
AI in the Public Eye: Decoding Perception of Generative AI Through Natural Language Processing
Copyright ⓒ 2025 by the Korean Society for Journalism and Communication Studies
Abstract
This study examined public perceptions of generative AI through the analysis of user comments on YouTube news videos regrading generative AI from three major South Korean broadcasting networks. Using structural topic modeling, 56,708 comments from 105 news videos were analyzed. Among nine distinct topics that emerged, the top three prevalent topics centered on labor market change, AI control concerns, and service automation benefits. Temporal analysis revealed evolving discourse patterns. Employment-related concerns peaked after the release of ChatGPT's but subsequently declined; three topics gained increasing prominence including human-centered AI development, control concerns, and educational applications. These findings offer theoretical and practical implications for individuals, organizations, and institutions adopting generative AI.
Keywords:
generative AI, public perception, natural language processing, structural topic modelingIn recent years, there has been a notable surge in the proliferation of commercially available large-language models powered by artificial intelligence (AI). Services such as OpenAI’s ChatGPT, Google’s Gemini, and Meta’s Llama have swiftly gained traction. With its remarkable ability to facilitate conversational interactions between systems and users, generative AI offers significant promise for both businesses and society (Obrenovic et al., 2025). The increasing prominence of generative AI, particularly with the introduction of ChatGPT, has drawn tremendous attention and interest (Buchholz, 2023; Hu, 2023; Ortiz, 2023). As a representative generative AI model, ChatGPT exhibits remarkable efficiency in user acquisition, achieving one million users within a mere five days. This rapid adoption outpaced Instagram (2.5 months) and Spotify (6 months) to reach the same user count (Teubner et al., 2023). In January 2023, two months after its launch, it was estimated to have reached 100 million monthly active users (Hu, 2023).
The application of generative AI in various sectors has become widespread (Čartolovni et al., 2023; Chiu, 2024; Kusiak, 2020). Generative AI is expected to play a pivotal role in the communication industry, such as generating written content for press releases; creating images and videos for advertising purposes; and producing synthetic multimedia encompassing text, images, audio, and videos (Bandi et al., 2023; Campbell et al., 2022). The profound impact of generative AI on communication and its expanding influence across diverse domains make it an imminent and integral technological presence.
The introduction of ChatGPT has brought about substantial societal reactions, such as bans in some public schools in the United States (Rosenblatt, 2023) and a complete ban in Italy (McCallum, 2023). Experts call for caution regarding unwarranted expectations and the fear that AI is harmful to society, as excessive expectations might lead to subsequent disappointment and unnecessary anxiety could hinder the adoption of this technology through overregulation (Cave, et al., 2019; Fast & Horvitz, 2017). Previous studies and reports have substantiated the potential negative consequences, which entail an early and deep understanding of the public perception towards generative AI.
The literature on generative AI has predominantly focused on its applications and adoption, with limited consideration given to understanding public responses regarding the acceptance of this technology (Kshetri et al., 2023; Nah et al., 2023). More importantly, despite the increasing use of generative AI in corporate communication, only a limited number of studies have explored its strategic application in building relationships with the public (Jiang et al., 2022; Men et al., 2023). Furthermore, there is a notable absence of quantitative research examining public perceptions of generative AI (Miyazaki et al., 2024).
To address this gap, this study examines the strategic management of generative AI in public communication contexts. Using natural language processing techniques, the study investigates how the public perceives generative AI through user comments on YouTube news content. Extensive research has demonstrated that media significantly influences individuals’ perceptions and attitudes on various societal topics (DeFleur & Ball-Rokeach, 1989); similarly, public perspectives on emerging technologies such as generative AI are substantially shaped by media framing. By analyzing viewers’ comments on YouTube news content, we gain insight into public perceptions and responses to generative AI’s emergence. These findings inform our recommendations for strategic approaches to adopting and implementing generative AI in both personal and organizational contexts, while providing guidance on how stakeholders should manage this technology to maximize benefits and mitigate concerns.
LITERATURE REVIEW
Generative AI: Current Status and Issues
Generative AI has emerged as a transformative force across various domains and has shown remarkable advancements in recent years. Generative AI is distinguished by its focus on creating contextually relevant content and expanding its applications from image synthesis to natural language generation. Termed “multimodal,” it can generate text, images, audio, video, and combinations of three or more dimensions (Bandi et al., 2023). OpenAI models such as ChatGPT and DALL-E exemplify the current state-of-the-art generative capabilities, proving proficiency in diverse creative tasks (Nah et al., 2023).
Generative AI holds significant promise for positive individual, organizational, and societal impacts (Obrenovic et al., 2025). At the individual level, it enhances creativity and productivity, benefiting creative professionals and those engaged in routine tasks (Agrawal, 2024). For example, graphic designers can leverage image generators for planning, concept development, detailed design, and refinement (Saadi & Yang, 2023). Public relations practitioners have also benefited from the generation of AI-written news releases. Currently, applications range from content generation (Nah et al., 2023) to the implementation of chatbots for customer interactions (Cheng & Jiang, 2020), streamlining operations, and enhancing management efficiency (Korzynski et al., 2023). Societally, generative AI contributes to advancements in healthcare, education, and manufacturing, fostering innovation and addressing societal challenges (Čartolovni et al., 2023; Chiu, 2024; Kusiak, 2020).
Despite its potential, generative AI presents ethical challenges. Primary concerns revolve around the ethical ramifications of misinformation and deep-fake generation, posing risks to the trust and authenticity of content (Li & Huang, 2020; Meskys et al., 2020). For instance, an advertising campaign for Dior J’adore used deepfake technology to swap Charlize Theron’s face with Rowan Atkinson’s (known as Mr. Bean), utilizing generative adversarial networks to substitute the attributes (e.g., face, voice, and gender) of a source with those of a target (Campbell et al., 2022). While the producers did not intend to mislead the public, highly sophisticated synthetic advertising can inevitably fool or trick the public.
As discussed, generative AI, owing to its inherent attributes, presents both advantages and disadvantages with potential impacts at the individual, organizational, and societal levels. However, public perception of this enormous influence remains unclear. To address this gap, this study aimed to provide a nuanced understanding of public perceptions toward generative AI, which is crucial for its responsible deployment.
Public Perception of Generative AI
Generative AI is considered a novel tool for improving decision-making capabilities in many areas of society. Along with its benefits, controversy and criticism of generative AI, followed by media narratives suggesting negative issues such as job security, human dignity, and ethical concerns (Brower, 2023; Liu, 2021), are establishing public perceptions towards this new technology. Fast and Horvitz’s (2017) analysis of the New York Times over the past 30 years showed that discussions around AI were consistently optimistic, whereas concerns about its negative consequences for jobs have grown recently.
The process of implementing a technology depends on its adoption and acceptance of that particular technology by individual users. Therefore, it is important to understand the public perceptions towards AI in its early phases (Binder et al., 2012). While the majority of Americans had been exposed to ChatGPT, only a few had experienced the technology themselves (Vogel, 2023). Public perception of AI is still evolving (Qi et al., 2024), and unlike earlier research on the adoption of generative AI, researchers are increasingly investigating the broader adoption process in a variety of public environments (Agrawal, 2024; Fichman & Kemerer, 1999; Lund et al., 2020; Zhu et al., 2006).
According to several studies, users have mixed views of AI. Araujo et al. (2020) examined the perceived usefulness of AI in diverse contexts and found that individuals were generally concerned about its risks and question its fairness and usefulness in society. In a survey conducted by the Pew Research Center, 38% of U.S. adults considered AI moderation on social media to be good for society, whereas 31% thought it was a bad idea, and approximately 30% were not sure. The respondents showed anxiety about the increasing use of AI in everyday life due to employment and privacy concerns; 81% of Americans worried about AI adoption in the workplace because they believed AI development would cause work layoffs (Kelly et al., 2023). Liang and Lee (2017) find that fear of AI and robots is associated with fear of unemployment. Similarly, from the interviews with Americans on AI, Zhang and Dafoe (2019) found that more individuals believe AI would eliminate more jobs than it will create (49% vs. 26%); however, more individuals support AI development than those who oppose it (41% vs. 22%). In a survey that explored individuals’ expectations and evaluations of AI, Brauner et al. (2023) find that participants associate AI with both positive and negative evaluations and with the expectation of certain developments. However, unlike many studies in which participants were worried about lower employment or lower wages, the participants were not concerned about development or risks; rather, they anticipated a positive effect on overall economic performance with the creation of new jobs.
Social media is frequently used to understand public perceptions of emerging technologies. Previous studies have found that, in general, most social media users are positive or neutral toward new technologies, whereas some problematic issues have been identified from text analyses (Bian et al., 2016; Kohl et al., 2018; Nuortimo et al., 2018). Twitter is the most popular medium for aggregating public narratives on generative AI and/or ChatGPT. Manikonda and Kambhampati (2018) analyzed AI-related tweets and found that sentiments expressed in AI conversations were generally more positive than typical Twitter sentiments. Haque et al. (2022) have analyzed the sentiments of over 10,000 tweets from early ChatGPT users and find that the majority showed positive emotional sentiments. Leiter et al. (2024) have conducted a sentiment analysis of more than 300,000 tweets about ChatGPT on Twitter and discover that ChatGPT is associated with positive sentiments and the emotion of joy. Taecharungroj (2023) use the latent Dirichlet allocation (LDA) topic modelling algorithm to analyze tweets and find that the tweets are mostly clustered around three major topics, that is, news, technology, and reactions, with five functional domains of ChatGPT: creative writing, essay composition, prompt generation, code scripting, and question answering.
Wang’s (2023) investigation of user perceptions of AI on social media indicates that familiarity and political ideology are negatively related to user perceptions, whereas algorithm acceptance is positively associated with user perceptions. In addition, trust in AI moderation mediated the relationship between the three characteristics and user perceptions, suggesting that the more familiar users were with AI moderation, the less likely they were to perceive it positively. Wang (2023) also emphasizes trust as a critical factor through which familiarity, political ideology, and algorithm acceptance are associated with perceptions of AI moderation. So et al. (2024) examine how the general public perceives generative AI in educational settings by analyzing YouTube user comments from news clips in South Korea. Analysis of the sentiments expressed in comments and discussion topics reveals that both optimistic and skeptical viewpoints coexist with divergent values and unsettled opinions during the generative AI introduction. In their study, while positive sentiments marginally outweighed negative sentiments, most public comments fell into the neutral category towards generative AI in education.
Understanding the determinants and barriers to AI adoption at the societal, individual, and personal levels is crucial. At the individual level, factors such as trust, security, cost, intrinsic motivation, social influence, utilitarian benefits, performance expectancy, and prior experience with AI influence AI adoption (Budeanu et al., 2023). According to Lukyanenko et al. (2022), trust is a critical psychological factor that lowers perceived uncertainty in AI development and deployment. Broadly defined as “the attitude that an agent will help achieve an individuals’ goals in a situation characterized by uncertainty and vulnerability” (Lee & See, 2004, p. 51), trust can be a critical factor in increasing public acceptance and use of AI (Choung et al., 2023; European Commission, 2020; WHO, 2018). Molina and Sundar (2022) find that users who had more experience with AI and less fear of technology were more likely to trust AI in the context of moderating hate speech and suicidal ideation. Similarly, trust in AI-based fake news detection systems is higher when users are younger and have higher overall trust in AI (Shin & Chan-Olmsted, 2023). Familiarity is an important factor in understanding public perceptions of AI. Kahan et al. (2008) argue that individuals are more likely to accept and support a particular technology when they become more familiar with or have more knowledge about it. A lack of knowledge about scientific topics results in public skepticism, which can be solved by providing sufficient information on those topics (Bauer et al., 2007; Sturgis & Allum, 2004).
The media has a significant impact on public perceptions by covering and framing selected features of emerging technologies (DeFleur & Ball-Rokeach, 1989). According to Johnson and Verdicchio (2017), inaccurate portrayals of AI in the media and the absence of humans result in anxiety among the general population. Although media discourse regarding AI has become sophisticated, the quality of information and content still needs improvement (Ouchchy et al., 2020). Furthermore, as Ittefaq et al. (2025) pointed out, the mainstream media coverage tend to be critical, suggesting potential risks such as job losses and compromised privacy. Hence, scholars argue for a balanced framing of AI in the media with even-handed media reporting, including both negative and positive social implications (Sartori & Bocca, 2023).
Overall, the collective body of research reveals the complex nature of public perceptions of generative AI. Although some optimistic viewpoints suggest the technology’s potential for development, numerous studies have highlighted challenges. Hence, some researchers maintain that AI is still a “black box” of which neither opportunities nor risks have been adequately addressed (Brauner et al., 2023). Therefore, it is essential to examine the intricate dynamics of adopting emerging technologies, particularly the issues that can lead to biased and irrational control beliefs in the public perception of AI.
AI Adoption and Diffusion of Innovation
The well-known theory of the Diffusion of Innovations has been used as a theoretical framework in many studies to describe, predict, and explain the innovation adoption process (Atkin et al., 2015; Dearing, 2021; Rogers, 2003). Diffusion is the process by which innovation is adopted or rejected over time among the members of a society (Rogers, 2003). Diffusion research has identified five attributes that explain the variance in adoption: relative advantage, complexity, compatibility, trialability, and observability (Rogers, 2003). Relative advantage, one of the strongest predictors of adoption, is the extent to which an innovation is perceived as superior to its predecessor or existing alternatives; the bigger the perceived benefits of innovation, the more likely individuals are to adopt it (Atkin et al., 2015; Rogers, 2003). Complexity refers to the degree to which an innovation is perceived as easy or difficult to understand and use. New technologies that are easier to use are adopted faster than innovations that are more difficult to learn. Compatibility refers to how well an innovation aligns with current processes, practices, and the needs of potential adopters; when an innovation is considered to be more compatible with the existing work style or situation, it is more likely to be adopted. Observability refers to the visibility of the outcomes or results of adoption to others. According to Rogers (2003), innovations with relatively high observability, such as cellphones, diffuse more rapidly than personal computers. Finally, trialability refers to the degree to which potential adopters can experiment with an innovation on a limited basis. Having the opportunity to innovate can help reduce uncertainty. Generally, innovations that can be attempted are readily adopted. While all five characteristics are relevant, the first three (compatibility, relative advantage, and complexity) are widely found to explain and predict innovation diffusion in several studies (Agrawal, 2024). Rogers (2003) maintains that the subjective evaluation of an innovation is more critical than its objective attributes; individuals’ personal experiences and perceptions affect their attitudes toward innovation and subsequent adoption behaviors.
Rogers (2003) identifies five categories of adopters: innovators, the first individuals to adopt an innovation; early adopters, the trendsetters who do not rush to adopt an innovation but evaluate and adopt the innovation only after determining its value; early majority, those who follow the leadership of the early adopters once the value of the innovation has been established; late majority, those who are naturally skeptical toward an innovation and adopt the innovation later than average; and laggards, traditionalists who actively resist the innovation. Using these adopter categories as demographic variables for a population, it may be possible to examine how different adopter categories view emerging topics differently (Lund et al., 2020).
Lund et al. (2020) examine librarians’ perceptions of AI based on the diffusion of innovation models. Although some respondents were concerned about AI replacing their job roles, this was a minority perception. Academic librarians generally have a favorable opinion of AI and the integration of new technology into their work. This result aligns well with the findings for the adopter categories, indicating that most participants fell within the categories of either early adopters or the early majority.
In the context of AI adoption in the workplace, Xu et al. (2023) have examined employees’ attitudes toward AI adoption within the theoretical framework of diffusion and find that relative advantage, compatibility, and observability correlated with more positive attitudes, whereas complexity and trialability had no significant influence. Furthermore, Xu et al. (2023) attempt to extend the theory by incorporating the threat of technologies, individuals’ perceptions of their susceptibility to, and the resulting severity of technologies as additional attributes. In the study, employees considered AI a threat that might impact their career prospects, job security, and employment opportunities. Consequently, considering innovation adoption as a dynamic process, Xu et al. (2023) argue for the need to understand beyond AI attributes that employees’ accumulated emotions and cognitions should be considered. The study also recommends AI designs and adoption campaigns that emphasize the benefits of AI in improving employees’ job performance and efficiency.
According to Dearing (2021), several factors affect the rate at which AI chatbots diffuse into and reach the general population. For example, how they are framed as innovations, how they are perceived by potential adopters, and the social structural positions of early adopters are crucial to their adoption (Dearing & Cox, 2018). Consequently, Dearing (2021) calls for the use of behavioral nudges, encouragement, and social support to facilitate the diffusion of this new technology.
Research Questions
This comprehensive body of research highlights the intricate landscape of public perception and acceptance of generative AI. While some optimistic perspectives emphasize the developmental potential of technology, many studies underscore the existence of challenges. Moreover, some researchers contend that AI remains a “black box” with inadequately addressed opportunities and risks (Brauner et al., 2023). Therefore, it is imperative to scrutinize the intrinsic dynamics of individual adoption of emerging technologies, particularly in their early stages. Besides, a limited number of study examined user discourses in response to news reports regarding generative AI; a majority of the studies on public perception employed survey methodologies, lacking nuanced interpretation of sentiment. To gain insight into public perceptions during the nascent phase, the following research questions were posed:
- RQ1. What are the dominant themes emerging from public discourse in comments on generative AI news?
- RQ2. How have these thematic patterns shifted over time in public discussions of generative AI?
METHODS
Data Collection
This study investigates public perception of generative AI news as presented by major broadcasters. The initial data collection involved selecting news clips available on YouTube. First, to ensure the validity and credibility of the news clips, we focused on the video clips from three major South Korean broadcasting networks (KBS, MBC, and SBS) for sampling (So et al., 2024). In each broadcasting network’s YouTube channel, we collected news videos with the search keyword “generative AI.” We also added “ChatGPT” as a search term, given the increased attention to generative AI following the public release of ChatGPT 3.5 in November 2022 (Teubner et al., 2023). The initial search yielded 302 videos. We then filtered for videos with a minimum of 20 comments, resulting in 105 videos spanning from January 2022 to January 2025 for analysis. To analyze public perceptions of generative AI news videos on YouTube, we extracted public comments from each selected news video. All comments corresponding to the selected news videos were obtained through the YouTube API using the tuber wrapper in R (Sood, 2019). This process yielded 56,708 comments for analysis (see Table 1).
Text Preparation
Prior to implementing topic modeling, we conducted several text processing steps on the YouTube comments. First, we eliminated all numbers, punctuation marks, symbols, and URLs from the text (Sproat et al., 2001). Word tokenization was then applied to parse the text into individual lexical units (Vijayarani & Janani, 2016). Finally, we removed stopwords, including both common linguistic elements (e.g., “that,” “is,” and “about”) and domain-specific terms (e.g., “AI” and “artificial intelligence”). We removed domain-specific terms from the analysis because their ubiquity across the corpus limited their discriminatory power in topic identification (Alshanik et al., 2020).
Topic Modeling
Topic modeling represents a text mining approach that systematically categorizes textual content into meaningful thematic clusters, termed topics, through automated procedures (Mohr & Bogdanov, 2013). This computational method minimizes human intervention, offering a more inductive approach compared to conventional text analysis methods in social sciences. While various topic modeling approaches exist, including Latent Dirichlet Allocation (LDA) (Blei et al., 2003) and Correlated Topic Models (CTM) (Blei & Lafferty, 2007), this study employs the Structural Topic Model (STM). STM extends beyond these foundational probabilistic frameworks through its distinctive capability to incorporate document metadata, enabling researchers to examine the relationship between document attributes and topical composition (Roberts et al., 2016). The model’s ability to process temporal information proves particularly advantageous for examining thematic developments across time periods (Roberts et al., 2016). Our investigation leverages this temporal analysis capability to examine the evolution of discourse patterns in YouTube comments chronologically.
We implemented our topic identification process according to the STM framework developed by Roberts et al. (2019):
Step 1. Data Preparation After preprocessing the text, we generated a document-term matrix (DTM) to structure our dataset, where individual comments constituted rows and unique corpus words formed columns. We utilized the textProcessor function in R to incorporate the complete vocabulary of our corpus. The resulting processed dataset encompassed 56,708 comments containing 364,660 unique words.
Step 2. Topic Number Selection Prior to conducting topic modeling, researchers must determine the number of topics by considering statistical, theoretical, and practical reasons (Blei et al., 2003). From a statistical standpoint, topic modeling operates as a generative probabilistic model for a corpus. The underlying concept posits that documents are depicted as random mixtures of latent topics, each characterized by a distribution of words (Blei et al., 2003). Within this framework, when a researcher selects a specific number of topics, a distribution is automatically assigned. From a theoretical perspective, predetermined categories aid in the exploration of novel and useful categorization schemes for measuring relevant quantities in large text collections (Grimmer & Stewart, 2013). On a practical level, the interpretability of latent topics takes precedence over maximizing the model fit (Chang et al., 2009).
Following established methodological protocols (e.g., Chung et al., 2022), we employed the searchK function from R to systematically evaluate model performance across different topic configurations. Our experimental framework examined K values ranging from 5 to 20, with sequential single-unit increments. The evaluation protocol prioritized semantic coherence metrics, which quantify the co-occurrence frequency of high-probability terms within each topic. This statistical evaluation indicated that models containing eight to ten topics demonstrated optimal semantic coherence values.
Relying solely on statistical approaches for determining optimal topic numbers presents recognized methodological limitations. As DiMaggio et al. (2013) emphasize, computational metrics may yield misleading results, as models frequently segregate statistical noise into distinct topics, thereby artificially elevating the coherence metrics of the remaining thematic clusters. To address this limitation, we complemented our quantitative analysis with a qualitative evaluation of “semantic interpretability” (DiMaggio, 2015, p. 3). A comparative examination of models with eight, nine, and ten topics revealed that the ninetopic configuration provided the most coherent and interpretable thematic framework.
Step 3. Structural Topic Modeling Using the STM framework, we first identified the dominant keywords and computed topic distribution patterns across the corpus. This methodology facilitates the examination of relationships between document metadata and topical distributions (Chung et al., 2022). To investigate temporal dynamics, we analyzed variations in topic prevalence throughout our study period (2021-2024). The temporal analysis incorporated year as a covariate and utilized a B-spline model with four degrees of freedom, allowing us to capture non-linear relationships within the data (Perperoglou et al., 2019).
RESULTS
Prevalent Topics Discussed on YouTube
To address RQ1, we applied STM analysis to the preprocessed corpus. Through this methodological approach, we identified nine distinct, interpretable, and mutually exclusive topics (Chang et al., 2009). The emergent topics encompassed a spectrum of public perceptions regarding generative AI, ranging from optimistic expectations and expressions of amazement to discussions about appropriate societal responses to the technology.
Analysis of public discourse revealed nine distinct themes in discussions about generative AI. HUMAN-CENTERED GENERATIVE AI (Topic 1, 2.79%) emerged as the least discussed theme, emphasizing the importance of human agency in generative AI development through keywords like “human,” “empower,” and “priority.” Public discourse also reflected significant interest in GENERATIVE AI ALGORITHM BIAS (Topic 2, 11.53%), characterized by technical terms such as “computer” and “programmer,”while acknowledging concerns about “fallacy” and “bias.”
Societal anxieties manifested prominently in GENERATIVE AI CONTROL CONCERNS (Topic 3, 16.80%), where references to science fiction narratives like “Terminator,” “Skynet,” and “Matrix” intertwined with concrete fears about “nuclear weapon” and “virus.” Technical discussions coalesced around GENERATIVE AI ARCHITECTURE (Topic 4, 6.95%), focusing on infrastructure components through terms like “bigdata,” “NVIDIA,” “hardware,” “semiconductor,” and “software.”
Educational implications emerged through GENERATIVE AI IN EDUCATION (Topic 5, 6.86%), exploring generative AI’s role in learning environments via keywords such as “digital,” “textbook,” and “teacher.” The most prominent theme, LABOR MARKET CHANGE (Topic 6, 20.70%), captured broader workforce implications through terms like “job,” “employee,” and “unemployment.”
Technological advancement discussions appeared in AI DRIVEN TECHNOLOGY (Topic 7, 9.72%), featuring references to “programming,” “deep-learning,” and industry figures like “Elon-Musk.” SERVICE AUTOMATION BENEFITS (Topic 8, 14.07%) explored efficiency gains through keywords such as “automation,” “benefit,” and “labor-cost.” The last discourse addressed PROFESSIONAL DISPLACEMENT (Topic 9, 10.88%), highlighting concerns about professional displacement across various fields such as “officer,” “lawyer,” “prosecutor,” and “judge.”
Topic Evolution
To address Research Question 2, we examined temporal patterns in topic prevalence since the emergence of generative AI as a public concern. While most topics maintained relatively stable proportions since 2022, our analysis revealed two notable shifts in topic distribution.
First, Topic 6 (LABOR MARKET CHANGE), the most prevalent theme overall, reached its peak in early 2023 following the release of ChatGPT, but subsequently showed a gradual decline in discussion frequency. Similarly, Topic 9 (PROFESSIONAL DISPLACEMENT) exhibited a decreasing trend in prevalence over time.
Conversely, several topics demonstrated increasing prominence throughout the study period. Topic 1 (HUMAN-CENTERED GENERATIVE AI), despite having the smallest overall proportion in the corpus, showed consistent growth in prevalence. Topic 3 (GENERATIVE AI CONTROL CONCERNS), which captured anxieties about AI dominance through science fiction references, also gained increasing attention. Additionally, Topic 5 (GENERATIVE AI IN EDUCATION), focusing on the integration of generative AI in educational contexts, showed steady growth in discussion frequency over time.
Topic Prevalence among Broadcasting Networks
During data collection, we observed a notable disparity in user engagement across the three major broadcasting networks. While KBS and MBC garnered relatively similar levels of engagement (25 videos with 6,625 comments and 31 videos with 7,260 comments, respectively, as shown in Table 1), SBS demonstrated substantially higher engagement with 42,823 comments from 49 videos. Although not initially posed as a research question, we conducted an additional analysis to investigate the factors driving higher user engagement with SBS’s generative AI news clips by comparing topic prevalence between SBS and the other two networks.
Figure 2 illustrates the marginal effects of topic proportion differences across networks. The points represent the mean estimated difference between SBS and the other two networks, while the bars indicate 95% confidence intervals. The distance between each point estimate and the dotted line (representing zero effect) indicates the magnitude of topic prevalence variation.
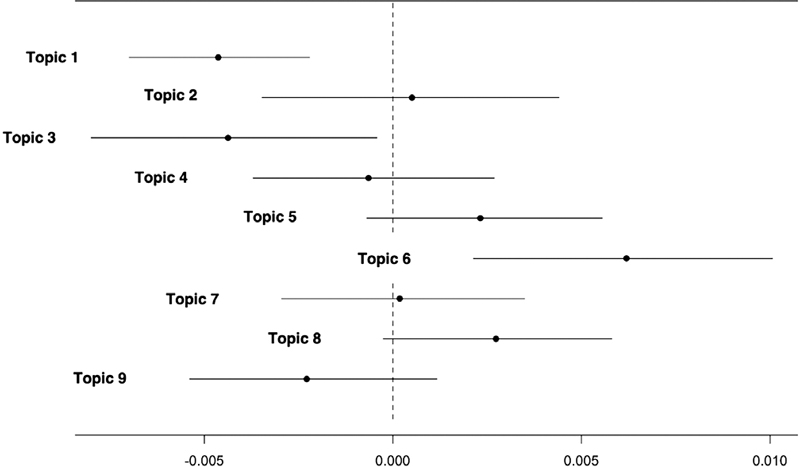
Topic Prevalence Difference between SBS and Other TwoNote. The figure displays the estimated differences in topic proportions between SBS and the other two broadcasting networks (KBS and MBC), showing point estimates and their corresponding 95% confidence intervals. Positive point estimates indicate topics that were more prevalent in SBS comments, while negative estimates indicate topics that were less prevalent in SBS comments relative to the other networks.
Analysis revealed that comments on SBS content exhibited higher prevalence of Topics 2, 5, 6, 7, and 8, while showing lower prevalence of Topics 1, 3, and 9. Notably, Topic 6, which represented the largest proportion across the entire corpus, demonstrated significantly higher prevalence in SBS comments.
DISCUSSION
As one of the initial empirical investigations into public perceptions of generative AI through YouTube news comments, this study employed structural topic modeling to analyze public perception. Our analysis revealed three key findings that illuminate public responses to contemporary generative AI developments.
The identified topics encompassed both advantages and potential challenges of generative AI implementation. Primary concerns centered on employment implications (Topics 6 and 9), algorithmic bias (Topic 2), and AI controllability (Topic 3). Positive perspectives emerged through discussions of service automation benefits (Topic 8), while neutral discourse focused on technical aspects such as AI architecture (Topic 4) and technological advancement (Topic 7). Forwardlooking discussions emphasized the necessity of human-centric AI development (Topic 1) and the integration of AI in educational contexts (Topic 5).
The temporal analysis of topic prevalence revealed distinct evolutionary patterns in public discourse. While initial concerns about job displacement peaked following generative AI's public release (e.g., release of ChatGPT), these discussions have subsequently declined. Conversely, three themes have gained increasing prominence: the importance of human-centric AI development (Topic 1), concerns about AI control over human society (Topic 3), and the integration of AI in educational contexts (Topic 5).
Our analysis of engagement patterns revealed that news content addressing job-related implications, digital education, and service automation benefits generated higher levels of public interaction. That is, these topics prompted more extensive commenting behavior. Together, our findings on thematic content, temporal trends, and engagement patterns offer valuable insights for both theoretical frameworks and practical applications, which we examine in detail below.
Theoretical implications
Our findings align with previous research on public perceptions of generative AI’s usefulness, risks, and challenges (Araujo et al., 2020; Brauner et al., 2023). Most prominently, our analysis revealed that concerns about labor market transformation emerged as the dominant theme. This corresponds with prior studies documenting public anxiety about workplace AI adoption, particularly regarding potential job displacement (Kelly et al., 2023). Furthermore, research has established a significant correlation between AI-related fears and unemployment concerns (Liang & Lee, 2017). The keywords identified through our topic modeling —“data,” “job,” “employee,” “copyright,” “computer-scientist,” “unemployment,” and “position”— suggest widespread public apprehension about job security, specifically regarding potential displacement by data professionals and computer scientists.
In relation to labor market transformation concerns, Topic 9 identified specific professions perceived as vulnerable to generative AI displacement, particularly legal occupations including “lawyer,” “prosecutor,” and “judge.” This finding reflects growing concerns about the potential automation of legal professions, given that many legal processes can be standardized and routinely executed by AI systems (Martinho, 2025).
Topics 2 and 3 revealed negative perceptions regarding algorithmic bias and AI controllability, highlighting public concerns about potential individual and societal harm. While early research on AI algorithms suggested that user acceptance increases with familiarity (Wang, 2023), recent studies have demonstrated that perceptions of generative AI—whether positive or negative— are more closely tied to AI literacy and trust in the technology (Schiavo et al., 2024; Shin, 2022). These findings suggest that enhancing public AI literacy may be crucial for addressing anxieties about generative AI.
Analysis of Topic 8 revealed positive perceptions toward generative AI, particularly regarding anticipated service automation benefits. The multi-modal capabilities of generative AI position it to play a pivotal role in content creation, encompassing written materials, images, videos, and integrated multimedia content (Bandi et al., 2023; Campbell et al., 2022). At the individual level, service automation is expected to enhance creativity and productivity, benefiting both creative professionals and workers engaged in routine tasks (Agrawal, 2024). At the organizational level, the implementation of generative AI-based chatbots for customer interactions, operational streamlining, and internal communication promises improved management efficiency (Cheng & Jiang, 2020; Korzynski et al., 2023; Shim et al., 2024). From a broader societal perspective, generative AI contributes to advancements in healthcare, education, and manufacturing sectors, fostering innovation and addressing significant societal challenges (Čartolovni et al., 2023; Chiu, 2024; Kusiak, 2020).
The simultaneous presence of positive and negative perceptions toward generative AI illustrates the nuanced relationship between technological understanding and public acceptance. Although technological familiarity typically enhances acceptance, research has shown that scientific knowledge can increase public skepticism, necessitating thorough information provision to address concerns (Bauer et al., 2007; Sturgis & Allum, 2004). The current ambivalence in public perception may thus reflect insufficient AI literacy resulting from inadequate access to comprehensive information about generative AI.
The temporal analysis of topics revealed two distinct patterns: increasing and decreasing prevalence. Notably, Topic 1 demonstrated consistent growth in prevalence despite its relatively small overall proportion. While most topics addressed either positive or negative aspects of generative AI, this topic advocated for a proactive, human-centered approach to technological implementation. This alignment suggests a pathway toward addressing previously identified concerns about ethical, credible, and authentic AI development (Li & Huang, 2020; Meskys et al., 2020).
Drawing on Rogers’ (2003) technology acceptance framework, particularly the concept of relative advantage—perceived superiority over existing alternatives—helps explain the increasing prevalence of human-centered generative AI discourse. While public recognition of AI’s superiority suggests acceptance based on perceived relative advantage, the growing topic prevalence indicates an emerging understanding of the necessity for human-directed application of this technology. Similarly, discussions about generative AI in education showed an upward trend. This increasing proportion reflects public perception of compatibility, which Rogers (2003) defines as the alignment between an innovation and existing processes, practices, and adopter needs. The growth in educational discourse suggests public recognition that generative AI could be effectively integrated into current educational frameworks.
The growing prevalence of Topic 3 revealed increasing concerns about generative AI control, influenced by science fiction narratives such as “Terminator,” “Matrix,” and “I, Robot.” This upward trend can be understood through Rogers’ (2003) concept of observability—the degree to which innovation outcomes are visible to potential adopters. While generative AI’s direct effects may lack the immediate visibility of technologies like mobile phones (Rogers, 2003), the public has vicariously observed potential consequences through science fiction media, leading to heightened concerns about AI control.
Conversely, Topics 6 and 9, which addressed job transformation and displacement, exhibited decreasing prevalence over time. While previous research has demonstrated that AI discussions maintained general optimism over the past three decades, concerns about employment consequences have intensified in recent years (Fast & Horvitz, 2017). Our findings align with this trajectory, as negative discourse regarding potential job loss dominated the initial period following generative AI’s release. However, the gradual decline in these topics suggests that the actual labor market impact of generative AI has proven less severe than initially anticipated after the technology’s public deployment.
Lastly, our analysis of topic proportion differences across broadcasting networks revealed significant variations. Topic 6 was substantially more prevalent in comments on SBS news clips. Given that SBS garnered more than three times the comment volume of MBC and KBS combined, it is reasonable that the most prevalent topic identified in RQ1 emerged predominantly in SBS content. However, it is noteworthy that Topic 3—the second most prevalent topic overall—was among the least discussed topics in SBS comments, while featuring prominently in MBC and KBS discussions. This divergence suggests that users engaging with generative AI news on different broadcasting networks may develop distinct perceptions based on varying content framing approaches. The differential prominence of Topic 3 indicates that MBC and KBS may have presented generative AI with greater emphasis on potential control risks and societal concerns, while SBS coverage potentially focused more on practical implications like job market impacts. This finding aligns with media framing theory, which posits that news organizations’ presentation of issues influences audience perception and interpretation (Entman, 1993; Scheufele, 1999), suggesting that broadcasting networks may play a significant role in shaping public discourse about emerging technologies through their distinctive framing choices.
Practical Implications
Our findings offer several actionable insights for users and organizations navigating the implementation of generative AI technologies. These implications address key concerns identified in public discourse. Below, we outline three primary practical implications derived from our analysis.
First, the findings underscore the necessity of communication strategies geared towards mitigating public fear and anxiety associated with the adoption of generative AI. Organizations offering generative AI services must articulate the advantages of harnessing collective intelligence through collaboration between generative AI and human effort. A potential key message should emphasize the superior outcomes achievable through collective efforts, surpassing the capabilities of individuals or machines working in isolation.
Second, the prevalence of employment-related concerns suggests that organizations implementing generative AI should develop comprehensive change management and internal communication strategies. Organizations are advised to provide transparency regarding how generative AI will complement rather than replace human workers, to offer reskilling opportunities, and to clearly articulate the intended role of AI within their operational frameworks.
Third, the increasing prevalence of humancentered AI discourse indicates growing public recognition of the necessity for human-centered AI development and governance. Enhanced discourse on human-AI collaboration is needed at both individual life management and organizational levels to align with evolving public expectations and foster greater acceptance and trust in AI technologies. The findings suggests that addressing public apprehension towards generative AI requires positioning AI-supported services as efficient and timesaving tools (Cheng & Jiang, 2020).
Finally, the concerns about AI control revealed through science fiction references suggest that public communication initiatives about generative AI should directly address misconceptions while acknowledging legitimate ethical considerations. Technology companies and educational institutions should develop AI literacy programs that balance realistic capabilities with responsible development frameworks to mitigate unfounded fears while promoting informed technological citizenship.
Limitations and Future Directions
The current study presents some limitations that indicate avenues for future research. Our analysis focused exclusively on public comments about generative AI news on YouTube using structural topic modeling. While this approach enabled us to identify meaningful thematic patterns in public discourse, it cannot establish the direct consequences of these discussions for individual attitudes or societal acceptance. Thus, future research should examine the relationship between public discourse and measurable outcomes such as trust, acceptance, and willingness to use toward generative AI technologies.
Second, the study focused on public responses regarding generative AI news; consequently, the findings fall short of delineating the origins and drivers of positive and negative sentiments. Alternatively, a traditional statistical approach may be warranted to explore the deeper relationships among public perception, attitude, and behavior towards the acceptance of generative AI. Future research could scrutinize the news agenda surrounding generative AI to ascertain prevalent new agendas and public responses.
Additionally, more diverse methodological approaches could significantly extend our understanding of generative AI discourse and its effects. Longitudinal studies might track how exposure to specific narrative patterns influences individual perceptions and acceptance over time, revealing causal mechanisms that crosssectional analyses cannot capture. Experimental research could systematically manipulate news framing to identify how different presentation approaches affect public comprehension, risk perception, and acceptance of generative AI. Such experimental designs might compare technological determinism frames against human agency frames, or benefit-oriented versus riskoriented presentations, to determine optimal communication strategies for fostering informed public engagement.
Disclosure Statement
No potential conflict of interest was reported by the author.
References
-
Agrawal, K. (2024). Towards adoption of generative AI in organizational settings. Journal of Computer Information Systems, 64(5), 636–651.
[https://doi.org/10.1080/08874417.2023.2240744]
-
Alshanik, F., Apon, A., Herzog, A., Safro, I., & Sybrandt, J. (2020). Accelerating text mining using domain-specific stop word lists. Proceedings of 2020 IEEE International Conference on Big Data (pp. 2639–2648). IEEE.
[https://doi.org/10.1109/BigData50022.2020.9378226]
-
Araujo, T., Helberger, N., Kruikemeier, S., & Vreese, C. H. de (2020). In AI we trust? Perceptions about automated decision-making by artificial intelligence. AI & SOCIETY, 35(3), 611–623.
[https://doi.org/10.1007/s00146-019-00931-w]
-
Atkin, D. J., Hunt, D. S., & Lin, C. A. (2015). Diffusion theory in the new media environment: Toward an integrated technology adoption model. Mass Communication & Society, 18(5), 623–650.
[https://doi.org/10.1080/15205436.2015.1066014]
-
Bandi, A., Adapa, P. V. S. R., & Kuchi, Y. E. V. P. K. (2023). The power of generative AI: A review of requirements, models, input-output formats, evaluation metrics, and challenges. Future Internet, 15(8), 260.
[https://doi.org/10.3390/fi15080260]
-
Bauer, M. W., Allum, N., & Miller, S. (2007). What can we learn from 25 years of PUS survey research? Liberating and expanding the agenda. Public Understanding of Science, 16(1), 79–95.
[https://doi.org/10.1177/0963662506071287]
-
Bian, J., Yoshigoe, K., Hicks, A., Yuan, J., He, Z., Xie, M., Guo, Y., Prosperi, M., Salloum, R., & Modave, F. (2016). Mining Twitter to assess the public perception of the “Internet of Things”. PLoS ONE, 11(7), e0158450.
[https://doi.org/10.1371/journal.pone.0158450]
-
Binder, A. R., Cacciatore, M. A., Scheufele, D. A., Shaw, B. R., & Corley, E. A. (2012). Measuring risk/benefit perceptions of emerging technologies and their potential impact on communication of public opinion toward science. Public Understanding of Science, 21(7), 830–847.
[https://doi.org/10.1177/0963662510390159]
-
Blei, D. M., & Lafferty, J. D. (2007). A correlated topic model of science. The Annals of Applied Statistics, 1(1), 17–35.
[https://doi.org/10.1214/07-AOAS114]
- Blei, D. M., Ng, A. Y., & Jordan, M. I. (2003). Latent dirichlet allocation. Journal of Machine Learning Research, 3(1), 993–1022.
-
Brauner, P., Hick, A., Philipsen, R., & Ziefle, M. (2023). What does the public think about artificial intelligence?—A criticality map to understand bias in the public perception of AI. Frontier of Computer Science, 5, 1113903.
[https://doi.org/10.3389/fcomp.2023.1113903]
- Brower, T. (2023, May 5). People fear being replaced by AI and ChatGPT: 3 ways to lead well amidst anxiety. Forbes. https://www.forbes.com/sites/tracybrower/2023/03/05/people-fear-being-replaced-by-ai-and-chatgpt-3-ways-to-lead-well-amidst-anxiety
- Buchholz, K. (2023, July 7). Chart: Threads shoots past one million user mark at lightning speed. Statista. https://www.statista.com/chart/29174/time-to-one-million-users
- Budeanu, A. M., Ţurcanu, D., & Rosner, D. (2023). European perceptions of artificial intelligence and their social variability. An exploratory study. Proceedings of the 24th International Conference on Control Systems and Computer Science (pp. 436–443). IEEE.
-
Campbell, C., Plangger, K., Sands, S., & Kietzmann, J. (2022). Preparing for an era of deepfakes and AI-generated ads: A framework for understanding responses to manipulated advertising. Journal of Advertising, 51(1), 22–38.
[https://doi.org/10.1080/00913367.2021.1909515]
-
Čartolovni, A., Malešević, A., & Poslon, L. (2023). Critical analysis of the AI impact on the patient-physician relationship: A multi-stakeholder qualitative study. Digital Health, 9.
[https://doi.org/10.1177/20552076231220833]
-
Cave, S., Coughlan, K., & Dihal, K. (2019). “Scary Robots” examining public responses to AI. Proceedings of the 2019 AAAI/ACM Conference on AI, Ethics, and Society (pp. 331–337). ACM.
[https://doi.org/10.1145/3306618.3314232]
- Chang, J., Gerrish, S., Wang, C., Boyd-graber, J., & Blei, D. (2009). Reading tea leaves: How humans interpret topic models. NIPS ’09: Proceedings of the 23rd International Conference on Neural Information Processing Systems, (pp. 288–296). ACM.
-
Cheng, Y., & Jiang, H. (2020). How do AI-driven chatbots impact user experience? Examining gratifications, perceived privacy risk, satisfaction, loyalty, and continued use. Journal of Broadcasting & Electronic Media, 64(4), 592–614.
[https://doi.org/10.1080/08838151.2020.1834296]
-
Chiu, T. K. F. (2024). The impact of Generative AI (GenAI) on practices, policies and research direction in education: A case of ChatGPT and Midjourney. Interactive Learning Environments, 32(10), 6187–6203.
[https://doi.org/10.1080/10494820.2023.2253861]
- Choung, H., David, P., & Ross, A. (2023). Trust in AI and its role in the acceptance of AI technologies. International Journal of Human-Computer Interaction, 39(9), 1727–1739.
-
Chung, G., Rodriguez, M., Lanier, P., & Gibbs, D. (2022). Text-mining open-ended survey responses using structural topic modeling: A practical demonstration to understand parents’ coping methods during the COVID-19 pandemic in Singapore. Journal of Technology in Human Services, 40(4), 296–318.
[https://doi.org/10.1080/15228835.2022.2036301]
-
Dearing, J. W. (2021). What will affect the diffusion of AI agents? Human-Machine Communication, 3, 83–89.
[https://doi.org/10.30658/hmc.3.6]
- Dearing, J. W., & Cox, J. G. (2018). Diffusion of innovations theory, principles, and practice. Health Affairs, 37(2), 183–190.
- DeFleur, M., & Ball-Rokeach, S. (1989). Media system dependency theory. In M. DeFleur & S. Ball-Rokeach (Eds.), Theories of mass communication (pp. 292–327). Longman.
-
DiMaggio, P. (2015). Adapting computational text analysis to social science (and vice versa). Big Data & Society, 2(2).
[https://doi.org/10.1177/2053951715602908]
-
DiMaggio, P., Nag, M., & Blei, D. (2013). Exploiting affinities between topic modeling and the sociological perspective on culture: Application to newspaper coverage of U.S. government arts funding. Poetics, 41(6), 570–606.
[https://doi.org/10.1016/j.poetic.2013.08.004]
- Entman, R. M. (1993). Framing: Toward clarification of a fractured paradigm. Journal of Communication, 43(4), 51–58.
- European Commission. (2020). White paper on Artificial Intelligence: A European approach to excellence and trust. https://commission.europa.eu/publications/white-paper-artificial-intelligence-european-approach-excellence-and-trust_en
-
Fast, E., & Horvitz, E. (2017). Long-term trends in the public perception of artificial intelligence. AAAI-17: Proceedings of the 31st AAAI Conference on Artificial Intelligence (pp. 963– 969). AAAI.
[https://doi.org/10.1609/aaai.v31i1.10635]
-
Fichman, R. G., & Kemerer, C. (1999). The illusory diffusion of innovation: An examination of assimilation gaps. Information System Research, 10(3), 255–275.
[https://doi.org/10.1287/isre.10.3.255]
-
Grimmer, J., & Stewart, B. M. (2013). Text as data: The promise and pitfalls of automatic content analysis methods for political texts. Political Analysis, 21(3), 267–297.
[https://doi.org/10.1093/pan/mps028]
-
Haque, M. U., Dharmadasa, I., Sworna, Z. T., Rajapakse, R. N., & Ahmad, H. (2022). “I think this is the most disruptive technology”: Exploring sentiments of ChatGPT early adopters using Twitter data. ArXiv.
[https://doi.org/10.48550/arXiv.2212.05856]
- Hu, K. (2023, February 3). ChatGPT sets record for fastest-growing user base - analyst note. Reuters. https://www.reuters.com/technology/chatgpt-sets-record-fastest-growing-user-base-analyst-note-2023-02-01/
-
Ittefaq, M., Zain, A., Arif, R., Ala-Uddin, M., Ahmad, T., & Iqbal, A. (2025). Global news media coverage of Artificial Intelligence (AI): A comparative analysis of frames, sentiments, and trends across 12 countries. Telematics and Informatics, 96, 102223.
[https://doi.org/10.1016/j.tele.2024.102223]
-
Jiang, H., Cheng, Y., Yang, J., & Gao, S. (2022). AI-powered chatbot communication with customers: Dialogic interactions, satisfaction, engagement, and customer behavior. Computers in Human Behavior, 134, 107329.
[https://doi.org/10.1016/j.chb.2022.107329]
-
Johnson, D. G., & Verdicchio, M. (2017). AI anxiety. Journal of American Society Information Science, 68(9), 2267–2270.
[https://doi.org/10.1002/asi.23867]
-
Kahan, D. M., Braman, D., Slovic, P., Gastil, J., & Cohen, G. L. (2008). The future of nanotechnology risk perceptions: An experimental investigation of two hypotheses (Harvard Law School Program on Risk Regulation Research Paper No. 08–24). Harvard Law School.
[https://doi.org/10.2139/ssrn.1089230]
-
Kelly, S., Kaye, S.-A., & Oviedo-Trespalacios, O. (2023). What factors contribute to the acceptance of artificial intelligence? A systematic review. Telematics and Informatics, 77, 101925.
[https://doi.org/10.1016/j.tele.2022.101925]
- Kohl, C., Knigge, M., Baader, G., Böhm, M., & Krcmar, H. (2018). Anticipating acceptance of emerging technologies using Twitter: The case of self-driving cars. Journal of Business Economics, 88(5), 617–642.
-
Korzynski, P., Mazurek, G., Altmann, A., Ejdys, J., Kazlauskaite, R., Paliszkiewicz, J., Wach, K., & Ziemba, E. (2023). Generative artificial intelligence as a new context for management theories: Analysis of ChatGPT. Central European Management Journal, 31(1), 3–13.
[https://doi.org/10.1108/CEMJ-02-2023-0091]
-
Kshetri, N., Dwivedi, Y. K., Davenport, T. H., & Panteli, N. (2023). Generative artificial intelligence in marketing: Applications, opportunities, challenges, and research agenda. International Journal of Information Management, 75, 102716.
[https://doi.org/10.1016/j.ijinfomgt.2023.102716]
-
Kusiak, A. (2020). Convolutional and generative adversarial neural networks in manufacturing. International Journal of Production Research, 58(5), 1594–1604.
[https://doi.org/10.1080/00207543.2019.1662133]
- Lee, J. D., & See, K. A. (2004). Trust in automation: Designing for appropriate reliance. Human Factors, 46(1), 50–80.
-
Leiter, C., Zhang, R., Chen, Y., Belouadi, J., Larionov, D., Fresen, V., & Eger, S. (2024). Chatgpt: A meta-analysis after 2.5 months. Machine Learning with Applications, 16, 100541.
[https://doi.org/10.1016/j.mlwa.2024.100541]
-
Li, J., & Huang, J.-S. (2020). Dimensions of artificial intelligence anxiety based on the integrated fear acquisition theory. Technology in Society, 63, 101410.
[https://doi.org/10.1016/j.techsoc.2020.101410]
-
Liang, Y., & Lee, S. A. (2017). Fear of autonomous robots and artificial intelligence: Evidence from national representative data with probability sampling. International Journal of Social Robotics, 9(3), 379–384.
[https://doi.org/10.1007/s12369-017-0401-3]
-
Liu, B. (2021). In AI we trust? Effects of agency locus and transparency on uncertainty reduction in human-AI interaction. Journal of Computer-Mediated Communication, 26(6), 384–402.
[https://doi.org/10.1093/jcmc/zmab013]
- Lukyanenko, R., Maass, W., & Storey, V. C. (2022). Trust in artificial intelligence: From a Foundational Trust Framework to emerging research opportunities. Electronic Markets, 32(4), 1993–2020.
- Lund, B. D., Omame, I., Tijani, S., & Agbaji, D. (2020). Perceptions toward Artificial Intelligence among academic library employees and alignment with the diffusion of innovations’ adopter categories. College & Research Libraries, 81(5), 865–882.
-
Manikonda, L., & Kambhampati, S. (2018). Tweeting AI: Perceptions of lay versus expert Twitterati. ICWSM 2018: Proceedings of the 12th International AAAI Conference on Web and Social Media (pp. 652–655). AAAI.
[https://doi.org/10.1609/icwsm.v12i1.15061]
-
Martinho, A. (2025). Surveying Judges about artificial intelligence: Profession, judicial adjudication, and legal principles. AI & SOCIETY, 40(2), 569–584.
[https://doi.org/10.1007/s00146-024-01869-4]
- McCallum, S. (2023, April 1). ChatGPT banned in Italy over privacy concerns. BBC. https://www.bbc.com/news/technology-65139406
-
Men, L. R., Zhou, A., Jin, J., & Thelen, P. (2023). Shaping corporate character via chatbot social conversation: Impact on organization-public relational outcomes. Public Relations Review, 49(5), 102385.
[https://doi.org/10.1016/j.pubrev.2023.102385]
-
Meskys, E., Liaudanskas, A., Kalpokiene, J., & Jurcys, P. (2020). Regulating deep fakes: Legal and ethical considerations. Journal of Intellectual Property Law & Practice, 15(1), 24–31.
[https://doi.org/10.1093/jiplp/jpz167]
-
Miyazaki, K., Murayama, T., Uchiba, T., An, J., & Kwak, H. (2024). Public perception of generative AI on Twitter: An empirical study based on occupation and usage. DPJ Data Science, 13(1), 2.
[https://doi.org/10.1140/epjds/s13688-023-00445-y]
-
Mohr, J. W., & Bogdanov, P. (2013). Introduction—Topic models: What they are and why they matter. Poetics, 41(6), 545–569.
[https://doi.org/10.1016/j.poetic.2013.10.001]
-
Molina, M. D., & Sundar, S. S. (2022). Does distrust in humans predict greater trust in AI? Role of individual differences in user responses to content moderation. New Media & Society, 26(6), 3638–3656.
[https://doi.org/10.1177/14614448221103534]
-
Nah, F.-H. F., Zheng, R., Cai, J., Siau, K., & Chen, L. (2023). Generative AI and ChatGPT: Applications, challenges, and AI-human collaboration. Journal of Information Technology Case and Application Research, 25(3), 277–304.
[https://doi.org/10.1080/15228053.2023.2233814]
- Nuortimo, K., Härkönen, J., & Karvonen, E. (2018). Exploring the global media image of solar power. Renew Sustain Energy Review, 81, 2806–2811.
-
Obrenovic, B., Gu, X., Wang, G., Godinic, D., & Jakhongirov, I. (2025). Generative AI and human-robot interaction: Implications and future agenda for business, society and ethics. AI & SOCIETY, 40(2), 677–690.
[https://doi.org/10.1007/s00146-024-01889-0]
- Ortiz, S. (2023, November 13). What is ChatGPT and why does it matter? Here’s what you need to know. ZDNET. https://www.zdnet.com/article/what-is-chatgpt-and-why-does-it-matter-heres-everything-you-need-to-know/
-
Ouchchy, L., Coin, A., & Dubljević, V. (2020). AI in the headlines: The portrayal of the ethical issues of Artificial Intelligence in the media. AI & SOCIETY, 35(4), 927–936.
[https://doi.org/10.1007/s00146-020-00965-5]
-
Perperoglou, A., Sauerbrei, W., Abrahamowicz, M., & Schmid, M. (2019). A review of spline function procedures in R. BMC Medical Research Methodology, 19(1), 46.
[https://doi.org/10.1186/s12874-019-0666-3]
- Qi, W., Pan, J., Lyu, H., & Luo, J. (2024). Excitements and concerns in the post-ChatGPT era: Deciphering public perception of AI through social media analysis. Telematics and Informatics, 92, 102158.
-
Roberts, M. E., Stewart, B. M., & Airoldi, E. M. (2016). A model of text for experimentation in the social sciences. Journal of the American Statistical Association, 111(515), 988–1003.
[https://doi.org/10.1080/01621459.2016.1141684]
-
Roberts, M. E., Stewart, B. M., & Tingley, D. (2019). stm: An R package for structural topic models. Journal of Statistical Software, 91(2), 1–40.
[https://doi.org/10.18637/jss.v091.i02]
- Rogers, E. M. (2003). Diffusion of innovations (5th ed.). Free Press.
- Rosenblatt, K. (2023, January 6). ChatGPT banned from New York City public schools’ devices and networks. NBC News. https://www.nbcnews.com/tech/tech-news/new-york-city-public-schools-ban-chatgpt-devices-networks-rcna64446
-
Saadi, J. I., & Yang, M. C. (2023). Generative design: Reframing the role of the designer in early-stage design process. Journal of Mechanical Design, 145(4), 041411.
[https://doi.org/10.1115/1.4056799]
-
Sartori, L., & Bocca, G. (2023). Minding the gap(s): Public perceptions of AI and socio-technical imaginaries. AI & SOCIETY, 38(2), 443–458.
[https://doi.org/10.1007/s00146-022-01422-1]
- Scheufele, D. A. (1999). Framing as a theory of media effects. Journal of Communication, 49(1), 103–122.
- Schiavo, G., Businaro, S., & Zancanaro, M. (2024). Comprehension, apprehension, and acceptance: Understanding the influence of literacy and anxiety on acceptance of artificial Intelligence. Technology in Society, 77, 102537.
- Shim, H., Cho, J., & Sung, Y. H. (2024). Unveiling secrets to AI agents: Exploring the interplay of conversation type, self-disclosure, and privacy insensitivity. Asian Communication Research, 21(2), 195–216.
- Shin, D. (2022). How do people judge the credibility of algorithmic sources? AI & SOCIETY, 37(1), 81–96.
- Shin, J., & Chan-Olmsted, S. (2023). User perceptions and trust of explainable machine learning fake news detectors. International Journal of Communication, 17, 518–540.
-
So, H. J., Jang, H., Kim, M., & Choi, J. (2024). Exploring public perceptions of generative AI and education: Topic modelling of YouTube comments in Korea. Asia Pacific Journal of Education, 44(1), 61–80.
[https://doi.org/10.1080/02188791.2023.2294699]
- Sood, G. (2019). Tuber: Access YouTube from R. R package version 0.9.8. https://github.com/soodoku/tuber
-
Sproat, R., Black, A. W., Chen, S., Kumar, S., Ostendorf, M., & Richards, C. D. (2001). Normalization of non-standard words. Computer Speech & Language, 15(3), 287–333.
[https://doi.org/10.1006/csla.2001.0169]
-
Sturgis, P., & Allum, N. (2004). Science in society: Re-Evaluating the deficit model of public attitudes. Public Understanding of Science, 13(1), 55–74.
[https://doi.org/10.1177/0963662504042690]
-
Taecharungroj, V. (2023). “What can ChatGPT do?” Analyzing early reactions to the innovative AI chatbot on Twitter. Big Data and Cognitive Computing, 7(1), 35.
[https://doi.org/10.3390/bdcc7010035]
-
Teubner, T., Flath, C. M., Weinhardt, C., van der Aalst, W., & Hinz, O. (2023). Welcome to the Era of ChatGPT et al. Business & Information Systems Engineering, 65(2), 95–101.
[https://doi.org/10.1007/s12599-023-00795-x]
-
Vijayarani, S., & Janani, R. (2016). Text mining: Open source tokenization tools - An analysis. Advanced Computational Intelligence: An International Journal, 3(1), 37–47.
[https://doi.org/10.5121/acii.2016.3104]
- Vogel, M. (2023). The ChatGPT list of lists: A collection of 3000+ prompts, GPTs, use-cases, tools, APIs, extensions, fails and other resources. https://medium.com/@maximilian.vogel/the-chatgpt-list-of-lists-a-collection-of-1500-useful-mind-blowing-and-strange-use-cases-8b14c35eb
- Wang, S. (2023). Factors related to user perceptions of artificial intelligence (AI)-based content moderation on social media. Computers in Human Behavior, 149, 107971.
- WHO (World Health Organization). (2018). Big data and artificial intelligence for achieving universal health coverage: An international consultation on ethics. https://www.who.int/ethics/publications/big-data-artificial-intelligence-report/en/
-
Xu, S., Kee, K. F., Li, W., Yamamoto, M., & Riggs, R. E. (2023). Examining the diffusion of Innovations from a dynamic, differential-effects perspective: A longitudinal study on AI adoption among employees. Communication Research, 51(7), 843–866.
[https://doi.org/10.1177/00936502231191832]
-
Zhang, B., & Dafoe, A. (2019). Artificial intelligence: American attitudes and trends. Center for the Governance of AI, Future of Humanity Institute, University of Oxford.
[https://doi.org/10.2139/ssrn.3312874]
-
Zhu, K., Dong, S., Xu, S., & Kraemer, K. L. (2006). Innovation diffusion in global contexts: Determinants of post-adoption digital transformation of European companies. European Journal of Information System, 15(6), 601–616.
[https://doi.org/10.1057/palgrave.ejis.3000650]