137). Routledge.
[https://doi.org/10.1080/23808985.1999.11678960]
-
Louis, W., Thomas, E., McGarty, C., Lizzio-Wilson, M., Amiot, C., & Moghaddam, F. (2020). The volatility of collective action: Theoretical analysis and empirical data. Political Psychology, 41(S1), 35–74.
[https://doi.org/10.1111/pops.12671]
-
Major, B., Hunger, J. M., Bunyan, D. P., & Miller, C. T. (2014). The ironic effects of weight stigma. Journal of Experimental Social Psychology, 51, 74–80.
[https://doi.org/10.1016/j.jesp.2013.11.009]
-
Marwick, A. E., & boyd, D. (2011). I tweet honestly, I tweet passionately: Twitter users, context collapse, and the imagined audience. New Media & Society, 13(1), 114–133.
[https://doi.org/10.1177/1461444810365313]
-
McCoy, S. K., & Major, B. (2003). Group identification moderates emotional responses to perceived prejudice. Personality and Social Psychology Bulletin, 29(8), 1005–1017.
[https://doi.org/10.1177/0146167203253466]
-
Moore, D. J., Mowen, J. C., & Reardon, R. (1994). Multiple sources in advertising appeals: When product endorsers are paid by the advertising sponsor. Journal of the Academy of Marketing Science, 22(3), 234–243.
[https://doi.org/10.1177/0092070394223004]
-
Moore, D. J., & Reardon, R. (1987). Source magnification: The role of multiple sources in the processing of advertising appeals. Journal of Marketing Research, 24(4), 412–417.
[https://doi.org/10.1177/002224378702400409]
-
Murphy, M. C., Steele, C. M., & Gross, J. J. (2007). Signaling threat: How situational cues affect women in math, science, and engineering settings. Psychological Science, 18(10), 879–885.
[https://doi.org/10.1111/j.1467-9280.2007.01995.x]
-
Nagpal, A., Lei, J., & Khare, A. (2015). To choose or to reject: The effect of decision frame on food customization decisions. Journal of Retailing, 91(3), 422–435.
[https://doi.org/10.1016/j.jretai.2014.12.001]
- Nakagawa, S. (2013, July 7). Asiana Airline crash brings out the racists on Twitter. Race Flies. http://www.racefiles.com/2013/07/07/asiana-airline-crash-brings-out-the-racists-on-twitter/
-
Noh, S., Beiser, M., Kaspar, V., Hou, F., & Rummens, J. (1999). Perceived racial discrimination, depression, and coping: A study of Southeast Asian refugees in Canada. Journal of Health and Social Behavior, 40(3), 193–207.
[https://doi.org/10.2307/2676348]
-
Outten, H. R., & Schmitt, M. T. (2015). The more “intergroup” the merrier? The relationship between ethnic identification, coping options, and life satisfaction among South Asian Canadians. Canadian Journal of Behavioural Science/Revue canadienne des sciences du comportement, 47(1), 12–20.
[https://doi.org/10.1037/a0035907]
-
Pascoe, E. A., & Richman, L. S. (2009). Perceived discrimination and health: A meta-analytic review. Psychological Bulletin, 135(4), 531– 554.
[https://doi.org/10.1037/a0016059]
-
Pascoe, E. A., & Richman, L. S. (2011). Effect of discrimination on food decisions. Self and Identity, 10(3), 396–406.
[https://doi.org/10.1080/15298868.2010.526384]
-
Peleg-Oren, N., Cardenas, G. A., Comerford, M., & Galea, S. (2012). An association between bullying behaviors and alcohol use among middle school students. The Journal of Early Adolescence, 32(6), 761–775.
[https://doi.org/10.1177/0272431610387144]
-
Phinney, J. S. (1992). The multigroup ethnic identity measure: A new scale for use with diverse groups. Journal of Adolescent Research, 7(2), 156–176.
[https://doi.org/10.1177/074355489272003]
-
Roberts, R. E., Phinney, J. S., Masse, L. C., Chen, Y. R., Roberts, C. R., & Romero, A. (1999). The structure of ethnic identity of young adolescents from diverse ethnocultural groups. The Journal of Early Adolescence, 19(3), 301–322.
[https://doi.org/10.1177/0272431699019003001]
-
Sabik, N. J., & Tylka, T. L. (2006). Do feminist identity styles moderate the relation between perceived sexist events and disordered eating? Psychology of Women Quarterly, 30(1), 77–84.
[https://doi.org/10.1111/j.1471-6402.2006.00264.x]
- Sedler, R. A. (1991). The unconstitutionality of campus bans on “racist speech”: The view from without and within. University of Pittsburgh Law Review, 53(3), 631–683.
-
Selkie, E. M., Kota, R., Chan, Y.-F., & Moreno, M. (2015). Cyberbullying, depression, and problem alcohol use in female college students: A multisite study. CyberPsychology, Behavior, and Social Networking, 18(2), 79–86.
[https://doi.org/10.1089/cyber.2014.0371]
-
Sellers, R. M., & Shelton, J. N. (2003). The role of racial identity in perceived racial discrimination. Journal of Personality and Social Psychology, 84(5), 1079–1092.
[https://doi.org/10.1037/0022-3514.84.5.1079]
-
Richman, L. S., Bennett, G. G., Pek, J., Suarez, E. C., Siegler, I., & Williams Jr, R. B. (2007). Discrimination, dispositions, and cardiovascular responses to stress. Health Psychology, 26(6), 675–683.
[https://doi.org/10.1037/0278-6133.26.6.675]
-
Snell, W. E., Johnson, G., Lloyd, P. J., & Hoover, M. W. (1991). The health orientation scale: A measure of psychological tendencies associated with health. European Journal of Personality, 5(2), 169–183.
[https://doi.org/10.1002/per.2410050208]
- Tsesis, A. (2001). Hate in cyberspace: Regulating hate speech on the Internet. San Diego Law Review, 38(3), 817–874.
-
Tynes, B. M., Giang, M. T., & Thompson, G. N. (2008). Ethnic identity, intergroup contact, and outgroup orientation among diverse groups of adolescents on the Internet. CyberPsychology & Behavior, 11(4), 459–465.
[https://doi.org/10.1089/cpb.2007.0085]
-
Vergeer, M., & Hermans, L. (2013). Campaigning on Twitter: Microblogging and online social networking as campaign tools in the 2010 general elections in the Netherlands. Journal of Computer-Mediated Communication, 18(4), 399–419.
[https://doi.org/10.1111/jcc4.12023]
-
Yim, M. C., & Jeong, H. (2020). Reframing of outgroup: How activists mobilize civic engagement. Asian Communication Research, 17(1), 110–139.
[https://doi.org/10.20879/acr.2020.17.1.110]
-
Yoo, H. C., & Lee, R. M. (2005). Ethnic identity and approach-type coping as moderators of the racial discrimination/well-being relation in Asian Americans. Journal of Counseling Psychology, 52(4), 497–506.
[https://doi.org/10.1037/0022-0167.52.4.497]
-
Zhang, Y., Dixon, T. L., & Conrad, K. (2009). Rap music videos and African American women's body image: The moderating role of ethnic identity. Journal of Communication, 59(2), 262–278.
[https://doi.org/10.1111/j.1460-2466.2009.01415.x]