
A Society that Encourages AI: The Impact of Social Influence Factors on the Adoption of Generative AI Based on Cognitive Appraisal Theory
Copyright ⓒ 2025 by the Korean Society for Journalism and Communication Studies
Abstract
The rapid diffusion of generative AI into daily life, exemplified by the swift adoption of services like ChatGPT, underscores the need to understand the factors driving its acceptance. Traditional models, such as the Technology Acceptance Model (TAM), have limitations in capturing the complex dynamics of AI adoption, particularly the role of social influence, which has expanded from immediate social circles to include online networks. This study investigates the psychological determinants of generative AI acceptance, focusing on how “social influence”, “effort expectancy”, “performance expectancy”, “trust”, and “emotion” shape the intention to use AI. Grounded in Cognitive Appraisal Theory, this study examines the sequential relationships between these factors and their impact on emotions and behavioral intentions. Data collected from 350 users in South Korea was analyzed using structural equation modeling (SEM). The findings reveal that while social influence does not directly affect performance expectancy, it significantly influences trust and effort expectancy, which, in turn, shape user emotions and intentions. The study highlights the importance of trust and social influence in the adoption process of generative AI and provides insights for future research on AI technology acceptance.
Keywords:
generative AI, social influence, AI trust, cognitive appraisal theory, AI acceptance, technology acceptanceSince the sudden launch of ChatGPT, a variety of generative AI services have rapidly permeated virtually all aspects of daily life. The ability of such services to quickly produce high-quality results in numerous creative realms, once deemed uniquely human, astonishingly attracted over one million users within the first five days of its release. The rapid advancement of digital media technologies like AI has led to such swift changes in the levels of their acceptance as well as in their consumption patterns, thus further underscoring the need for continued research.
The acceptance of new media and new technologies has long been studied using the Technology Acceptance Model (TAM). Recent discussions on AI, including studies examining user acceptance of self-driving cars (Jun, 2022), chatbot anthropomorphism (Choi & Noh, 2022), and the adoption of voice recognition agents (Upadhyay et al., 2022), have all incorporated TAM and its variables to explain why people use AI services. However, these technology acceptance mechanisms often fall short in capturing the characteristics of individuals who quickly adopt emerging technologies and media such as AI.
Accordingly, this study takes a new approach to investigating the psychological determinants of individuals’ acceptance of generative AI by acknowledging that, with the expansion of SNS, individuals’ decision-making reference groups are expanding from friends, family, and colleagues to key figures in their SNS networks (Lu et al., 2019). Given that studies indicate Millennials and Generation Z individuals often rely on the choices of others when deciding whether to use a service (Bhattacharyya et al., 2022), it is necessary to consider the growing influence of ‘social influence’ factors on the acceptance of new technologies such as generative AI. This suggests that in intelligent systems, such as generative AI, where interactions between individuals and the system are highly dynamic and social influence is strong, the causal relationships among TAM variables can change.
Meanwhile, as the structure of AI algorithms is not easily understood by humans, concerns arise that such black-box characteristics can lead to unpredictability and uncertainty, which, in turn, can result in trust issues (Choung et al., 2022). As individuals ultimately accept new technologies encountered through social influence, “trust” in the technology becomes even more crucial. Studies examining the factors contributing to trust in AI indicate (Gillath et al., 2021; Lockey et al., 2021; Rheu et al., 2021) that trust in both AI technology and the information it provides is an issue that cannot be ignored in the AI technology domain.
Thus, this study examines how causal relationships influenced by social influence operate as specific mechanisms in AI usage. Previous studies that comprehensively explore technology acceptance have confirmed the impact of social influence factors on technology adoption intention (Venkatesh et al., 2003, 2012). However, these studies primarily verify the direct impact of these factors on the intention to use AI without fully considering the relationships between social influence and other variables. Thus, such findings are inadequate for effectively explaining the decision-making mechanisms behind the diffusion of new technologies like generative AI. Given the complexity of AI technology and the highly interactive nature of its environment, it is necessary to examine the sequential occurrence of factors rather than just evaluating them independently.
Therefore, this study proposes a model that systematically examines the relationships between key variables in technology acceptance research, considering “social influence” as a factor triggering the psychological decision-making process in individuals who accept and utilize generative AI. By expanding the scope of social influence to include social networking services (SNS), this study aims to engage in an in-depth discussion about the highly interactive AI environment. Moreover, by addressing trust issues related to AI technology, which is largely perceived as a black box, this study seeks to provide a more comprehensive analysis of the AI technology acceptance process. This “AI Acceptance Model” is expected not only to highlight the importance of social influence in the acceptance of new technologies represented by artificial intelligence, but also to redefine the acceptance process.
The Spread of Generative AI and Social Influence
The expansion of ChatGPT has happened at such a rapid pace that the decision to use the technology has already been made even before individuals have had the opportunity to experience its benefits and integrate them into their decision-making processes. In today's world, where the internet and social media are pervasive, information flows swiftly from one central network hub to another, facilitating high interactivity and rapid dissemination (Barabási, 2002; Kim et al., 2012). At the individual level of adopting AI technologies, it can be inferred that as soon as a new technology is endorsed by someone’s social network hub, the technology enters their or her decision-making system.
The concept of “social influence,” which is closely related to this phenomenon, refers to “the degree to which an individual perceives that important others believe they should use the new system” (Venkatesh et al., 2003). It is one of the cognitive factors influencing individuals’ decisions to adopt technology. Social influence was originally reflected in the Theory of Planned Behavior (TPB) (Ajzen, 1991) as the concept of subjective norm, which denotes organizational and social pressures. This concept was later integrated into the Technology Acceptance Model (TAM) and applied in various studies. As technology acceptance research diversified, Venkatesh and colleagues synthesized 32 variables and concepts from eight different acceptance theories, leading to the development of the Unified Theory of Acceptance and Use of Technology (UTAUT) (Venkatesh & Davis, 1996). In UTAUT, social influence is identified as one of the primary factors predicting behavioral intention, alongside performance expectancy, effort expectancy, and facilitating conditions. The social influence factor within UTAUT encompasses related concepts such as subjective norms (TPB), social factors (MPCU), and image (Venkatesh & Davis, 1996). Like most studies on technology acceptance, UTAUT primarily explains social influence in organizational contexts. With the rise of the internet and mobile technologies, Venkatesh expanded the model to propose UTAUT2, which adapts the acceptance model for consumer contexts. In UTAUT2, the scope of social influence shifted from the attitudes of organizations or influential acquaintances to include the perspectives of larger, unspecified groups (Venkatesh et al., 2003, 2012).
The primary research question of this study is whether the constructs and scales of social influence, which are based on subjective norms influenced by specific individuals such as family, friends, colleagues, acquaintances, and social organizations, as well as injunctive norms referring to socially prevalent attitudes, can effectively explain the social relationships that are increasingly expanding through social networking services (SNS). For example, in the context of AI adoption, users may feel social pressure when individuals with higher reputations than they do use AI devices (Gursoy et al., 2019) or experience social pressure stemming from online relationships such as SNS and blogs (Bhattacharyya et al., 2022). These extended social relationships underscore the need for advanced discussions on the concept of social influence. Based on long-standing research on technology acceptance, there is theoretical consensus that social influence (SI) is a critical factor in individuals’ adoption of technology. However, it is necessary to expand the conceptualization of social influence to account for the evolving social dynamics that shape technology adoption today.
Another issue to consider regarding social influence factors is that, in the UTAUT framework, “social influence” is positioned alongside variables such as “performance expectancy” and “effort expectancy” as an antecedent factor that explains behavioral intention. Recent studies on the diffusion of new technologies suggest that social influence may precede and exert influence over other variables. This implies that individuals may perceive technologies as useful and decide to adopt them if their significant referents believe they should, even when they themselves do not have a favorable attitude toward the system (Zhang et al., 2020). Furthermore, social influence enhances the likelihood of technology adoption by influencing perceived usefulness and perceived ease of use (Joo et al., 2013). For instance, a study on the intention to use ChatGPT services has also reported cases verifying the impact of social influence on both performance expectancy and effort expectancy (Park et al., 2023).
In conclusion, this study highlights the importance of “recommendations from others” in the use of generative AI, emphasizing that as interpersonal relationships expand from offline contexts to SNS-based social connections, the scale and locus of social influence in AI acceptance models may differ from those of other factors. In this context, Lazarus’s (1991b, 1991c) “Cognitive Appraisal Theory” illustrates how the “social influence” factor operates within the relevance assessment in an individual’s decision-making, leading to a structure in which the emotions involved in the adoption of technology are intensified.
Cognitive Appraisal Theory and the Acceptance of Generative AI Technology
The rapid spread and advancement of generative AI raise the need to reassess the relationships between variables discussed in existing technology acceptance research (Venkatesh et al., 2022). Variables such as “social influence” are closely connected to the cognitive and emotional responses that should be given importance in the acceptance of AI technology.
In this regard, Lazarus’s Cognitive Appraisal Theory provides a theoretical framework applicable to the acceptance process of generative AI technology by positing that an individual’s decision-making regarding the relevance of a particular object and the coping potential (Lazarus, 1991a) undergoes a process of “cognitive-motivational-emotional” appraisal (Lazarus, 1991b, 1991c). In Cognitive Appraisal Theory, cognition is defined as “knowledge and appraisal of what is happening”. Also, knowledge consists of “situational and generalized beliefs about how things work,” while appraisal refers to “an evaluation of the personal significance of what is happening in an encounter with the environment” (Lazarus, 1991c). Based on the results of the appraisal, individuals experience positive or negative emotions toward the object, which subsequently leads to behavioral and psychological responses (Lazarus, 1999).
Individuals undergo two stages of appraisal in the decision-making process. In the “primary appraisal” stage, they consider the “relevance” of what is being evaluated (Lazarus, 1991a). It is the context for evaluating the importance of the object. When individuals lack sufficient knowledge about technology, the “social influence” factor becomes relevant to the extent that they believe important others have a positive perception of information technology use (Chang, 2012), thereby contributing to the relevance assessment.
Next, if individuals perceive the object as relevant and important to them, they undergo a “secondary appraisal” process, where they evaluate whether they can effectively cope with the object or situation (Lazarus, 1991a). This is the stage in which a cognitive assessment of motivation weighs the costs and benefits of the choice. This appraisal shapes the individual’s feelings towards their behavior and influences their decision to act (Lazarus, 1991c). In other words, cognitive efficacy fosters positive emotions, which in turn enhance the intention to use.
In Cognitive Appraisal Theory, “emotion” that arises from the cognitive appraisal process predicts intention (Lazarus, 1991b, 1991c). Emotions serve as mediators between cognitive stimuli and behavioral responses, and are recognized as outcomes of conscious and cognitive appraisals (Lim & Kim, 2019). However, unlike the concept of “attitude” in the technology acceptance model, which is a mix of cognitive and emotional evaluations, in Cognitive Appraisal Theory, cognition and emotion are sequentially separated; emotion emerges as a result of cognitively motivated evaluation. Particularly, emotions can be influenced by both positive and negative factors, reflecting this dual nature within the model’s structure (Gursoy et al., 2019). By separating cognition from emotion, acceptance models can more accurately reflect the facilitative emotions associated with new technologies as well as any ambivalence induced by ethical concerns. This means that the influence exerted by a reference group before using any new technology can have a cascading effect on an individual’s cognitive and emotional judgments about AI. Therefore, based on this process, the way individuals respond to new technologies like generative AI is likely determined through stages, driven by emotions that stem from cognitive appraisal of specific stimuli. This approach allows for an explanation of the sequence of occurrences of factors affecting usage intention based on their causal characteristics rather than simply evaluating all antecedents as independent factors.
Meanwhile, social influence has been reported in previous studies as an antecedent factor influencing an individual’s belief system related to the perceived ease of use and usefulness of technology ( Joo et al., 2013; Park et al., 2023). Approaching social influence through the lens of Cognitive Appraisal Theory, social influence serves as a stage where users evaluate the relevance of a technology to themselves (Gursoy et al., 2019; Lu et al., 2019; Vitezić & Perić, 2021). Furthermore, these cognitive evaluations of performance expectancy and effort expectancy are also expected to impact emotions associated with the use of AI. Ultimately, considering the characteristics and relationships of these factors, one can infer a sequential relationship among the variables within the mechanism of generative AI acceptance.
Trust in the Acceptance of Generative AI Technology
Trust (Söllner et al., 2016), which is considered another major predictor in the adoption of new technologies, can play an integral role in the primary appraisal stage of Cognitive Appraisal Theory (Lazarus, 1991b). Trust encompasses a wide range of areas and has been identified as influential in the use of online services, including new information systems (Tung et al., 2008), online games (Wu & Liu, 2007), banking (Suh & Han, 2002), social network sites (Sledgianowski & Kulviwat, 2009), shopping (Gefen et al., 2003), etc. More recently, research in AI robotics has conceptualized trust as a “comprehensive cognitive belief that is the result of a user’s evaluation of sub-factors such as performance, functionality, and working environment” (Chi et al., 2021; Tussyadiah et al., 2020).
Based on its characteristics, trust has consistently been found to have a significant positive influence on an individual’s efficacy in using a system (Wu et al., 2011). Especially in relation to the social influence factor, it has been reported that individuals’ decision-making can be influenced by others, which in turn affects their trust behavior (Wei et al., 2019). Trust has also been identified as a mediator between factors such as social influence and intention to use (Chung, 2019). Similarly, social influence has been shown to have a significant effect on trust in information provided by the Internet (Chin et al., 2009).
Particularly in the functional aspect, trust in AI has been shown to influence perceived usefulness (Choung et al., 2022), and in the context of generative AI, trust has been identified as a significant positive predictor of perceived usefulness (Kim, 2024). As a key variable in the cognitive appraisal process of technology acceptance, trust has also been reported to be significantly related to the emotions that result from the evaluation. For example, a study examining the intention to use AI speakers explored how trust (rational-function and emotional-affectivity) influences attitudes and intentions (Jun, 2023). The findings showed that the path between rational (functional) trust and attitude was significant, but the path between emotional (affective) trust and attitude was not significant. Additionally, trust in AI technology has been shown to positively influence attitudes toward the technology (Lee & Jun, 2022).
Taken together, these findings suggest that trust plays a pivotal role when discussing the stages of acceptance of generative AI technologies in the context of the cognitive evaluation process of Cognitive Appraisal Theory (Lazarus, 1991c). However, as a number of previous studies have pointed out, there is still a lack of consensus on the role of trust in AI technology acceptance (Chi et al., 2023). Given the complexities of the trust factor and the unique and varied contexts of AI technologies, a distinct approach may be required for studying trust in AI in comparison to other areas (Chi et al., 2021; Tussyadiah et al., 2020; Yagoda & Gillan, 2012). Therefore, it is essential to verify whether the complex relationships between key variables related to technology acceptance, such as social influence, performance expectancy, and emotions, are similarly evident in the domain of generative AI.
Research Model and Hypotheses
This study focuses on the factor of social influence in AI acceptance and proposes a model to explain the sequential decision-making process it triggers. To achieve this, it tests Lazarus’s (1991b, 1991c) cognitive-motivational-emotional framework, building upon the variables integrated into the UTAUT. While the UTAUT primarily identifies direct factors influencing behavioral intention, the proposed model emphasizes examining the relationships between these factors.
The UTAUT identifies the impact of key factors, including Social Influence, Performance Expectancy, Effort Expectancy, and Facilitating Conditions, on technology acceptance behaviors. Performance Expectancy is defined as the degree to which using an IT system provides advantages to users in performing their tasks and corresponds to the belief system of perceived usefulness (PU) in TAM. Effort Expectancy refers to the perceived ease of use (PEOU) of a technology and is defined as the degree to which a user believes that using a particular system will require minimal effort (Venkatesh et al., 2003). Facilitating Conditions, while directly influencing IT system use in UTAUT, are limited to organizational contexts (Venkatesh et al., 2003) and have been found to be less predictive of behavioral intention in consumer contexts (Gansser & Reich, 2021). Given these limitations, this study excludes Facilitating Conditions from its analysis. Additionally, in the UTAUT framework, the aforementioned variables function as antecedents positioned at the same level. However, this study integrates Lazarus’s (1991b, 1991c) cognitive- motivational-emotional framework to explain the phased influences and relationships between these variables.
As a first step, this study focuses on “social influence,” a key variable in UTAUT and a factor hypothesized to trigger the diffusion of generative AI. According to prior research, individuals using automated vehicles (AVs) perceive AV systems as useful if important others hold such beliefs, even if the individuals themselves are not favorable toward the system (Zhang et al., 2020). In other words, social influence is considered to have a direct impact on performance expectancy (PE). In contrast, the relationship between social influence and effort expectancy (EE) is less consistent. While most studies report that social influence positively impacts effort expectancy, other research indicates negative effects (Man et al., 2025). Thus, the relationship between social influence and effort expectancy requires further investigation. Additionally, social influence consistently impacts trust in system use. This effect is particularly evident when users lack direct knowledge of the system, leading them to seek and trust the opinions of others (Li et al., 2008). Such prior studies suggest that recommendations and opinions encountered within social networks serve as critical cues in evaluating the relevance of technologies like generative AI (Lu et al., 2019; Park et al., 2023). Based on the Cognitive Appraisal Model, when users initially evaluate relevance through social influence (primary appraisal), it subsequently affects secondary appraisal processes, including “effort expectancy,” “performance expectancy,” and “trust” (Chin et al., 2009; Joo et al., 2013; Park et al., 2023). At this stage, the relationships among social influence, performance expectancy, effort expectancy, and trust fall within the domain of cognitive appraisal, determining the perceived cognitive efficacy of AI systems (Gursoy et al., 2019; Lu et al., 2019; Vitezić & Perić, 2021). Based on this framework, the following hypotheses can be established.
- H1. Social influence on AI users will have a positive influence on their effort expectancy.
- H2. Social influence on AI users will have a positive influence on their performance expectancy.
- H3. Social influence on AI users will have a positive influence on their trust in AI.
In the case of “performance expectancy,” which relates to how useful generative AI technology is perceived to be, if users feel that the technology is difficult to use in terms of “effort expectancy,” they may negatively evaluate its potential contribution to performance (Davis, 1989; Venkatesh et al., 2003). In other words, as effort expectancy reflects an individual’s assessment of the ease of use of generative AI technology, this assessment connects to performance expectancy, which evaluates the technology’s usefulness. Consequently, the easier users perceive the technology to be, the more likely they are to positively evaluate its potential to contribute to their performance. Meanwhile, “trust” also acts as a critical factor mediating the relationship between social influence and cognitive appraisal variables, such as performance expectancy. Trust reduces the uncertainty users feel about generative AI technology and enhances the perceived reliability of the system. Prior research has shown that social influence can have a positive effect on online information trust (Chin et al., 2009), and that users who trust AI systems are more likely to have higher expectations of the accuracy and efficiency of the system’s results (Choung et al., 2022; Kim, 2024). Social influence fosters positive evaluations of generative AI’s performance through trust, which in turn increases the likelihood of users adopting the technology. These relationships among variables align with the sequential processes outlined in the secondary appraisal phase of Cognitive Appraisal Theory (Lazarus, 1991a), suggesting that they play a critical role in individuals’ adoption of generative AI technology.
- H4. AI users’ effort expectancy will have a positive influence on their performance expectancy.
- H5. AI users’ trust in AI will have a positive influence on their performance expectancy.
“Emotion” is a variable formed as a result of cognitive appraisal and plays a pivotal mediating role in predicting users’ behavioral intentions during the technology adoption process (Lazarus, 1991b). In particular, the more positive outcomes that are derived from cognitive appraisals such as “effort expectancy” and “performance expectancy,” the more likely users are to develop positive emotions toward the technology, which directly influences their “intention to use” (Gursoy et al., 2019; Vitezić & Perić, 2021). Furthermore, the context of prior findings reporting the positive effects of “trust,” another variable influencing the cognitive appraisal process, on emotion can also be applied here (Jun, 2023; Lee & Jun, 2022). Therefore, in the context of generative AI adoption, emotion is not merely an outcome variable but a decisive step leading to the intention to use. Consequently, the hypotheses derived to verify the relationships among variables such as effort expectancy, performance expectancy, emotion, and intention to use in generative AI adoption are as follows.
- H6. AI users’ effort expectancy will have a positive influence on their emotions.
- H7. AI users’ performance expectancy will have a positive influence on their emotions.
- H8. AI users’ trust in AI will have a positive influence on their emotions.
- H9. AI users’ emotions will have a positive influence on their intention to use AI.
Additionally, the research model constructed based on the nine hypotheses proposed in this study is illustrated in Figure 1. This research model demonstrates originality by comprehensively exploring the mediating and sequential effects among variables that have been overlooked in existing technology acceptance models (TAM, UTAUT). While the UTAUT framework emphasizes the direct relationship between independent variables and behavioral intention, this study applies Cognitive Appraisal Theory to analyze the interactive relationships among social influence, cognitive appraisal, trust, and emotion.
Through this approach, the model clarifies the sequential relationships between variables in the complex process of adopting advanced technologies such as generative AI, while also highlighting the significance of emotional and trust-related factors.
METHOD
Data Collection
This study surveyed adults over the age of 20 in South Korea with experience using generative AI. The survey was conducted by the research agency Research & Research, using a random sample of users during the week of December 13–20, 2023. This study adhered to ethical guidelines for human research. Participation was voluntary, and all participants were informed about the study’s purpose, their right to withdraw at any time, and the confidentiality of their responses. Informed consent was obtained from all participants, and data were collected and stored in compliance with ethical research standards to ensure anonymity and confidentiality.
Through this process, we ultimately analyzed data from 350 respondents after excluding unreliable responses. The demographic breakdown of the respondents included 151 males (43.1%) and 199 females (56.9%). By age, 132 respondents (37.7%) were in their 20s, 106 (30.3%) were in their 30s, and 112 (32.0%) were aged 40 or older.
In terms of usage frequency, the most common response was using generative AI once a month (26.3%), while a small group (8%) reported using it almost daily. The primary purpose for using generative AI was to find information (26%), and the least common purpose was creation (7.1%). Usage was evenly distributed across activities related to learning and education, work, play and hobbies, and life and convenience.
When participants were asked to identify their favorite or most frequently used services, ChatGPT (GPT-3.5) was the most popular at 44.1%, followed by Bard, CLOVA X, New Bing, and ChatGPT (GPT-4). Other services mentioned included Playground, Photoshopai, Carat, A., and Wrtn (2 respondents).
Measures
To measure social influence, a five-item scale was constructed by utilizing scales from Bhatt (2022), Lu et al. (2019), Gursoy et al. (2019), and Bhattacharyya et al. (2022). As this study aims to address relationships formed on SNS as key social factors influencing the acceptance of generative AI, we have developed and cited a scale that reflects this. Responses to all items in this study were measured on a five-point Likert scale ranging from “strongly disagree” (1) to “strongly agree” (5) (M = 3.24, SD = .81).
Effort expectancy was based on the scales developed by Venkatesh et al. (2003, 2012) and Strzelecki (2024). A total of four items were measured. Each item was rated on 5-point Likert scale ranging from “strongly disagree” (1) to “strongly agree” (5) (M = 3.54, SD = .73).
Performance expectancy was a three-item scale adapted from Venkatesh et al. (2003, 2012) and Strzelecki (2024). All items were rated on a 5-point Likert scale ranging from “strongly disagree” (1) to “strongly agree” (5) (M = 3.67, SD= .67).
To measure AI trust, we used the “Functional Trust Scale” developed by Choung et al. (2022) to assess five items. Each item was rated on a 5-point Likert scale ranging from “strongly disagree” (1) to “strongly agree” (5) (M = 3.40, SD = .75).
To measure emotions, we adapted the semantic differential scale developed by Lin et al. (2020) and assessed three items: “hopeless–hopeful,” “dissatisfied–satisfied,” and “annoyed–pleasant,” rated on a 5-point scale (M = 3.52, SD = .69).
For intention to use, three items were constructed based on the scale developed by Venkatesh et al. (2003, 2012). Each item was rated on a 5-point Likert scale ranging from “strongly disagree” (1) to “strongly agree” (5) (M = 3.53, SD = .72).
RESULTS
Measurement Models: Confirmatory Factor Analysis (CFA) and Validity Test
This study employed structural equation modeling (SEM) to evaluate the research model and hypotheses, following the two-step approach proposed by Anderson and Gerbing (1988). Initially, a confirmatory factor analysis was carried out using RStudio to determine whether the variables of the aforementioned measures accurately represented each construct. The standardized estimate, composite reliability (C.R.), and average variance extracted (AVE) for each observational variable, confirming the validity of the constructs are shown in Table 1.
The confirmatory factor analysis showed that the overall goodness-of-fit of the measurement model was good, and the 23 measurements for the six constructs had high standardized estimates of at least .645 (EM3). Adequate measurement of the constructs should manifest convergent validity between the measurements and the constructs, as well as discriminant validity among the different constructs.
Three criteria were applied to determine the convergent validity of the measurement model, as suggested by Fornell and Larcker (1981). First, the average variance extracted (AVE), which represents the amount of variance explained by the observed variables for the latent variables in each construct, should be greater than .5; second, the composite reliabilities (C.R.) for each construct, which measure the internal consistency of the data collected, should exceed .7; and third, the standardized estimate for all items should be greater than .5.
When examining the convergent validity of the confirmatory factor analysis according to this criterion, the standardized estimates, which represent the explanatory power of each observed variable on the constructs, exceeded the minimum required value of .5 for all items. The AVE values for the constructs all surpassed the required minimum of .5. The composite reliabilities of the six constructs were all above .7, with a minimum value of .757, indicating that convergent validity is secured.
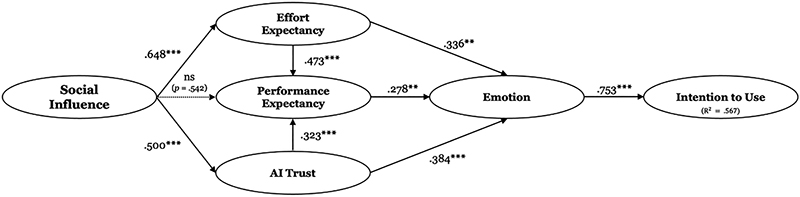
Results of Structural Model Path AnalysisNote 1. Coefficients are standardized regression weights.Note 2. CMIN/dF (N = 350) = 2.444, p < .001, TLI = .909, CFI = .920, RMSEA = .064, SRMR = .078
Meanwhile, the discriminant validity of the measurement model can be deemed valid when the average variance extracted (AVE) value found in convergent validity is greater than the square of the correlation coefficient between two different constructs (AVE > φ²). As shown in Table 2, the square root of the AVE is larger than the correlation coefficients between each construct. Therefore, the constructs used in this study can be considered distinct concepts.
Based on the data above, the goodness-of-fit of the measurement model is good, and a total of 23 observed variables strongly explain the six constructs, indicating that the constructs are clearly distinguishable from each other.
Structural Model Path Analysis
Based on the results of the measurement model’s validity, a path analysis of the structural model was conducted to test the research model and hypotheses. First, the model fit of the collected data was generally good (CMN/dF (N = 350) = 2.444, p < .001, TLI = .909, CFI = .920, RMSEA = .064, SRMR = .078). Accordingly, the results of the path analysis regarding the primary influence of the social influence variable on the use of generative AI are presented in Figure 2.
The analysis along each path in the model shows that social influence on generative AI users has a strong influence on the users’ effort expectancy (H1: β = .648, p < .001). Social influence also greatly affects users’ trust in AI (H3: β = .500, p < .001). However, the influence of social influence on users’ performance expectancy (H2) is not significant (p > .05).
We also found that generative AI users’ effort expectancy (H4: β = .473, p < .001) and AI trust (H5: β = .323, p < .001) significantly influence performance expectancy. The influence of effort expectancy on performance expectancy aligns with findings from previous technology acceptance studies. When users perceive AI as easy to use, they are more likely to believe that it will enhance their job performance. Furthermore, the more users trust AI, the higher their expectations of AI’s performance are likely to be. Additionally, the influences of effort expectancy (H6: β = .336, p < .01), performance expectancy (H7: β = .278, p < .01), and AI trust (H8: β = .384, p < .001) on generative AI users’ emotions were all found to be significant.
Finally, we examined the influence of users’ emotions on their intention to use generative AI. The results show that positive emotions strongly influence the intention to use generative AI (H9: β = .754, p < .001). Additionally, the research model validated in this study was found to be able to predict the intention to use generative AI in approximately 56.7% of cases (R² = .567), based on the squared multiple correlation (SMC) value. The hypothesis testing results of the model are confirmed in Table 3. All paths, except for the influence of the social influence variable on performance expectancy, were positive, suggesting that the cascade of cognitive appraisal triggered by the social influence variable in the use of generative AI can be discussed as a significant technology acceptance process.
In addition to the direct influences presented through hypothesis testing, the indirect influences of the variables were analyzed. The indirect influences of social influence, trust, and other determinants of intention to use are detailed in Table 4. The significance test for the indirect influences was conducted based on bootstrapping with 5,000 samples.
The results of the analysis indicate that while social influence does not directly affect performance expectancy, it likely has an indirect effect on performance expectancy (.393***) through effort expectancy and AI trust. It also tends to have an indirect effect on emotion (.340***) and intention to use (.469*). Effort expectancy has an indirect effect on emotion (.114**) but does not show a statistically significant indirect effect on intention to use. AI trust has an indirect effect on emotion (.073*) and intention to use (.309**), while performance expectancy has an indirect effect on intention to use (.210*).
The primary objective of this research model is to identify the factors that explain the intention to use. The results show that emotion (.753***), with a direct influence on intention to use, has the strongest effect. According to Cognitive Appraisal Theory, while emotion has the greatest influence on intention to use, social influence also plays a notable indirect role in shaping intention to use through the cognitive appraisal-emotion stage (.469*). This positions social influence at the outset of the development process in each stage of the research model and has the largest indirect influence on the ultimate goal of intention to use. A key observation is that social influence has an indirect effect on performance expectancy. Social influence contributes to performance expectancy (.393***) through the mediating effects of trust (.135***) and effort expectancy (.258***). These findings suggest that while social influence does not directly determine intention to use through performance expectancy, its indirect effects via effort expectancy and trust play a role in shaping intention to use.
DISCUSSION
This study, based on Cognitive Appraisal Theory, proposes an acceptance model focused on generative AI with the goal of explaining the cascading decision-making process triggered by social influence factors in individuals’ acceptance of AI technology. We analyzed the structural relationships affecting the intention to use generative AI, focusing on the key variables of “social influence,” “performance expectancy,” “effort expectancy,” “trust,” and “emotion”, which are commonly featured in technology acceptance studies such as the UTAUT model.
The results indicated that all nine hypotheses, except for the relationship between social influence and performance expectancy, had a significant positive effect. These findings not only support those of previous studies but also confirm the role of social influence in the process of new technology acceptance, particularly in today’s highly interactive media environment.
The Impact of Social Influence
Considering that social influence variables are becoming increasingly important in maintaining relationships with others as the influence of SNS continues to grow (Lim & Kim, 2018), it is noteworthy that the causal relationship between variables reflecting this phenomenon was found to be significant. This finding indicates that the selection of new and previously unexplored technologies, such as generative AI, is strongly influenced by the initial impact of extended reference groups on social media. The accelerated diffusion of new technologies as they emerge can be explained by the fact that individuals are more likely to comply with and internalize the experiences and recommendations of those with a strong reputation for the technology, rather than experimenting with it themselves and evaluating the benefits on their own.
One study (Kim et al., 2021) found that users are skeptical about accepting AI-generated recommendations due to concerns about the potential misuse of generative AI and social bias, suggesting that the ethical discourse surrounding AI can influence users’ decisions. Additionally, widespread concerns about AI, such as bias and instability, lack of transparency, and job displacement, are considered factors that undermine trust in these systems (Chakravorti, 2024). Nevertheless, the findings of this study show that greater social influence is associated with higher trust in AI. The social influence factor not only increases trust in generative AI but also helps individuals become more comfortable and accepting of generative AI.
On the other hand, Hypothesis 2, which proposed that social influence would positively influence performance expectancy, was not supported. This indicates that even if social influence is high, it does not necessarily lead to the expectation that AI will enhance individual performance. The evaluation of a new technology’s usefulness is more likely to be determined by an individual’s cognitive assessment rather than by social influence, demonstrating that performance expectancy tends to form as a post-experience evaluation rather than as an a priori expectation. Therefore, while social influence increases trust in AI and helps users positively assess its usability, the judgment of AI’s usefulness is more significantly shaped by an individual’s experiential evaluation, distinguishing this study from previous research.
These findings suggest that social influence is a key facilitator in AI adoption, but it does not directly shape all stages of the evaluation process. For AI technologies to spread rapidly, it is crucial to establish an environment in which users can trust the technology and access it easily, even without direct experience. This implies the need to consider social recommendation systems and trust-centered AI service design strategies.
Stepwise Causality in a Model based on Cognitive Appraisal Theory
The social influence variable has been treated as an antecedent factor explaining behavioral intention alongside performance expectancy and effort expectancy in the technology adoption process (Venkatesh et al., 2003, 2012). However, in this study, we tested whether “social influence” triggers the intention to use through the process of cognitive, motivational, and emotional evaluation of individuals through a model based on Cognitive Appraisal Theory. Instead of evaluating all antecedents as independent factors, we delineated the sequence of occurrences according to the characteristics of the factors affecting the intention to use, explaining the chain of decision-making mechanisms of individuals adopting AI.
According to Cognitive Appraisal Theory, stimuli that are not relevant to the user do not trigger evaluation or emotions (Lazarus, 1991a, 1991b, 1991c). This study hypothesizes that users assess the relevance of AI usage to themselves through social influence. If AI is perceived to have a positive impact on an individual’s values or belief system, the likelihood of trusting AI increases, which subsequently leads to an evaluation of its ease of use and usefulness (Chin et al., 2009). In other words, the more users perceive AI as easy to use, trustworthy, and beneficial for their work performance, the more likely they are to develop positive emotions, which in turn result in AI adoption behavior (Gursoy et al., 2019; Lim & Kim, 2019).
In the Technology Acceptance Model (TAM) and the Unified Theory of Acceptance and Use of Technology (UTAUT), attitude is considered a factor influencing behavioral intention; however, attitude is a concept that combines cognitive evaluation and emotional response (Venkatesh et al., 2003). In contrast, this study, grounded in Cognitive Appraisal Theory, explains the sequential process whereby users first recognize AI as easy to use, useful, and trustworthy through social influence, and subsequently develop emotional responses.
In summary, regarding the adoption of new technologies, we now live in a world in which individuals are exposed to social evaluations of the technology before they actually use it and determine its usefulness and ease of use. Users’ cognitive evaluation enhances positive emotions toward the technology. Therefore, practitioners seeking to promote the diffusion of new technologies should focus their efforts on stimulating the cognitive domain of technology perception. A significant portion of this effort can be effectively driven by individuals with a strong reputation for their expertise in the technology. These findings are crucial for the acceptance of new technologies and could lead to more diverse and improved policy and industry strategies.
Ethical Issues and Trust in AI
Ethical discourse surrounding AI can influence users’ choices regarding AI adoption. Trust is the process of building interdependence between communicating parties while overcoming risk. In this context, trust can be categorized as either human or mechanical (Choung et al., 2022). In this study, both human trust and mechanical trust were tested, but human trust was not validated in the confirmatory factor analysis. This suggests that participants did not perceive AI as human or encountered problems that made it difficult for users to distinguish it from other latent variables.
Ultimately, trust in technology and systems is qualitatively distinct from trust in people. Humans are moral agents, but it is difficult to assign a moral agent role to technology. Based on previous research, we measured trust in relation to functionality and expertise dependency at a technical level. We found that trust in AI functionality had a significant direct (.384***) and indirect (.073*) influence on positive feelings towards generative AI. When determining intentions to use AI, trust in the functionality of the AI system emerges as an important factor in shaping perceptions of its ethical aspects. This suggests that the system is perceived as sufficiently expert to be trusted and relied upon. The more positive the user’s experience of being informed and influenced by someone with a technical reputation for AI, the higher the level of trust in AI. A higher level of trust in AI leads to greater performance expectations and stronger positive emotions.
The total influence of AI trust and effort expectancy on emotions appears to be similar in magnitude, but the indirect influence of effort expectancy on intention to use is not significant, indicating that the influence of AI trust on intention to use is stronger. If we disregard the direct influence of emotion on intention to use, we observe that social influence plays an initial role, followed by trust in AI. These results suggest that trust in AI is as crucial a factor in AI adoption as its usability.
Research Achievements and Limitations
In current communication research, there is no consensus on which theoretical framework best reflects the reality of how people understand and adopt AI (Lee, 2020). While generative AI has shown promising results in productivity and creativity, we do not know how users’ psychological decision-making mechanisms operate during the diffusion of the technology. This study proposed that the rapid diffusion of generative AI reflected the receptive characteristics of users driven by social influences. Furthermore, by modeling these characteristics based on usability and functional trust, it provided a foundational framework for communication AI research.
The significant role of social influence has been well-documented in prior research on AI and the adoption of new technologies. However, this study empirically confirms that social influence is not merely a factor affecting intention to use but serves as a key variable that shapes AI trust and mediates performance expectancy. In particular, social influence plays the most significant role in shaping trust. Even when cognitive appraisals of AI are unstable due to ethical concerns, positive evaluations from peers, prominent figures, or influencers can enhance users’ trust in AI. When trust increases through social influence, users are more likely to positively assess AI’s performance. Ultimately, social influence has the strongest indirect effect on the final goal of intention to use and serves not merely as an external factor but as a crucial starting point in the process of building trust in new technologies.
The decisive role of social influence was made evident through the use of a stepwise framework aimed at understanding the relationships between variables, rather than focusing solely on their direct impact on intention to use, as seen in UTAUT. Research on UTAUT suggests examining the multi-stage relationships within technology acceptance (Venkatesh et al., 2012), and this study represents a response to that proposal.
As social networking services (SNS) continue to expand, the importance of social influence in maintaining relationships with others has grown significantly. Social influence now encompasses relationships formed through SNS. Accordingly, this study redefined the concept of social influence beyond the existing frameworks of subjective norms and injunctive norms, expanding it to include social media networks and online influencers. Users tend to rely on the decisions of others when adopting new services (Bhattacharyya et al., 2022), and this tendency may be even more pronounced in the case of emerging technologies such as AI. This study not only empirically identified the sequential relationship through which social influence affects AI adoption via trust but also validated the reliability and validity of the expanded concept of social influence in AI adoption. This contribution is particularly significant as it proposes an adoption model that reflects the social influence of digital networks in the diffusion of new technologies.
Nevertheless, this study has several limitations. First, the survey was limited to people who already had experience using generative AI, which may not allow for a comprehensive understanding in various contexts. In particular, given that the majority of generative AI users during the survey period were concentrated in relatively younger age groups, there may be limitations in generalizing the findings to older adults or individuals with lower digital literacy. Considering the influence and rapid spread of generative AI, it is reasonable to predict that the user demographics will expand to a broader age range. Therefore, future research should expand its focus to include older adults as generative AI users and comprehensively consider factors such as the reasons for non-adoption of generative AI. It is also necessary to discuss the barriers to generative AI technology adoption and strategies to address them.
Second, this study focused on the early stages of generative AI technology acceptance and examined the role of social influence. According to previous research (Venkatesh & Davis, 2000), the influence of reference groups may diminish over time. As individuals accumulate experience, they increasingly rely on their own judgment of usability to make decisions. This study has a limitation in that it assessed the magnitude of social influence based on its effect at the time of the study, rather than evaluating it according to the users’ level of experience. This limitation could be addressed in future research by conducting longitudinal studies that more precisely measure how social influence evolves over time.
Third, this study focuses on exploring the impact of positive social influence on the adoption of generative AI, building on the fact that numerous prior studies have addressed social influence in a favorable context (Park et al., 2023; Venkatesh et al., 2003). This aligns with existing research suggesting that positive social influence strengthens the intention to adopt technology through the mediating roles of trust in technology and cognitive appraisal (Chin et al., 2009; Lu et al., 2019). However, it is necessary to acknowledge the potential downsides of social influence that may exist beneath the surface of these discussions. Social influence does not always operate positively; in some cases, it may hinder technology adoption. This study identifies a limitation in not fully capturing these potential adverse effects and the complexities of technology adoption. Therefore, future research should analyze the interplay between positive and negative social influences in the AI adoption process. By doing so, it will be possible to explore the mechanisms of technology acceptance in a more comprehensive manner, accounting for diverse contexts and environments.
References
-
Ajzen, I. (1991). The theory of planned behavior. Organizational Behavior and Human Decision Processes, 50(2), 179–211.
[https://doi.org/10.1016/0749-5978(91)90020-T]
-
Anderson, J. C., & Gerbing, D. W. (1988). Structural equation modeling in practice: A review and recommended two-step approach. Psychological Bulletin, 103(3), 411–423.
[https://doi.org/10.1037/0033-2909.103.3.411]
- Barabási, A.-L. (2002). Linked: The new science ofnetwork. Dongasiabooks.
-
Bhatt, K. (2022). Adoption of online streaming services: moderating role of personality traits. International Journal of Retail & Distribution Management, 50(4), 437-457.
[https://doi.org/10.1108/IJRDM-08-2020-0310]
-
Bhattacharyya, S. S., Goswami, S., Mehta, R., & Nayak, B. (2022). Examining the factors influencing adoption of Over The Top (OTT) services among Indian consumers. Journal of Science and Technology Policy Management, 13(3), 652–682.
[https://doi.org/10.1108/jstpm-09-2020-0135]
- Chakravorti, B. (2024, May 3). AI’s trust problem. Harvard Business Review. https://hbr.org/2024/05/ais-trust-problem
-
Chang, A. (2012). UTAUT and UTAUT 2: A review and agenda for future research. The Winners, 13(2), 106–114.
[https://doi.org/10.21512/tw.v13i2.656]
-
Chi, O. H., Chi, C. G., Gursoy, D., & Nunkoo, R. (2023). Customers’ acceptance of Artificially Intelligent service robots: The influence of trust and culture. International Journal of Information Management, 70, 102623.
[https://doi.org/10.1016/j.ijinfomgt.2023.102623]
-
Chi, O. H., Jia, S., Li, Y., & Gursoy, D. (2021). Developing a formative scale to measure consumers’ trust toward interaction with Artificially Intelligent (AI) social robots in service delivery. Computers in Human Behavior, 118, 106700.
[https://doi.org/10.1016/j.chb.2021.106700]
-
Chin, A. J., Wafa, S. A. W. S. K., & Ooi, A. Y. (2009). The effect of internet trust and social influence towards willingness to purchase online in Labuan, Malaysia. International Business Research, 2(2), 72–81.
[https://doi.org/10.5539/ibr.v2n2p72]
-
Choi, J. H., & Noh, G. Y. (2022). AI chatbot’s anthropomorphic effects on parasocial interaction with AI chatbot: The mediating effects of perceived homophily and social presence. The Korean Journal of Advertising and Public Relations, 24(4), 521–549.
[https://doi.org/10.16914/kjapr.2022.24.4.521]
-
Choung, H., David, P., & Ross, A. (2022). Trust in AI and its role in the acceptance of AI technologies. International Journal of Human- Computer Interaction, 39(9), 1727–1739.
[https://doi.org/10.1080/10447318.2022.2050543]
- Chung, B. G. (2019). Influential factors on technology acceptance of mobile banking: Focusing on mediating effect of trust. Journal of Distribution and Management Research, 22(1), 101–115.
-
Davis, F. D. (1989). Perceived usefulness, perceived ease of use, and user acceptance of information technology. MIS Quarterly, 13(3), 319–340.
[https://doi.org/10.2307/249008]
-
Fornell, C., & Larcker, D. F. (1981). Evaluating structural equation models with unobservable variables and measurement error. Journal of Marketing Research, 18(1), 39–50.
[https://doi.org/10.1177/002224378101800104]
-
Gansser, O. A., & Reich, C. S. (2021). A new acceptance model for artificial intelligence with extensions to UTAUT2: An empirical study in three segments of application. Technology in Society, 65, 101535.
[https://doi.org/10.1016/j.techsoc.2021.101535]
-
Gefen, D., Karahanna, E., & Straub, D. W. (2003). Trust and TAM in online shopping: An integrated model. MIS Quarterly, 27(1), 51–90.
[https://doi.org/10.2307/30036519]
-
Gillath, O., Ai, T., Branicky, M. S., Keshmiri, S., Davison, R. B., & Spaulding, R. (2021). Attachment and trust in Artificial Intelligence. Computers in Human Behavior, 115, 106607.
[https://doi.org/10.1016/j.chb.2020.106607]
-
Gursoy, D., Chi, O. H., Lu, L., & Nunkoo, R. (2019). Consumers acceptance of Artificially Intelligent (AI) device use in service delivery. International Journal of Information Management, 49, 157–169.
[https://doi.org/10.1016/j.ijinfomgt.2019.03.008]
- Joo, Y. J., Seol, H. N., & Yoo, N. Y. (2013). An analysis of the impact of cyber university students’ self-efficacy, subjective norms on the behavioral intention to use mobile web service. The Journal of Korean Association of Computer Education, 16(3), 1–12.
- Jun, J. W. (2022). Media effects and mediating roles of playfulness on acceptance of autonomous driving. Broadcasting & Communication, 23(2), 5–26.
-
Jun, J. W. (2023). Effect of AI technology perception on purchase intention of AI speakers: A focus on mediating roles of dual trust. Journal of Cybercommunication Academic Society, 40(3), 101–126.
[https://doi.org/10.36494/JCAS.2023.09.40.3.101]
- Kim, B. S., Bae, S. H., & Baek, S. I. (2012). Exploring the network structure of online communities by using social network analysis. Entrue Journal of Information Technology, 11(1), 59–72.
-
Kim, C.-W. (2024). University learners’ intention to use ChatGPT using the extended technology acceptance model: Focusing on personal innovativeness, perceived trust, and perceived risk. Journal of the Korea Contents Association, 24(2), 462–475.
[https://doi.org/10.5392/JKCA.2024.24.02.462]
-
Kim, J., Giroux, M., & Lee, J. C. (2021). When do you trust AI? The effect of number presentation detail on consumer trust and acceptance of AI recommendations. Psychology & Marketing, 38(7), 1140–1155.
[https://doi.org/10.1002/mar.21498]
-
Lazarus, R. S. (1991a). Emotion and adaptation. Oxford University Press.
[https://doi.org/10.1093/oso/9780195069945.001.0001]
-
Lazarus, R. S. (1991b). Cognition and motivation in emotion. American Psychologist, 46(4), 352–367.
[https://doi.org/10.1037/0003-066X.46.4.352]
-
Lazarus, R. S. (1991c). Progress on a cognitive- motivational-relational theory of emotion. American Psychologist, 46(8), 819–834.
[https://doi.org/10.1037//0003-066X.46.8.819]
- Lazarus, R. S. (1999). Stress and emotion: A new synthesis. Springer Publishing Company.
-
Lee, D., & Jun, J. W. (2022). Influences of AI technology and news consumption on the intention to watch baseball games of AI umpire. Journal of Media Economics & Culture, 20(4), 7–31.
[https://doi.org/10.21328/JMEC.2022.11.20.4.7]
- Lee, J. H. (2020). Artificial Intelligence and media-communication studies. Journal of communication research, 57(3), 5–40.
-
Li, X., Hess, T. J., & Valacich, J. S. (2008). Why do we trust new technology? A study of initial trust formation with organizational information systems. The Journal of Strategic Information Systems, 17(1), 39–71.
[https://doi.org/10.1016/j.jsis.2008.01.001]
-
Lim, I. J., & Kim, Y. W. (2019). The influencing path of the types of climate change reporting on behavioral intentions: A focus on the cognitive appraisal theory of emotion. Korean Journal of Communication & Information, 96, 37–72.
[https://doi.org/10.46407/kjci.2019.08.96.37]
-
Lim, S. H., & Kim, S. H. (2018). Factors affecting user intentions in omnichannel environment: Focusing on unified theory of acceptance and use of technology. The Korean Journal of Advertising, 29(4), 95–129.
[https://doi.org/10.14377/KJA.2018.5.31.95]
-
Lin, H., Chi, O. H., & Gursoy, D. (2020). Antecedents of customers’ acceptance of Artificially Intelligent robotic device use in hospitality services. Journal of HospitalityMarketing & Management, 29(5), 530–549.
[https://doi.org/10.1080/19368623.2020.1685053]
-
Lockey, S., Gillespie, N., Holm, D., & Someh, I. A. (2021). A review of trust in Artificial Intelligence: Challenges, vulnerabilities and future directions. HICSS 2021: Proceedings of the 54th Hawaii International Conference on System Sciences (pp. 5463–5472). ScholarSpace.
[https://doi.org/10.24251/HICSS.2021.664]
-
Lu, L., Cai, R., & Gursoy, D. (2019). Developing and validating a service robot integration willingness scale. International Journal of Hospitality Management, 80, 36–51.
[https://doi.org/10.1016/j.ijhm.2019.01.005]
-
Man, S. S., Ding, M., Li, X., Chan, A. H. S., & Zhang, T. (2025). Acceptance of highly automated vehicles: The role of facilitating condition, technology anxiety, social influence and trust. International Journal of Human- Computer Interaction, 41(6), 3684–3695.
[https://doi.org/10.1080/10447318.2024.2316389]
-
Park, W. S., Oh, Y. S., & Cho, J. H. (2023). A study on the intention to use ChatGPT service using Unified Theory of Acceptance and Use of Technology (UTAUT): Focusing on the age group 20-40s. Korean Journal of Broadcasting and Telecommunication Studies, 37(5), 52–97.
[https://doi.org/10.22876/kab.2023.37.5.002]
-
Rheu, M., Shin, J. Y., Peng, W., & Huh-Yoo, J. (2021). Systematic review: Trust-building factors and implications for conversational agent design. International Journal of Human- Computer Interaction, 37(1), 81–96.
[https://doi.org/10.1080/10447318.2020.1807710]
-
Sledgianowski, D., & Kulviwat, S. (2009). Using social network sites: The effects of playfulness, critical mass and trust in a hedonic context. Journal of Computer Information Systems, 49(4), 74–83.
[https://doi.org/10.1080/08874417.2009.11645342]
-
Söllner, M., Hoffmann, A., & Leimeister, J. M. (2016). Why different trust relationships matter for information systems users. European Journal of Information Systems, 25(3), 274–287.
[https://doi.org/10.1057/ejis.2015.17]
-
Strzelecki, A. (2024). To use or not to use ChatGPT in higher education? A study of students’ acceptance and use of technology. Interactive Learning Environments, 32(9), 5142–5155.
[https://doi.org/10.1080/10494820.2023.2209881]
-
Suh, B., & Han, I. (2002). Effect of trust on customer acceptance of internet banking. Electronic Commerce Research and Applications, 1(3–4), 247–263.
[https://doi.org/10.1016/S1567-4223(02)00017-0]
-
Tung, F.-C., Chang, S.-C., & Chou, C.-M. (2008). An extension of trust and TAM model with IDT in the adoption of the electronic logistics information system in HIS in the medical industry. International Journal of Medical Informatics, 77(5), 324–335.
[https://doi.org/10.1016/j.ijmedinf.2007.06.006]
-
Tussyadiah, I. P., Zach, F. J., & Wang, J. (2020). Do travelers trust intelligent service robots? Annals of Tourism Research, 81, 102886.
[https://doi.org/10.1016/j.annals.2020.102886]
-
Upadhyay, N., Upadhyay, S., & Dwivedi, Y. K. (2022). Theorizing Artificial Intelligence acceptance and digital entrepreneurship model. International Journal of Entrepreneurial Behavior & Research, 28(5), 1138–1166.
[https://doi.org/10.1108/ijebr-01-2021-0052]
-
Venkatesh, V. (2022). Adoption and use of AI tools: A research agenda grounded in UTAUT. Annals of Operations Research, 308(1), 641–652.
[https://doi.org/10.1007/s10479-020-03918-9]
-
Venkatesh, V., & Davis, F. D. (1996). A model of the antecedents of perceived ease of use: Development and test. Decision sciences, 27(3), 451–481.
[https://doi.org/10.1111/j.1540-5915.1996.tb01822.x]
-
Venkatesh, V., & Davis, F. D. (2000). A theoretical extension of the technology acceptance model: Four longitudinal field studies. Management Science, 46(2), 186–204.
[https://doi.org/10.1287/mnsc.46.2.186.11926]
-
Venkatesh, V., Morris, M. G., Davis, G. B., & Davis, F. D. (2003). User acceptance of information technology: Toward a unified view. MIS Quarterly, 27(3), 425–478.
[https://doi.org/10.2307/30036540]
-
Venkatesh, V., Thong, J. Y., & Xu, X. (2012). Consumer acceptance and use of information technology: Extending the unified theory of acceptance and use of technology. MIS Quarterly, 36(1), 157–178.
[https://doi.org/10.2307/41410412]
-
Vitezić, V., & Perić, M. (2021). Artificial Intelligence acceptance in services: connecting with Generation Z. The Service Industries Journal, 41(13–14), 926–946.
[https://doi.org/10.1080/02642069.2021.1974406]
-
Wei, Z., Zhao, Z., & Zheng, Y. (2019). Following the majority: Social influence in trusting behavior. Frontiers in Neuroscience, 13, 89.
[https://doi.org/10.3389/fnins.2019.00089]
- Wu, J., & Liu, D. (2007). The effects of trust and enjoyment on intention to play online games. Journal of Electronic Commerce Research, 8(2), 128–140.
-
Wu, K., Zhao, Y., Zhu, Q., Tan, X., & Zheng, H. (2011). A meta-analysis of the impact of trust on technology acceptance model: Investigation of moderating influence of subject and context type. International Journal of Information Management, 31(6), 572–581.
[https://doi.org/10.1016/j.ijinfomgt.2011.03.004]
-
Yagoda, R. E., & Gillan, D. J. (2012). You want me to trust a robot? The development of a human- robot interaction trust scale. International Journal of Social Robotics, 4(3), 235–248.
[https://doi.org/10.1007/s12369-012-0144-0]
-
Zhang, T., Tao, D., Qu, X., Zhang, X., Zeng, J., Zhu, H., & Zhu, H. (2020). Automated vehicle acceptance in China: Social influence and initial trust are key determinants. Transportation Research Part C: Emerging Technologies, 112, 220–233.
[https://doi.org/10.1016/j.trc.2020.01.027]